In order to guarantee that management fully understands the process, you have mapped out the following steps to ensure you have captured the process and have data to support your findings and to share with management. Your plan is to demonstrate computations on a simple 3 x 3 matrix where the computations are easier to follow. Then you will perform similar computations on a large image to compress the image data without significantly degrading image quality. To develop your idea proposal, work the problems described below. As you complete each part, make sure to show your work and carefully describe how you arrive at your final answer. Any MATLAB code or MATLAB terminal outputs you generate should be included in your idea proposal to support your answers and work. 1. Consider the matrix: 3 x 3: [1 2 31 A 3 3 4 5 6 7 Use the svd() function in MATLAB to compute A₁, the rank-1 approximation of A. Clearly state what A₁ is, rounded to 4 decimal places. Also, compute the root mean square error (RMSE) between A and A₁. 2. Use the svd() function in MATLAB to compute A2, the rank-2 approximation of A. Clearly state what A2 is, rounded to 4 decimal places. Also, compute the root mean square error (RMSE) between A and A2. Which approximation is better, A₁ or A2? Explain.
In order to guarantee that management fully understands the process, you have mapped out the following steps to ensure you have captured the process and have data to support your findings and to share with management. Your plan is to demonstrate computations on a simple 3 x 3 matrix where the computations are easier to follow. Then you will perform similar computations on a large image to compress the image data without significantly degrading image quality. To develop your idea proposal, work the problems described below. As you complete each part, make sure to show your work and carefully describe how you arrive at your final answer. Any MATLAB code or MATLAB terminal outputs you generate should be included in your idea proposal to support your answers and work. 1. Consider the matrix: 3 x 3: [1 2 31 A 3 3 4 5 6 7 Use the svd() function in MATLAB to compute A₁, the rank-1 approximation of A. Clearly state what A₁ is, rounded to 4 decimal places. Also, compute the root mean square error (RMSE) between A and A₁. 2. Use the svd() function in MATLAB to compute A2, the rank-2 approximation of A. Clearly state what A2 is, rounded to 4 decimal places. Also, compute the root mean square error (RMSE) between A and A2. Which approximation is better, A₁ or A2? Explain.
Advanced Engineering Mathematics
10th Edition
ISBN:9780470458365
Author:Erwin Kreyszig
Publisher:Erwin Kreyszig
Chapter2: Second-order Linear Odes
Section: Chapter Questions
Problem 1RQ
Related questions
Question
100%
Hi there - I am looking for some assitance on how to answer the explanation for problem 2.
If the value of the RSME result is the same for A1 and A2, then does that mean both approximations are equal to one another? Or is approximation still better than the other? I feel like I am missing something.
Thank you!
Problem 1
Use the svd() function in MATLAB to compute , the rank-1 approximation of A. Clearly state what is, rounded to 4 decimal places. Also, compute the root-mean square error (RMSE) between A and .
My Solution:
A = [1 2 2; 3 3 4; 5 6 7]
[u, s, v] = svd(A)
k = 1
A1 = u(:,1:k)*s(1:k,1:k)*v(:,1:k)'
rmse = rms(sqrt(mean((A - A1).^2)))
Problem 2
Use the svd() function in MATLAB to compute , the rank-2 approximation of A. Clearly state what is, rounded to 4 decimal places. Also, compute the root-mean square error (RMSE) between A and . Which approximation is better, or ? Explain.
My Solution:
A = [1 2 2; 3 3 4; 5 6 7]
[u, s, v] = svd(A)
k = 2
A2 = u(:,1:k)*s(1:k,1:k)*v(:,1:k)'
rmse = rms(sqrt(mean((A - A1).^2)))
![---
### Understanding Matrix Approximations Using SVD in MATLAB
In order to guarantee that management fully understands the process, we have mapped out the following steps to ensure you have captured the process and have data to support your findings and to share with management. Your plan is to demonstrate computations on a simple \(3 \times 3\) matrix where the computations are easier to follow. Then you will perform similar computations on a large image to compress the image data without significantly degrading image quality.
To develop your idea proposal, work the problems described below. As you complete each part, make sure to show your work and carefully describe how you arrive at your final answer. Any MATLAB code or MATLAB terminal outputs you generate should be included in your idea proposal to support your answers and work.
