Geometric Perspective of Simple Linear Regression 2. In Lecture 12, we viewed both the simple linear regression model and the multiple linear regression model through the lens of linear algebra. The key geometric insight was that if we train a model on some design matrix X and true response vector Y, our predicted response Y^ = X0^is the vector in span(X) that is closest to Y. In the simple linear regression case, our optimal vector is Ô = [0, 1], and our design matrix is X2 *-1-4 X = = This means we can write our predicted response vector as Ŷ = X [6] = 601+0₂.7. In this problem, 1, is the n-vector of all 1s and refers to the n-length vector [x1, X2, ..., Xn]¹. Note, is a feature, not an observation. For this problem, assume we are working with the simple linear regression model, though the properties we establish here hold for any linear regression model that contains an intercept term. (a) Recall in the last assignment, we showed that Σ΄ n n ei = 0 algebraically. In i=1 n this question, explain why Σe; = 0 using a geometric property. (Hint: e = Y – Ŷ, i=1 and e = = [e₁, €2,..., en] T.) (b) Similarly, show that €₁x₁ = :0 using a geometric property. (Hint: Your Σ i=1 answer should be very similar to the above) (c) Briefly explain why the vector Y^ must also be orthogonal to the residual vector e.
Geometric Perspective of Simple Linear Regression 2. In Lecture 12, we viewed both the simple linear regression model and the multiple linear regression model through the lens of linear algebra. The key geometric insight was that if we train a model on some design matrix X and true response vector Y, our predicted response Y^ = X0^is the vector in span(X) that is closest to Y. In the simple linear regression case, our optimal vector is Ô = [0, 1], and our design matrix is X2 *-1-4 X = = This means we can write our predicted response vector as Ŷ = X [6] = 601+0₂.7. In this problem, 1, is the n-vector of all 1s and refers to the n-length vector [x1, X2, ..., Xn]¹. Note, is a feature, not an observation. For this problem, assume we are working with the simple linear regression model, though the properties we establish here hold for any linear regression model that contains an intercept term. (a) Recall in the last assignment, we showed that Σ΄ n n ei = 0 algebraically. In i=1 n this question, explain why Σe; = 0 using a geometric property. (Hint: e = Y – Ŷ, i=1 and e = = [e₁, €2,..., en] T.) (b) Similarly, show that €₁x₁ = :0 using a geometric property. (Hint: Your Σ i=1 answer should be very similar to the above) (c) Briefly explain why the vector Y^ must also be orthogonal to the residual vector e.
Advanced Engineering Mathematics
10th Edition
ISBN:9780470458365
Author:Erwin Kreyszig
Publisher:Erwin Kreyszig
Chapter2: Second-order Linear Odes
Section: Chapter Questions
Problem 1RQ
Related questions
Question
Please answer question (c)
![Geometric Perspective of Simple Linear Regression
2. In Lecture 12, we viewed both the simple linear regression model and the multiple
linear regression model through the lens of linear algebra. The key geometric insight
was that if we train a model on some design matrix X and true response vector Y, our
predicted response Y^ = XO^is the vector in span(X) that is closest to Y.
In the simple linear regression case, our optimal vector is Ô = [0, 1], and our design
matrix is
X2
--11-
X =
This means we can write our predicted response vector as Ŷ = x [6] = 601, + Ôrē.
X
In this problem, 1, is the n-vector of all 1s and refers to the n-length vector [x₁, x2, ..., Xn]¹.
Note, is a feature, not an observation.
= 1n x
For this problem, assume we are working with the simple linear regression model,
though the properties we establish here hold for any linear regression model that contains
an intercept term.
this question, explain why
and e = [e₁, €2,...,en]T.)
