Objective #1: Implement a function in Matlab that finds that parameters, b_hat, of a polynomial regression model. Begin from 'regress_fit_poly.m', which is a stub (i.e., unfinished) version of the function provided for you. The inputs and outputs of your function should conform to the following specifications: % Inputs: % x - A n-by-1 vector of feature values % (where n is number of data points) % y - A n-by-1 vector of response variable values % p - A scalar value, indicating the polynomial order % Outputs: % b_hat - a p+1-by-1 vector of regression coefficients Note: Your function should be able to calculate the polynomial regression parameters for a model of any order (i.e., an input ‘p’ of any value). Note: To see if your function is working correctly, you can check the outputs of your function against those produced by Matlab's 'polyfit' function. However, you should not call ‘polyfit’ inside your own function. Note: Pay special attention to the order of the parameters, which is important, and which may be different than you expect. If you notice that the parameters coming from your function differ from those of 'polyfit', think hard about how you might rearrange them to match. You have several options. For instance, you could change the ordering of columns in the design matrix. Alternatively, you could look into the Matlab functions 'fliplr' and 'flipud', which might help.
Objective #1:
Implement a function in Matlab that finds that parameters, b_hat, of a polynomial regression model.
Begin from 'regress_fit_poly.m', which is a stub (i.e., unfinished) version of the function provided for
you. The inputs and outputs of your function should conform to the following specifications:
% Inputs:
% x - A n-by-1
% (where n is number of data points)
% y - A n-by-1 vector of response variable values
% p - A scalar value, indicating the polynomial order
% Outputs:
% b_hat - a p+1-by-1 vector of regression coefficients
Note: Your function should be able to calculate the polynomial regression parameters for a model of any
order (i.e., an input ‘p’ of any value).
Note: To see if your function is working correctly, you can check the outputs of your function against
those produced by Matlab's 'polyfit' function. However, you should not call ‘polyfit’ inside your own
function.
Note: Pay special attention to the order of the parameters, which is important, and which may be different
than you expect. If you notice that the parameters coming from your function differ from those of 'polyfit',
think hard about how you might rearrange them to match. You have several options. For instance, you
could change the ordering of columns in the design matrix. Alternatively, you could look into the Matlab
functions 'fliplr' and 'flipud', which might help.


Trending now
This is a popular solution!
Step by step
Solved in 4 steps with 2 images

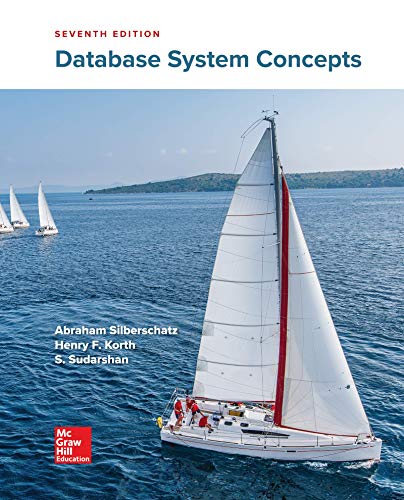
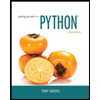
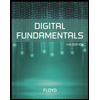
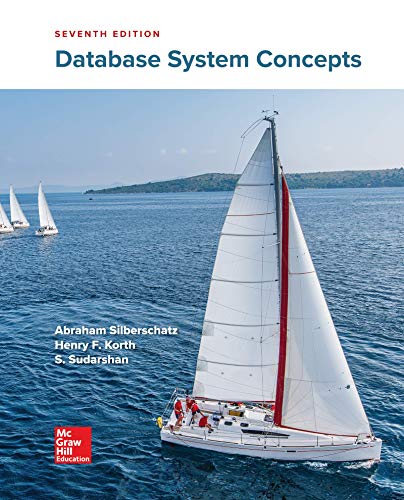
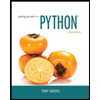
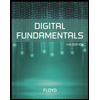
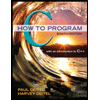
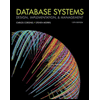
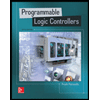