In Lecture 2, we found that h* error: = Mean (y1, 2, ..., Yn) is the constant prediction that minimizes mean squared n 1 Rsq (h) (Yi - h)² n i=1 To arrive at this result, we used calculus: we took the derivative of Rsq (h) with respect to h, set it equal to 0, and solved for the resulting value of h, which we called h*. 3 In this problem, we will minimize Rsq (h) in a way that doesn't use calculus. The general idea is this: if f(x) = (x−c)²+k, then we know that f is a quadratic function that opens upwards with a vertex at (c, k), meaning that x = c minimizes f. As we saw in class (see Lecture 2, slide 16), Rsq (h) is a quadratic function of h! Throughout this problem, let y1, 2, ..., yn be an arbitrary dataset, and let ÿ = ½-½ Σ²²±₁ y; be the mean of the y's. 1 n i=1 a) What is the value of 21 (yi - ÿ)? Justify your answer.
In Lecture 2, we found that h* error: = Mean (y1, 2, ..., Yn) is the constant prediction that minimizes mean squared n 1 Rsq (h) (Yi - h)² n i=1 To arrive at this result, we used calculus: we took the derivative of Rsq (h) with respect to h, set it equal to 0, and solved for the resulting value of h, which we called h*. 3 In this problem, we will minimize Rsq (h) in a way that doesn't use calculus. The general idea is this: if f(x) = (x−c)²+k, then we know that f is a quadratic function that opens upwards with a vertex at (c, k), meaning that x = c minimizes f. As we saw in class (see Lecture 2, slide 16), Rsq (h) is a quadratic function of h! Throughout this problem, let y1, 2, ..., yn be an arbitrary dataset, and let ÿ = ½-½ Σ²²±₁ y; be the mean of the y's. 1 n i=1 a) What is the value of 21 (yi - ÿ)? Justify your answer.
Calculus: Early Transcendentals
8th Edition
ISBN:9781285741550
Author:James Stewart
Publisher:James Stewart
Chapter1: Functions And Models
Section: Chapter Questions
Problem 1RCC: (a) What is a function? What are its domain and range? (b) What is the graph of a function? (c) How...
Related questions
Question

Transcribed Image Text:In Lecture 2, we found that h*
error:
=
Mean (y1, 2, ..., Yn) is the constant prediction that minimizes mean squared
n
1
Rsq (h)
(Yi - h)²
n
i=1
To arrive at this result, we used calculus: we took the derivative of Rsq (h) with respect to h, set it equal to
0, and solved for the resulting value of h, which we called h*.
3
In this problem, we will minimize Rsq (h) in a way that doesn't use calculus. The general idea is this: if
f(x) = (x−c)²+k, then we know that f is a quadratic function that opens upwards with a vertex at (c, k),
meaning that x = c minimizes f. As we saw in class (see Lecture 2, slide 16), Rsq (h) is a quadratic function
of h!
Throughout this problem, let y1, 2, ..., yn be an arbitrary dataset, and let ÿ = ½-½ Σ²²±₁ y; be the mean of the
y's.
1
n
i=1
a)
What is the value of 21 (yi - ÿ)? Justify your answer.
Expert Solution

This question has been solved!
Explore an expertly crafted, step-by-step solution for a thorough understanding of key concepts.
Step by step
Solved in 2 steps

Recommended textbooks for you
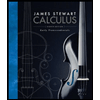
Calculus: Early Transcendentals
Calculus
ISBN:
9781285741550
Author:
James Stewart
Publisher:
Cengage Learning
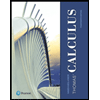
Thomas' Calculus (14th Edition)
Calculus
ISBN:
9780134438986
Author:
Joel R. Hass, Christopher E. Heil, Maurice D. Weir
Publisher:
PEARSON
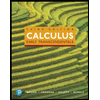
Calculus: Early Transcendentals (3rd Edition)
Calculus
ISBN:
9780134763644
Author:
William L. Briggs, Lyle Cochran, Bernard Gillett, Eric Schulz
Publisher:
PEARSON
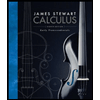
Calculus: Early Transcendentals
Calculus
ISBN:
9781285741550
Author:
James Stewart
Publisher:
Cengage Learning
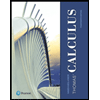
Thomas' Calculus (14th Edition)
Calculus
ISBN:
9780134438986
Author:
Joel R. Hass, Christopher E. Heil, Maurice D. Weir
Publisher:
PEARSON
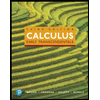
Calculus: Early Transcendentals (3rd Edition)
Calculus
ISBN:
9780134763644
Author:
William L. Briggs, Lyle Cochran, Bernard Gillett, Eric Schulz
Publisher:
PEARSON
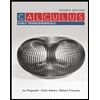
Calculus: Early Transcendentals
Calculus
ISBN:
9781319050740
Author:
Jon Rogawski, Colin Adams, Robert Franzosa
Publisher:
W. H. Freeman
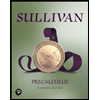
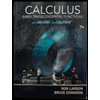
Calculus: Early Transcendental Functions
Calculus
ISBN:
9781337552516
Author:
Ron Larson, Bruce H. Edwards
Publisher:
Cengage Learning