Problem: Singular Value Decomposition and Advanced Matrix Concepts Let A ER4×4 be the matrix given by: /1 200 2 0 3 0 A = 0 3 0 1 0 0 1 1 (a) Compute the Singular Value Decomposition (SVD) of matrix A. Provide the following components of the SVD: The left singular matrix U, • The diagonal matrix of singular values Σ, The right singular matrix V. Explain the process of obtaining each component of the SVD, including intermediate steps. (b) Determine the eigenvalues and eigenvectors of ATA and AAT. • Show the relationship between the eigenvalues of ATA and the singular values of A. Verify that the eigenvectors of AT A correspond to the right singular vectors of A, and that the eigenvectors of AAT correspond to the left singular vectors of A. (c) Rank and approximation of A. • • • Calculate the rank of A using the SVD. Explain how the rank of a matrix can be derived from its singular values. Using the SVD, find the best rank-2 approximation A2 of matrix A. Discuss how the approximation is obtained by truncating the smaller singular values. Compute the Frobenius norm of the difference ||A - A2||F and interpret the result. (d) Low-rank matrix compression and energy capture. • Suppose you are using the SVD for data compression. How much energy (i.e., variance) is captured by the best rank-2 approximation of A? • Compute the energy captured by the rank-2 approximation as a percentage of the total energy, where the energy is defined as the sum of squares of the singular values.
Problem: Singular Value Decomposition and Advanced Matrix Concepts Let A ER4×4 be the matrix given by: /1 200 2 0 3 0 A = 0 3 0 1 0 0 1 1 (a) Compute the Singular Value Decomposition (SVD) of matrix A. Provide the following components of the SVD: The left singular matrix U, • The diagonal matrix of singular values Σ, The right singular matrix V. Explain the process of obtaining each component of the SVD, including intermediate steps. (b) Determine the eigenvalues and eigenvectors of ATA and AAT. • Show the relationship between the eigenvalues of ATA and the singular values of A. Verify that the eigenvectors of AT A correspond to the right singular vectors of A, and that the eigenvectors of AAT correspond to the left singular vectors of A. (c) Rank and approximation of A. • • • Calculate the rank of A using the SVD. Explain how the rank of a matrix can be derived from its singular values. Using the SVD, find the best rank-2 approximation A2 of matrix A. Discuss how the approximation is obtained by truncating the smaller singular values. Compute the Frobenius norm of the difference ||A - A2||F and interpret the result. (d) Low-rank matrix compression and energy capture. • Suppose you are using the SVD for data compression. How much energy (i.e., variance) is captured by the best rank-2 approximation of A? • Compute the energy captured by the rank-2 approximation as a percentage of the total energy, where the energy is defined as the sum of squares of the singular values.
Advanced Engineering Mathematics
10th Edition
ISBN:9780470458365
Author:Erwin Kreyszig
Publisher:Erwin Kreyszig
Chapter2: Second-order Linear Odes
Section: Chapter Questions
Problem 1RQ
Related questions
Question
Note for the student:
For the SVD, eigenvalue decompositions, and matrix approximations, you may use appropriate computational tools for complex calculations, but all theoretical steps and justifications must be clearly presented.

Transcribed Image Text:Problem: Singular Value Decomposition and Advanced Matrix Concepts
Let A ER4×4 be the matrix given by:
/1
200
2
0
3 0
A =
0
3 0 1
0
0 1 1
(a) Compute the Singular Value Decomposition (SVD) of matrix A.
Provide the following components of the SVD:
The left singular matrix U,
•
The diagonal matrix of singular values Σ,
The right singular matrix V.
Explain the process of obtaining each component of the SVD, including intermediate steps.
(b) Determine the eigenvalues and eigenvectors of ATA and AAT.
•
Show the relationship between the eigenvalues of ATA and the singular values of A.
Verify that the eigenvectors of AT A correspond to the right singular vectors of A, and that the
eigenvectors of AAT correspond to the left singular vectors of A.

Transcribed Image Text:(c) Rank and approximation of A.
•
•
•
Calculate the rank of A using the SVD. Explain how the rank of a matrix can be derived from its
singular values.
Using the SVD, find the best rank-2 approximation A2 of matrix A. Discuss how the
approximation is obtained by truncating the smaller singular values.
Compute the Frobenius norm of the difference ||A - A2||F and interpret the result.
(d) Low-rank matrix compression and energy capture.
•
Suppose you are using the SVD for data compression. How much energy (i.e., variance) is
captured by the best rank-2 approximation of A?
• Compute the energy captured by the rank-2 approximation as a percentage of the total energy,
where the energy is defined as the sum of squares of the singular values.
Expert Solution

This question has been solved!
Explore an expertly crafted, step-by-step solution for a thorough understanding of key concepts.
Step by step
Solved in 2 steps with 4 images

Recommended textbooks for you
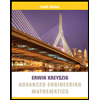
Advanced Engineering Mathematics
Advanced Math
ISBN:
9780470458365
Author:
Erwin Kreyszig
Publisher:
Wiley, John & Sons, Incorporated
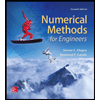
Numerical Methods for Engineers
Advanced Math
ISBN:
9780073397924
Author:
Steven C. Chapra Dr., Raymond P. Canale
Publisher:
McGraw-Hill Education
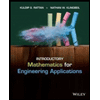
Introductory Mathematics for Engineering Applicat…
Advanced Math
ISBN:
9781118141809
Author:
Nathan Klingbeil
Publisher:
WILEY
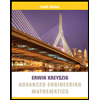
Advanced Engineering Mathematics
Advanced Math
ISBN:
9780470458365
Author:
Erwin Kreyszig
Publisher:
Wiley, John & Sons, Incorporated
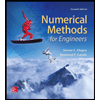
Numerical Methods for Engineers
Advanced Math
ISBN:
9780073397924
Author:
Steven C. Chapra Dr., Raymond P. Canale
Publisher:
McGraw-Hill Education
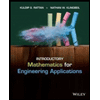
Introductory Mathematics for Engineering Applicat…
Advanced Math
ISBN:
9781118141809
Author:
Nathan Klingbeil
Publisher:
WILEY
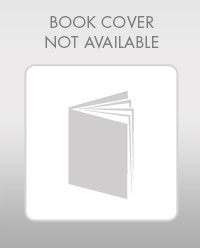
Mathematics For Machine Technology
Advanced Math
ISBN:
9781337798310
Author:
Peterson, John.
Publisher:
Cengage Learning,
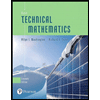
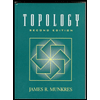