Data was collected on 54 observations on a response of interest, y, and four potential predictor variables x1, x2, x3, and x4. The output from regression analyses of the data is attached to the end of the page. f) Using the regression sums of squares information, test the null hypothesis H0:b2 = b4 = 0 for the full model. (Calculate an F statistic, obtain a tabled F value, and report the conclusion of your test, use a = .05).
Data was collected on 54 observations on a response of interest, y, and four potential predictor variables x1, x2, x3, and x4. The output from regression analyses of the data is attached to the end of the page. f) Using the regression sums of squares information, test the null hypothesis H0:b2 = b4 = 0 for the full model. (Calculate an F statistic, obtain a tabled F value, and report the conclusion of your test, use a = .05).
MATLAB: An Introduction with Applications
6th Edition
ISBN:9781119256830
Author:Amos Gilat
Publisher:Amos Gilat
Chapter1: Starting With Matlab
Section: Chapter Questions
Problem 1P
Related questions
Question
- Data was collected on 54 observations on a response of interest, y, and four potential predictor variables x1, x2, x3, and x4. The output from regression analyses of the data is attached to the end of the page. f) Using the regression sums of squares information, test the null hypothesis H0:b2 = b4 = 0 for the full model. (Calculate an F statistic, obtain a tabled F value, and report the conclusion of your test, use a = .05).

Transcribed Image Text:**Results for the Best Subsets Regression Analysis**
This analysis presents the outcomes of various regression models used to identify the best subsets of explanatory variables for predicting the dependent variable \( y \). The analysis uses the R-Square Selection Method, detailing several statistical measures for each model:
- **Model**: Number of variables included.
- **R-Square**: Proportion of variance in \( y \) explained by the model.
- **C(p)**: Mallow's C statistic, a criterion for model selection.
- **MSE**: Mean Squared Error.
- **BIC**: Bayesian Information Criterion.
- **Variables in Model**: Specific predictor variables used.
### Detailed Results:
#### Model 1 (Single Variable Models)
1. R-Square: 0.5259, Variables: x4
- R-Square: 0.4414, Variables: x3
- R-Square: 0.3447, Variables: x2
- R-Square: 0.1257, Variables: x1
#### Model 2 (Two Variable Models)
- R-Square: 0.8049, Variables: x2, x3
- R-Square: 0.6847, Variables: x3, x4
- R-Square: 0.6521, Variables: x1, x3
- R-Square: 0.6440, Variables: x2, x4
#### Model 3 (Three Variable Models)
- R-Square: 0.9712, Variables: x1, x2, x3
- R-Square: 0.8758, Variables: x2, x3, x4
- R-Square: 0.7215, Variables: x1, x2, x4
- R-Square: 0.6449, Variables: x1, x2, x4
#### Model 4 (Four Variable Model)
- R-Square: 0.9712, Variables: x1, x2, x3, x4
---
**Summary of SSR and SSE for Various Models:**
- **Terms in Model**: Indicates the predictor variables included.
- **SSR (Sum of Squares for Regression)**: Measures variation explained by the model.
- **SSE (Sum of Squares for Error or Residuals)**: Measures unexplained variation.
| Terms
Expert Solution

This question has been solved!
Explore an expertly crafted, step-by-step solution for a thorough understanding of key concepts.
This is a popular solution!
Trending now
This is a popular solution!
Step by step
Solved in 3 steps with 1 images

Recommended textbooks for you
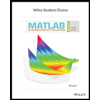
MATLAB: An Introduction with Applications
Statistics
ISBN:
9781119256830
Author:
Amos Gilat
Publisher:
John Wiley & Sons Inc
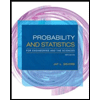
Probability and Statistics for Engineering and th…
Statistics
ISBN:
9781305251809
Author:
Jay L. Devore
Publisher:
Cengage Learning
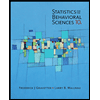
Statistics for The Behavioral Sciences (MindTap C…
Statistics
ISBN:
9781305504912
Author:
Frederick J Gravetter, Larry B. Wallnau
Publisher:
Cengage Learning
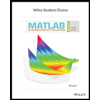
MATLAB: An Introduction with Applications
Statistics
ISBN:
9781119256830
Author:
Amos Gilat
Publisher:
John Wiley & Sons Inc
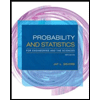
Probability and Statistics for Engineering and th…
Statistics
ISBN:
9781305251809
Author:
Jay L. Devore
Publisher:
Cengage Learning
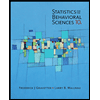
Statistics for The Behavioral Sciences (MindTap C…
Statistics
ISBN:
9781305504912
Author:
Frederick J Gravetter, Larry B. Wallnau
Publisher:
Cengage Learning
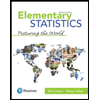
Elementary Statistics: Picturing the World (7th E…
Statistics
ISBN:
9780134683416
Author:
Ron Larson, Betsy Farber
Publisher:
PEARSON
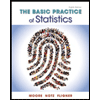
The Basic Practice of Statistics
Statistics
ISBN:
9781319042578
Author:
David S. Moore, William I. Notz, Michael A. Fligner
Publisher:
W. H. Freeman
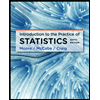
Introduction to the Practice of Statistics
Statistics
ISBN:
9781319013387
Author:
David S. Moore, George P. McCabe, Bruce A. Craig
Publisher:
W. H. Freeman