Find the regression equation, letting overhead width be the predictor (x) variable. Find the best predicted weight of a seal if the overhead width measured from a photograph is 1.5 cm. Can the prediction be correct? What is wrong with predicting the weight in this case? Use a significance level of 0.05. 7.3 121 Overhead Width (cm) 7.1 Weight (kg) 139 Click the icon to view the critical values of the Pearson correlation coefficient r. 7.4 154 8.9 209 The regression equation is y=+x. (Round to one decimal place as needed.) 9.9 223 8.5 188 The best predicted weight for an overhead width of 1.5 cm is kg. (Round to one decimal place as needed.) Can the prediction be correct? What is wrong with predicting the weight in this case? OA. The prediction cannot be correct because a negative weight does not make sense. The width in this case is beyond the scope of the available sample data. OB. The prediction cannot be correct because there is not sufficient evidence of a linear correlation. The width in this case is beyond the scope of the available sample data. OC. The prediction cannot be correct because a negative weight does not make sense and because there is not sufficient evidence of a linear correlation. OD. The prediction can be correct. There is nothing wrong with predicting the weight in this case.
Find the regression equation, letting overhead width be the predictor (x) variable. Find the best predicted weight of a seal if the overhead width measured from a photograph is 1.5 cm. Can the prediction be correct? What is wrong with predicting the weight in this case? Use a significance level of 0.05. 7.3 121 Overhead Width (cm) 7.1 Weight (kg) 139 Click the icon to view the critical values of the Pearson correlation coefficient r. 7.4 154 8.9 209 The regression equation is y=+x. (Round to one decimal place as needed.) 9.9 223 8.5 188 The best predicted weight for an overhead width of 1.5 cm is kg. (Round to one decimal place as needed.) Can the prediction be correct? What is wrong with predicting the weight in this case? OA. The prediction cannot be correct because a negative weight does not make sense. The width in this case is beyond the scope of the available sample data. OB. The prediction cannot be correct because there is not sufficient evidence of a linear correlation. The width in this case is beyond the scope of the available sample data. OC. The prediction cannot be correct because a negative weight does not make sense and because there is not sufficient evidence of a linear correlation. OD. The prediction can be correct. There is nothing wrong with predicting the weight in this case.
MATLAB: An Introduction with Applications
6th Edition
ISBN:9781119256830
Author:Amos Gilat
Publisher:Amos Gilat
Chapter1: Starting With Matlab
Section: Chapter Questions
Problem 1P
Related questions
Question
2

Transcribed Image Text:### Regression Analysis and Prediction
#### Problem Statement
Find the regression equation, letting overhead width be the predictor (x) variable. Find the best predicted weight of a seal if the overhead width measured from a photograph is 1.5 cm. Can the prediction be correct? What is wrong with predicting the weight in this case? Use a significance level of 0.05.
#### Data Provided
To find the regression equation, use the following data:
| Overhead Width (cm) | Weight (kg) |
|---------------------|-------------|
| 7.3 | 121 |
| 7.4 | 154 |
| 8.9 | 209 |
| 9.9 | 223 |
| 8.5 | 188 |
| 7.1 | 139 |
Click the icon to view the critical values of the Pearson correlation coefficient r.
#### Steps to Solve
1. **Find the Regression Equation**:
The typical form of a regression equation is \(\hat{y} = a + bx\).
- Here, \(\hat{y}\) is the predicted weight.
- \(x\) is the overhead width.
- \(a\) and \(b\) are constants derived from the data.
(The actual calculation to find a and b is not shown here, but needs to be carried out to derive the equation.)
2. **Compute the Predicted Weight**:
- Insert the overhead width of 1.5 cm into the regression equation to get the predicted weight.
#### Filling the Blanks
- **The regression equation is ** \(\hat{y} = \_ + \_x\) (Round to one decimal place as needed.)
- **The best predicted weight for an overhead width of 1.5 cm is ** \(\_\_\_\) kg. (Round to one decimal place as needed.)
#### Analysis
**Can the prediction be correct?**
- **What is wrong with predicting the weight in this case?**
**Answer options:**
- A. The prediction cannot be correct because a negative weight does not make sense. The width in this case is beyond the scope of the available sample data.
- B. The prediction cannot be correct because there is not sufficient evidence of a linear correlation. The width in this case is beyond the scope of the available sample data.
- C

