Imagine we are interested in the mean and variance of variable Y = g(X) with fixed function g(X), however we only know X, and we can obtain the mean of X and the variance of X. In the lectures learned we can ONLY find E(Y) = μy and Var(Y) = o from E(X) = µx and Var(X) = o if g(x) is linear, i.e g(X) = ax + b. However, if we don't know if g(x) is linear we can often solve this by linearization using a Taylor-expansion of g about #x. This
Imagine we are interested in the mean and variance of variable Y = g(X) with fixed function g(X), however we only know X, and we can obtain the mean of X and the variance of X. In the lectures learned we can ONLY find E(Y) = μy and Var(Y) = o from E(X) = µx and Var(X) = o if g(x) is linear, i.e g(X) = ax + b. However, if we don't know if g(x) is linear we can often solve this by linearization using a Taylor-expansion of g about #x. This
A First Course in Probability (10th Edition)
10th Edition
ISBN:9780134753119
Author:Sheldon Ross
Publisher:Sheldon Ross
Chapter1: Combinatorial Analysis
Section: Chapter Questions
Problem 1.1P: a. How many different 7-place license plates are possible if the first 2 places are for letters and...
Related questions
Question
I need this question completed in 10 minutes with handwritten working

Transcribed Image Text:the expected value of Y:
E(Y) ≈ 9(µ₂) + ¹⁄E((X − µ₂)²)g" (µz)
where we can use the definition E((X − µ)²) = o to rewrite this to
E(Y) ≈ 9(µ₂) + 1⁄o¾9″(µ₂)
Lets consider random variable X from a uniform distribution U(0, 1) and a
non-linear transformation g(x) = √√x.
1.Find E(X) = µx and Var(X) = 0².
2.Find the first and second derivatives of g(x) (g'(x)
g"(x) g(x))
=
3. Use the μ you found in part 1 to evaluate the g(x) and g"(x) at x = μx.
This gives you g(x) and g"(µx).
g(x) and
4. We now calculated all the parts we need to find E(y) by putting the
together in the following equation:
1
E(Y) ≈ g(µ«) +-og" (µx)
Find the variance of Y.
Find E(Y).
5. For the Var(Y) we will only use the first order linearization given by
of ≈ 0² (9¹(μ₂)) ²

Transcribed Image Text:Imagine we are interested in the mean and variance of variable Y = g(X)
with fixed function g(X), however we only know X, and we can obtain the
mean of X and the variance of X. In the lectures learned we can ONLY find
E(Y) = µy and Var(Y) = o} from E(X) = µx and Var(X) = o if g(x) is
linear, i.e g(X) =aX+b. However, if we don't know if g(x) is linear we can
often solve this by linearization using a Taylor-expansion of g about ux. This
method is known as the delta method or error of propagation ³. We will
use the Taylor-expansion up to the second order:
1
Y = g(X) ≈ g(µs) + (X − µx)g′(µx) + ½(X − µs)²g″ (µx)
where (X) and (X-μ)2 are the first and second order polynomial
terms, g'(x) and g"(r) are the first and second order derivatives of g(x)
evaluated at x = μlx.
In the next step we take the expectation, and since we know that E(X− μx) = =
0 our second term drops out. We are left with the following approximation of
Expert Solution

This question has been solved!
Explore an expertly crafted, step-by-step solution for a thorough understanding of key concepts.
This is a popular solution!
Trending now
This is a popular solution!
Step by step
Solved in 7 steps

Recommended textbooks for you
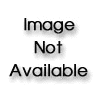
A First Course in Probability (10th Edition)
Probability
ISBN:
9780134753119
Author:
Sheldon Ross
Publisher:
PEARSON
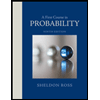
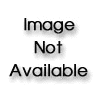
A First Course in Probability (10th Edition)
Probability
ISBN:
9780134753119
Author:
Sheldon Ross
Publisher:
PEARSON
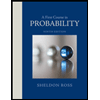