Document 2: Contains only "trucks". Document 3: Contains both "cars" and "trucks". Document 4: Contains neither "cars" nor "trucks". To evaluate Search A, clearly the search "(cars OR trucks)" will hit on Documents 1, 2, ar earch (which is Search A) will hit everything else, which is Document 4. Evaluating Search B, the search "(NOT cars)" will hit on documents that do not contain and 4. Similarly the search "(NOT trucks)" will hit on Documents 1 and 4. Applying the A searches (which is Search B) will hit on the documents that are common to these two s Please write a Python program to De Morgan's Law via document search. You can use four text files as document 1, 2 3 and 4. Search A: NOT (cars OR trucks) et A list of all docs (a, b, c, d) et B list of docs with trucks (a, c) et C = list of docs with cars (b, c) et D = list of docs with cars or trucks (a, b, c) et E = not D (d)
Types of Loop
Loops are the elements of programming in which a part of code is repeated a particular number of times. Loop executes the series of statements many times till the conditional statement becomes false.
Loops
Any task which is repeated more than one time is called a loop. Basically, loops can be divided into three types as while, do-while and for loop. There are so many programming languages like C, C++, JAVA, PYTHON, and many more where looping statements can be used for repetitive execution.
While Loop
Loop is a feature in the programming language. It helps us to execute a set of instructions regularly. The block of code executes until some conditions provided within that Loop are true.
I really need help with this I submitted it multiple times can someone help?
![De Morgan’s laws commonly apply to text searching using Boolean operators AND, OR, and NOT. Consider a set of documents containing the words “cars” and “trucks”. De Morgan’s laws hold that these two searches will return the same set of documents:
**Search A:** NOT (cars OR trucks)
**Search B:** (NOT cars) AND (NOT trucks)
The corpus of documents containing “cars” or “trucks” can be represented by four documents:
1. Document 1: Contains only the word “cars”.
2. Document 2: Contains only “trucks”.
3. Document 3: Contains both “cars” and “trucks”.
4. Document 4: Contains neither “cars” nor “trucks”.
To evaluate Search A, clearly the search “(cars OR trucks)” will hit on Documents 1, 2, and 3. So the negation of that search (which is Search A) will hit everything else, which is Document 4.
Evaluating Search B, the search “(NOT cars)” will hit on documents that do not contain “cars”, which is Documents 2 and 4. Similarly the search “(NOT trucks)” will hit on Documents 1 and 4. Applying the AND operator to these two searches (which is Search B) will hit on the documents that are common to these two searches, which is Document 4.
Please write a Python program to De Morgan's Law via document search.
You can use four text files as document 1, 2, 3 and 4.
```python
# Search A: NOT (cars OR trucks)
set A = list of all docs (a, b, c, d)
set B = list of docs with trucks (a, c)
set C = list of docs with cars (b, c)
set D = list of docs with cars or trucks (a, b, c)
set E = not D (d)
# Search B: (NOT cars) AND (NOT trucks)
set A = list of all docs (a, b, c, d)
set B = not cars (a,d)
set C = not trucks (b,d)
set D = (not Cars AND not Trucks) (d)
d = {}
d['cars'] = [a, c]
d['trucks'] = [b, c]
def get_word_count(word, file):
n = 0](/v2/_next/image?url=https%3A%2F%2Fcontent.bartleby.com%2Fqna-images%2Fquestion%2F10aa3c60-2143-49eb-a42e-7e7e91160ba4%2F0cecf033-867b-4e07-8cb4-c4a9a01793c5%2F40ebmn_processed.png&w=3840&q=75)
![**Code Snippet for Educational Purposes**
This Python script demonstrates the implementation of the **Single Responsibility Principle (SRP)** in software design. The SRP suggests dividing a main problem into smaller, logical sub-problems, solving each, and then using "glue code" to integrate these solutions into a functional program.
### Code Explanation:
1. **lines = x.readlines()**
- Reads lines from a file `x`.
2. **for line in lines:**
- Iterates over each line in the file.
3. **if line.find(word) > -1:**
- Checks if the `word` exists in the line.
4. **n+=1**
- Increments a counter `n` when the `word` is found.
5. **return n**
- Returns the count of lines containing the `word`.
### Main Function (`main()`):
1. **Read File**
- Reads and returns all lines from a file.
2. **Tokenize and Create Histogram**
- Tokenizes each line and creates a histogram (frequency count) for each word.
- Example:
- Initial Histogram: `h = {'this':[1, filename], 'is':[1, filename], 'my':[1, filename], 'car':[1, filename]}`
- Aggregated: `a = {'this':[2, [filename1, filename2]], 'is':[2, [filename1, filename2]], 'my':2, 'car':1, 'truck':1}`
3. **Aggregate Histograms**
- Combines histograms from different files.
4. **Glue Code**
- **Look for 'cars' and 'trucks'**:
```python
k = ['cars', 'trucks']
cars = []
trucks = []
if k[0] in a.keys():
cars += (a[k[0]][1])
if k[1] in a.keys():
trucks += (a[k[1]][1])
```
- This segment searches for the presence of 'cars' and 'trucks' within the aggregated data and appends occurrences to respective lists.
This example illustrates how breaking down a problem into smaller components and strategically recombining them facilitates a cleaner and more manageable code structure, embodying the essence of the SRP.](/v2/_next/image?url=https%3A%2F%2Fcontent.bartleby.com%2Fqna-images%2Fquestion%2F10aa3c60-2143-49eb-a42e-7e7e91160ba4%2F0cecf033-867b-4e07-8cb4-c4a9a01793c5%2Fmjj4tip_processed.png&w=3840&q=75)

Trending now
This is a popular solution!
Step by step
Solved in 3 steps

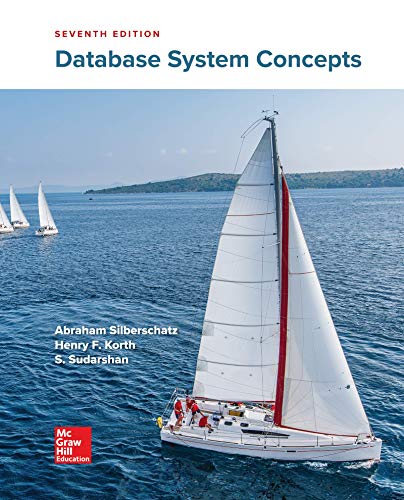
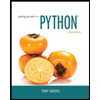
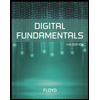
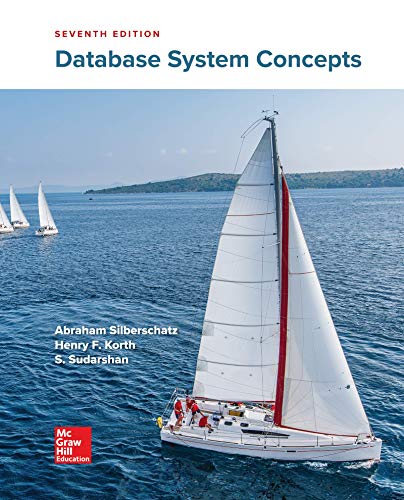
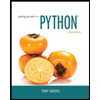
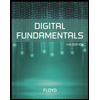
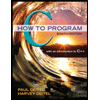
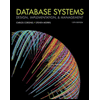
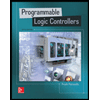