1. **Consider the matrix \( 3 \times 3 \):**
\[
A = \begin{bmatrix}
1 & 2 & 3 \\
3 & 3 & 4 \\
5 & 6 & 7
\end{bmatrix}
\]
2. **Using the svd() function in MATLAB to compute \(A_1\), the rank-1 approximation of \( A \).** Clearly state what \( A_1 \) is, rounded to 4 decimal places. Also, compute the root mean square error (RMSE) between \( A \) and \( A_1 \).
3. **Using the svd() function in MATLAB to compute \( A_2 \), the rank-2 approximation of \( A \).** Clearly state what \( A_2 \) is, rounded to 4 decimal places. Also, compute the root mean square error (RMSE) between \( A \) and \( A_2 \). Which approximation is better, \( A_1 \) or \( A_2 \)? Explain.
For each step, ensure you illustrate your calculations, MATLAB code examples, and clearly narrated explanations to facilitate understanding. This process is crucial for compressing image data effectively in larger applications.
---](/v2/_next/image?url=https%3A%2F%2Fcontent.bartleby.com%2Fqna-images%2Fquestion%2Faf8aa82a-31a1-4382-a30e-3ad6a3174f84%2F9035c8f5-5745-4fdb-a31a-0a36ccc75442%2Fpc6g48_processed.png&w=3840&q=75)
Transcribed Image Text:---
### Understanding Matrix Approximations Using SVD in MATLAB
In order to guarantee that management fully understands the process, we have mapped out the following steps to ensure you have captured the process and have data to support your findings and to share with management. Your plan is to demonstrate computations on a simple \(3 \times 3\) matrix where the computations are easier to follow. Then you will perform similar computations on a large image to compress the image data without significantly degrading image quality.
To develop your idea proposal, work the problems described below. As you complete each part, make sure to show your work and carefully describe how you arrive at your final answer. Any MATLAB code or MATLAB terminal outputs you generate should be included in your idea proposal to support your answers and work.
1. **Consider the matrix \( 3 \times 3 \):**
\[
A = \begin{bmatrix}
1 & 2 & 3 \\
3 & 3 & 4 \\
5 & 6 & 7
\end{bmatrix}
\]
2. **Using the svd() function in MATLAB to compute \(A_1\), the rank-1 approximation of \( A \).** Clearly state what \( A_1 \) is, rounded to 4 decimal places. Also, compute the root mean square error (RMSE) between \( A \) and \( A_1 \).
3. **Using the svd() function in MATLAB to compute \( A_2 \), the rank-2 approximation of \( A \).** Clearly state what \( A_2 \) is, rounded to 4 decimal places. Also, compute the root mean square error (RMSE) between \( A \) and \( A_2 \). Which approximation is better, \( A_1 \) or \( A_2 \)? Explain.
For each step, ensure you illustrate your calculations, MATLAB code examples, and clearly narrated explanations to facilitate understanding. This process is crucial for compressing image data effectively in larger applications.
---
![# Matrix Decomposition and Analysis
## v = 3x3
\[ v = \begin{bmatrix}
-0.4775 & -0.7215 & 0.5014 \\
-0.5656 & 0.6891 & 0.4531 \\
-0.6724 & -0.0673 & -0.7371
\end{bmatrix} \]
## Iteration 1 (k = 1)
### Matrix A1 = 3x3
\[ A1 = \begin{bmatrix}
1.4102 & 1.6704 & 1.9860 \\
2.7783 & 3.2099 & 3.9128 \\
5.0075 & 5.9314 & 7.0523
\end{bmatrix} \]
#### Root Mean Square Error
\[ \text{rmse} = 0.2176 \]
