In a regression problem with 1 output variable and p input variables, we carry out a a principal components regression with p principal components constructed from the input variables. This will result in a significant level of dimensionality reduction. PCR is one of the leading methods for dimension reduction. no dimensionality reduction whatsoever. a potentially good level of dimensionality reduction depending on the quality of the principal components us making a bad choice if we care about the output variable. In that case we should have used partial least squares as a better high dimensionality reduction technique.
In a regression problem with 1 output variable and p input variables, we carry out a a principal components regression with p principal components constructed from the input variables. This will result in a significant level of dimensionality reduction. PCR is one of the leading methods for dimension reduction. no dimensionality reduction whatsoever. a potentially good level of dimensionality reduction depending on the quality of the principal components us making a bad choice if we care about the output variable. In that case we should have used partial least squares as a better high dimensionality reduction technique.
Algebra and Trigonometry (MindTap Course List)
4th Edition
ISBN:9781305071742
Author:James Stewart, Lothar Redlin, Saleem Watson
Publisher:James Stewart, Lothar Redlin, Saleem Watson
Chapter1: Equations And Graphs
Section1.FOM: Focus On Modeling: Fitting Lines To Data
Problem 10P
Related questions
Question
-
In a regression problem with 1 output variable and p input variables, we carry out a a principal components regression with p principal components constructed from the input variables. This will result in
a significant level of dimensionality reduction. PCR is one of the leading methods for dimension reduction.
no dimensionality reduction whatsoever.
a potentially good level of dimensionality reduction depending on the quality of the principal components
us making a bad choice if we care about the output variable. In that case we should have used partial least squares as a better high dimensionality reduction technique.
Expert Solution

This question has been solved!
Explore an expertly crafted, step-by-step solution for a thorough understanding of key concepts.
Step by step
Solved in 2 steps

Recommended textbooks for you
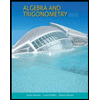
Algebra and Trigonometry (MindTap Course List)
Algebra
ISBN:
9781305071742
Author:
James Stewart, Lothar Redlin, Saleem Watson
Publisher:
Cengage Learning
Algebra & Trigonometry with Analytic Geometry
Algebra
ISBN:
9781133382119
Author:
Swokowski
Publisher:
Cengage
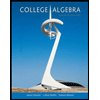
College Algebra
Algebra
ISBN:
9781305115545
Author:
James Stewart, Lothar Redlin, Saleem Watson
Publisher:
Cengage Learning
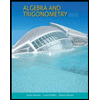
Algebra and Trigonometry (MindTap Course List)
Algebra
ISBN:
9781305071742
Author:
James Stewart, Lothar Redlin, Saleem Watson
Publisher:
Cengage Learning
Algebra & Trigonometry with Analytic Geometry
Algebra
ISBN:
9781133382119
Author:
Swokowski
Publisher:
Cengage
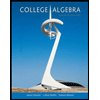
College Algebra
Algebra
ISBN:
9781305115545
Author:
James Stewart, Lothar Redlin, Saleem Watson
Publisher:
Cengage Learning
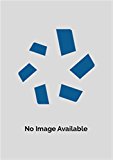
Big Ideas Math A Bridge To Success Algebra 1: Stu…
Algebra
ISBN:
9781680331141
Author:
HOUGHTON MIFFLIN HARCOURT
Publisher:
Houghton Mifflin Harcourt