Transcribed Image Text:### Critical Values of the Pearson Correlation Coefficient
This table provides the critical values of the Pearson correlation coefficient \( r \) at significance levels \( \alpha = 0.05 \) and \( \alpha = 0.01 \).
| \( n \) | \( \alpha = 0.05 \) | \( \alpha = 0.01 \) |
|--------|-------------------|-------------------|
| 4 | 0.950 | 0.990 |
| 5 | 0.878 | 0.959 |
| 6 | 0.811 | 0.917 |
| 7 | 0.754 | 0.875 |
| 8 | 0.707 | 0.834 |
| 9 | 0.666 | 0.798 |
| 10 | 0.632 | 0.765 |
| 11 | 0.602 | 0.735 |
| 12 | 0.576 | 0.708 |
| 13 | 0.553 | 0.684 |
| 14 | 0.532 | 0.661 |
| 15 | 0.514 | 0.641 |
| 16 | 0.497 | 0.623 |
| 17 | 0.482 | 0.606 |
| 18 | 0.468 | 0.590 |
| 19 | 0.456 | 0.575 |
| 20 | 0.444 | 0.561 |
| 25 | 0.396 | 0.505 |
| 30 | 0.361 | 0.463 |
| 35 | 0.334 | 0.430 |
| 40 | 0.312 | 0.402 |
| 45 | 0.294 | 0.378 |
| 50 | 0.279 | 0.361 |
| 60 | 0.254 | 0.330 |
| 70 |
Expert Solution

This question has been solved!
Explore an expertly crafted, step-by-step solution for a thorough understanding of key concepts.
This is a popular solution!
Trending now
This is a popular solution!
Step by step
Solved in 4 steps with 3 images

Recommended textbooks for you
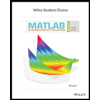
MATLAB: An Introduction with Applications
Statistics
ISBN:
9781119256830
Author:
Amos Gilat
Publisher:
John Wiley & Sons Inc
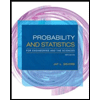
Probability and Statistics for Engineering and th…
Statistics
ISBN:
9781305251809
Author:
Jay L. Devore
Publisher:
Cengage Learning
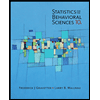
Statistics for The Behavioral Sciences (MindTap C…
Statistics
ISBN:
9781305504912
Author:
Frederick J Gravetter, Larry B. Wallnau
Publisher:
Cengage Learning
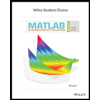
MATLAB: An Introduction with Applications
Statistics
ISBN:
9781119256830
Author:
Amos Gilat
Publisher:
John Wiley & Sons Inc
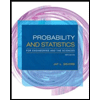
Probability and Statistics for Engineering and th…
Statistics
ISBN:
9781305251809
Author:
Jay L. Devore
Publisher:
Cengage Learning
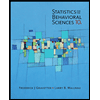
Statistics for The Behavioral Sciences (MindTap C…
Statistics
ISBN:
9781305504912
Author:
Frederick J Gravetter, Larry B. Wallnau
Publisher:
Cengage Learning
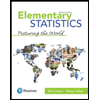
Elementary Statistics: Picturing the World (7th E…
Statistics
ISBN:
9780134683416
Author:
Ron Larson, Betsy Farber
Publisher:
PEARSON
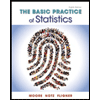
The Basic Practice of Statistics
Statistics
ISBN:
9781319042578
Author:
David S. Moore, William I. Notz, Michael A. Fligner
Publisher:
W. H. Freeman
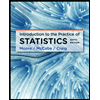
Introduction to the Practice of Statistics
Statistics
ISBN:
9781319013387
Author:
David S. Moore, George P. McCabe, Bruce A. Craig
Publisher:
W. H. Freeman