(a) Recall in the last assignment, we showed that ei = 0 algebraically. In
Σ
n
n
i=1
e; = 0 using a geometric property. (Hint: e = Y - Y,
i=1
n
(b) Similarly, show that
Σ
eixi 0 using a geometric property. (Hint: Your
liti
i=1
answer should be very similar to the above)
(c) Briefly explain why the vector Y^ must also be orthogonal to the residual
vector e.](/v2/_next/image?url=https%3A%2F%2Fcontent.bartleby.com%2Fqna-images%2Fquestion%2Fff93c792-abd7-4eff-ba98-8a1e3cbd1351%2Feeecbffe-a1cc-4fa6-9dd7-8a6068ba1aa9%2F38jgxt_processed.jpeg&w=3840&q=75)
Transcribed Image Text:Geometric Perspective of Simple Linear Regression
2. In Lecture 12, we viewed both the simple linear regression model and the multiple
linear regression model through the lens of linear algebra. The key geometric insight
was that if we train a model on some design matrix X and true response vector Y, our
predicted response Y^ = XO^is the vector in span(X) that is closest to Y.
In the simple linear regression case, our optimal vector is Ô = [0, 1], and our design
matrix is
X2
--11-
X =
This means we can write our predicted response vector as Ŷ = x [6] = 601, + Ôrē.
X
In this problem, 1, is the n-vector of all 1s and refers to the n-length vector [x₁, x2, ..., Xn]¹.
Note, is a feature, not an observation.
= 1n x
For this problem, assume we are working with the simple linear regression model,
though the properties we establish here hold for any linear regression model that contains
an intercept term.
this question, explain why
and e = [e₁, €2,...,en]T.)
(a) Recall in the last assignment, we showed that ei = 0 algebraically. In
Σ
n
n
i=1
e; = 0 using a geometric property. (Hint: e = Y - Y,
i=1
n
(b) Similarly, show that
Σ
eixi 0 using a geometric property. (Hint: Your
liti
i=1
answer should be very similar to the above)
(c) Briefly explain why the vector Y^ must also be orthogonal to the residual
vector e.
Expert Solution

This question has been solved!
Explore an expertly crafted, step-by-step solution for a thorough understanding of key concepts.
This is a popular solution!
Trending now
This is a popular solution!
Step by step
Solved in 3 steps with 3 images

Recommended textbooks for you
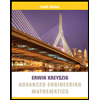
Advanced Engineering Mathematics
Advanced Math
ISBN:
9780470458365
Author:
Erwin Kreyszig
Publisher:
Wiley, John & Sons, Incorporated
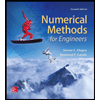
Numerical Methods for Engineers
Advanced Math
ISBN:
9780073397924
Author:
Steven C. Chapra Dr., Raymond P. Canale
Publisher:
McGraw-Hill Education
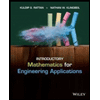
Introductory Mathematics for Engineering Applicat…
Advanced Math
ISBN:
9781118141809
Author:
Nathan Klingbeil
Publisher:
WILEY
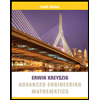
Advanced Engineering Mathematics
Advanced Math
ISBN:
9780470458365
Author:
Erwin Kreyszig
Publisher:
Wiley, John & Sons, Incorporated
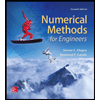
Numerical Methods for Engineers
Advanced Math
ISBN:
9780073397924
Author:
Steven C. Chapra Dr., Raymond P. Canale
Publisher:
McGraw-Hill Education
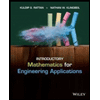
Introductory Mathematics for Engineering Applicat…
Advanced Math
ISBN:
9781118141809
Author:
Nathan Klingbeil
Publisher:
WILEY
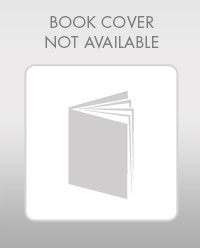
Mathematics For Machine Technology
Advanced Math
ISBN:
9781337798310
Author:
Peterson, John.
Publisher:
Cengage Learning,
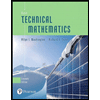
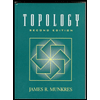