Credit Rating (feature1) Liabiltiy or not (Target) Yes No Total Excellent 3 1 4 Good 4 2 6 Poor 0 4 4 Total 7 7 14 Balance (feature2) Liabiltiy or not (Target) Yes No Total >50K 2 6 8 < 50 K 5 1 6 Total 7 7 14 Liability Tables Refer to the ‘Liability’ tables above, the target variable is Liability which can take on two values “Yes” and “No” and we 2 features: Credit Rating (which can take on values “Excellent”, “Good” and “Poor”), and Balance (which can take on values “> 50K”, “< 50K”). There are 14 observations in total. See the above liability table for different figures of ‘excellent’, ‘good’, ‘poor’ credit ratings and ‘Yes’, ‘No’ liability class. Use decision tree algorithm to work out which feature provides more information or reduces more uncertainty about our target variable out of the two using the concepts of entropy and information Gain.
Credit Rating (feature1) |
Liabiltiy or not (Target) |
||
Yes |
No |
Total |
|
Excellent |
3 |
1 |
4 |
Good |
4 |
2 |
6 |
Poor |
0 |
4 |
4 |
Total |
7 |
7 |
14 |
Balance (feature2) |
Liabiltiy or not (Target) |
||
Yes |
No |
Total |
|
>50K |
2 |
6 |
8 |
< 50 K |
5 |
1 |
6 |
Total |
7 |
7 |
14 |
Liability Tables
Refer to the ‘Liability’ tables above, the target variable is Liability which can take on two values “Yes” and “No” and we 2 features: Credit Rating (which can take on values “Excellent”, “Good” and “Poor”), and Balance (which can take on values “> 50K”, “< 50K”). There are 14 observations in total. See the above liability table for different figures of ‘excellent’, ‘good’, ‘poor’ credit ratings and ‘Yes’, ‘No’ liability class. Use decision tree
Please answer ASAP, will upvote! Thank you.

Step by step
Solved in 2 steps with 2 images

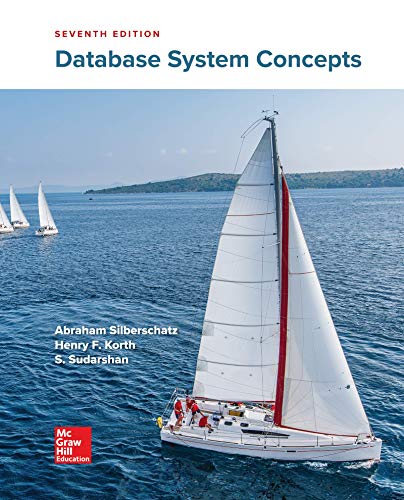
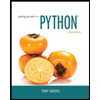
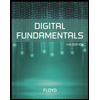
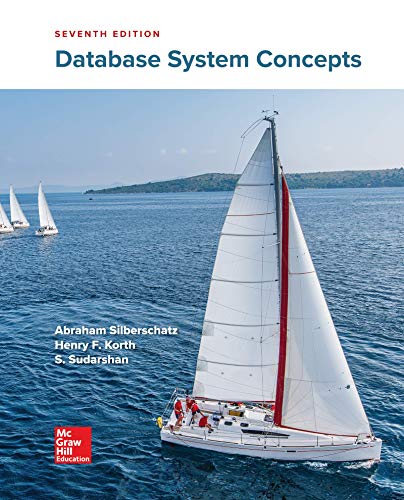
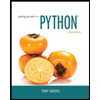
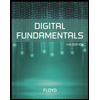
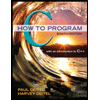
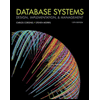
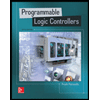