## Matrix A = 3x3
\[ A = \begin{bmatrix}
1 & 2 & 2 \\
3 & 3 & 4 \\
5 & 6 & 7
\end{bmatrix} \]
### Matrix u = 3x3
\[ u = \begin{bmatrix}
-0.2391 & 0.8155 & -0.5271 \\
-0.4711 & -0.5721 & -0.6714 \\
-0.8491 & 0.0877 & 0.5209
\end{bmatrix} \]
### Matrix s = 3x3
\[ s = \begin{bmatrix}
12.3521 & 0 & 0 \\
0 & 0.6403 & 0 \\
0 & 0 & 0.1264
\end{bmatrix} \]
### Matrix v = 3x3
\[ v = \begin{bmatrix}
-0.4775 & -0.7215 & 0.5014 \\
-0.5656 & 0.6891 & 0.4531 \\
-0.6724 & -0.0673 & -0.7371
\end{bmatrix} \]
## Iteration 2 (k = 2)
### Matrix A2 = 3x3
\[](/v2/_next/image?url=https%3A%2F%2Fcontent.bartleby.com%2Fqna-images%2Fquestion%2Faf8aa82a-31a1-4382-a30e-3ad6a3174f84%2F9035c8f5-5745-4fdb-a31a-0a36ccc75442%2Fkh3u4h_processed.png&w=3840&q=75)
Transcribed Image Text:# Matrix Decomposition and Analysis
## v = 3x3
\[ v = \begin{bmatrix}
-0.4775 & -0.7215 & 0.5014 \\
-0.5656 & 0.6891 & 0.4531 \\
-0.6724 & -0.0673 & -0.7371
\end{bmatrix} \]
## Iteration 1 (k = 1)
### Matrix A1 = 3x3
\[ A1 = \begin{bmatrix}
1.4102 & 1.6704 & 1.9860 \\
2.7783 & 3.2099 & 3.9128 \\
5.0075 & 5.9314 & 7.0523
\end{bmatrix} \]
#### Root Mean Square Error
\[ \text{rmse} = 0.2176 \]
## Matrix A = 3x3
\[ A = \begin{bmatrix}
1 & 2 & 2 \\
3 & 3 & 4 \\
5 & 6 & 7
\end{bmatrix} \]
### Matrix u = 3x3
\[ u = \begin{bmatrix}
-0.2391 & 0.8155 & -0.5271 \\
-0.4711 & -0.5721 & -0.6714 \\
-0.8491 & 0.0877 & 0.5209
\end{bmatrix} \]
### Matrix s = 3x3
\[ s = \begin{bmatrix}
12.3521 & 0 & 0 \\
0 & 0.6403 & 0 \\
0 & 0 & 0.1264
\end{bmatrix} \]
### Matrix v = 3x3
\[ v = \begin{bmatrix}
-0.4775 & -0.7215 & 0.5014 \\
-0.5656 & 0.6891 & 0.4531 \\
-0.6724 & -0.0673 & -0.7371
\end{bmatrix} \]
## Iteration 2 (k = 2)
### Matrix A2 = 3x3
\[
Expert Solution

This question has been solved!
Explore an expertly crafted, step-by-step solution for a thorough understanding of key concepts.
This is a popular solution!
Trending now
This is a popular solution!
Step by step
Solved in 3 steps with 4 images

Recommended textbooks for you
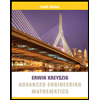
Advanced Engineering Mathematics
Advanced Math
ISBN:
9780470458365
Author:
Erwin Kreyszig
Publisher:
Wiley, John & Sons, Incorporated
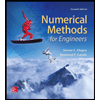
Numerical Methods for Engineers
Advanced Math
ISBN:
9780073397924
Author:
Steven C. Chapra Dr., Raymond P. Canale
Publisher:
McGraw-Hill Education
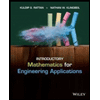
Introductory Mathematics for Engineering Applicat…
Advanced Math
ISBN:
9781118141809
Author:
Nathan Klingbeil
Publisher:
WILEY
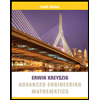
Advanced Engineering Mathematics
Advanced Math
ISBN:
9780470458365
Author:
Erwin Kreyszig
Publisher:
Wiley, John & Sons, Incorporated
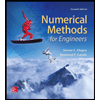
Numerical Methods for Engineers
Advanced Math
ISBN:
9780073397924
Author:
Steven C. Chapra Dr., Raymond P. Canale
Publisher:
McGraw-Hill Education
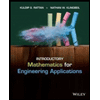
Introductory Mathematics for Engineering Applicat…
Advanced Math
ISBN:
9781118141809
Author:
Nathan Klingbeil
Publisher:
WILEY
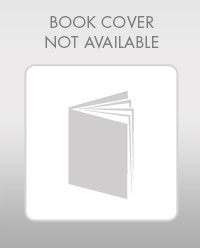
Mathematics For Machine Technology
Advanced Math
ISBN:
9781337798310
Author:
Peterson, John.
Publisher:
Cengage Learning,
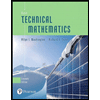
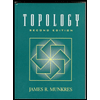