Furthermore, we can use the dot product definition < x, y >= |x||₂||y|l₂ cos(0) to further determine the angle between two vectors. For example, see the below image for computing and formula for computing the angle between two vectors using the dot product definition Machine learning often uses this idea of cosine similarity and the angle between vectors to compute the similarity between different data samples using their feature vectors (i.e., the columns of a dataset)! See examples here! Given the following two vectors find the angle between them (in degrees). Use the above equation, in the picture, but use the L2 norm or ||- ||₂ when computing x and y. Recall to compute the L2-norm for a vector x, we do the following: ||x|| 2 a+²+...+ 2= O 180 0-1 01 0 90 e arccos(x+y/1x1131) = y = -2
Furthermore, we can use the dot product definition < x, y >= |x||₂||y|l₂ cos(0) to further determine the angle between two vectors. For example, see the below image for computing and formula for computing the angle between two vectors using the dot product definition Machine learning often uses this idea of cosine similarity and the angle between vectors to compute the similarity between different data samples using their feature vectors (i.e., the columns of a dataset)! See examples here! Given the following two vectors find the angle between them (in degrees). Use the above equation, in the picture, but use the L2 norm or ||- ||₂ when computing x and y. Recall to compute the L2-norm for a vector x, we do the following: ||x|| 2 a+²+...+ 2= O 180 0-1 01 0 90 e arccos(x+y/1x1131) = y = -2
Advanced Engineering Mathematics
10th Edition
ISBN:9780470458365
Author:Erwin Kreyszig
Publisher:Erwin Kreyszig
Chapter2: Second-order Linear Odes
Section: Chapter Questions
Problem 1RQ
Related questions
Question
![Furthermore, we can use the dot product definition \( \langle x, y \rangle = \| x \|_2 \| y \|_2 \cos(\theta) \) to further determine the angle between two vectors. For example, see the below image for computing and formula for computing the angle between two vectors using the dot product definition.
### Diagram Explanation
The diagram shows two vectors, \( x \) and \( y \), with an angle \( \theta \) between them. The formula depicted is:
\[ \theta = \arccos \left( \frac{x \cdot y}{|x| |y|} \right) \]
Machine learning often uses this idea of cosine similarity and the angle between vectors to compute the similarity between different data samples using their feature vectors (i.e., the columns of a dataset!) See examples [here](#).
#### Problem
Given the following two vectors, find the **angle** between them (in degrees). Use the above equation, in the picture, but **use the L2 norm** or \(\|\cdot \|_2\) when computing \(|x|\) and \(|y|\). Recall to compute the L2-norm for a vector x, we do the following:
\[ \| x \|_2 = \sqrt{x_1^2 + x_2^2 + \cdots + x_n^2} \]
Vectors:
\[ x = \begin{bmatrix} 1 \\ 1 \end{bmatrix} \quad y = \begin{bmatrix} -2 \\ -2 \end{bmatrix} \]
#### Options
- 180
- -1
- 1
- 90](/v2/_next/image?url=https%3A%2F%2Fcontent.bartleby.com%2Fqna-images%2Fquestion%2F2e96339b-d3d9-4039-844d-3fc091742cc5%2F3ac2cd44-9f5e-4494-8018-db76938d3955%2Fd2tqc9q_processed.png&w=3840&q=75)
Transcribed Image Text:Furthermore, we can use the dot product definition \( \langle x, y \rangle = \| x \|_2 \| y \|_2 \cos(\theta) \) to further determine the angle between two vectors. For example, see the below image for computing and formula for computing the angle between two vectors using the dot product definition.
### Diagram Explanation
The diagram shows two vectors, \( x \) and \( y \), with an angle \( \theta \) between them. The formula depicted is:
\[ \theta = \arccos \left( \frac{x \cdot y}{|x| |y|} \right) \]
Machine learning often uses this idea of cosine similarity and the angle between vectors to compute the similarity between different data samples using their feature vectors (i.e., the columns of a dataset!) See examples [here](#).
#### Problem
Given the following two vectors, find the **angle** between them (in degrees). Use the above equation, in the picture, but **use the L2 norm** or \(\|\cdot \|_2\) when computing \(|x|\) and \(|y|\). Recall to compute the L2-norm for a vector x, we do the following:
\[ \| x \|_2 = \sqrt{x_1^2 + x_2^2 + \cdots + x_n^2} \]
Vectors:
\[ x = \begin{bmatrix} 1 \\ 1 \end{bmatrix} \quad y = \begin{bmatrix} -2 \\ -2 \end{bmatrix} \]
#### Options
- 180
- -1
- 1
- 90
Expert Solution

This question has been solved!
Explore an expertly crafted, step-by-step solution for a thorough understanding of key concepts.
Step by step
Solved in 2 steps with 1 images

Recommended textbooks for you
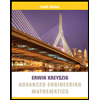
Advanced Engineering Mathematics
Advanced Math
ISBN:
9780470458365
Author:
Erwin Kreyszig
Publisher:
Wiley, John & Sons, Incorporated
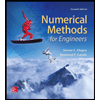
Numerical Methods for Engineers
Advanced Math
ISBN:
9780073397924
Author:
Steven C. Chapra Dr., Raymond P. Canale
Publisher:
McGraw-Hill Education
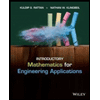
Introductory Mathematics for Engineering Applicat…
Advanced Math
ISBN:
9781118141809
Author:
Nathan Klingbeil
Publisher:
WILEY
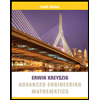
Advanced Engineering Mathematics
Advanced Math
ISBN:
9780470458365
Author:
Erwin Kreyszig
Publisher:
Wiley, John & Sons, Incorporated
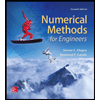
Numerical Methods for Engineers
Advanced Math
ISBN:
9780073397924
Author:
Steven C. Chapra Dr., Raymond P. Canale
Publisher:
McGraw-Hill Education
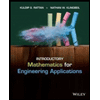
Introductory Mathematics for Engineering Applicat…
Advanced Math
ISBN:
9781118141809
Author:
Nathan Klingbeil
Publisher:
WILEY
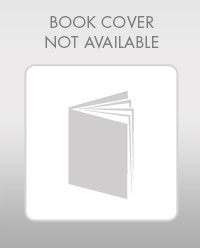
Mathematics For Machine Technology
Advanced Math
ISBN:
9781337798310
Author:
Peterson, John.
Publisher:
Cengage Learning,
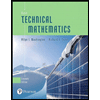
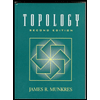