counting occurrences of one part of speech following another in a training corpus, counting occurrences of words together with parts of speech in a training corpus, • relative frequency estimation with smoothing, Gndine the hoot gogm ne of norte of anooch for o liat of worda in ho toat 0orn110
counting occurrences of one part of speech following another in a training corpus, counting occurrences of words together with parts of speech in a training corpus, • relative frequency estimation with smoothing, Gndine the hoot gogm ne of norte of anooch for o liat of worda in ho toat 0orn110
Computer Networking: A Top-Down Approach (7th Edition)
7th Edition
ISBN:9780133594140
Author:James Kurose, Keith Ross
Publisher:James Kurose, Keith Ross
Chapter1: Computer Networks And The Internet
Section: Chapter Questions
Problem R1RQ: What is the difference between a host and an end system? List several different types of end...
Related questions
Question
![Viterbi algorithm
You will develop a first-order HMM (Hidden Markov Model) for POS (part of speech)
tagging in Python. This involves:
• counting occurrences of one part of speech following another in a training corpus,
• counting occurrences of words together with parts of speech in a training corpus,
• relative frequency estimation with smoothing,
• finding the best sequence of parts of speech for a list of words in the test corpus,
according to a HMM model with smoothed probabilities,
• computing the accuracy, that is, the percentage of parts of speech that is guessed
correctly.
As discussed in the lectures, smoothing is necessary to avoid zero probabilities for
events that were not witnessed in the training corpus. Rather than implementing a
form of smoothing yourself, you can for this assignment take the implementation of
Witten-Bell smoothing in NLTK (among the forms of smoothing in NLTK, this seems
to be the most robust one). An example of use for emission probabilities is in file
smoothing.py; one can similarly apply smoothing to transition probabilities.
Run your application on the English (EWT) training and testing corpora. You
should get an accuracy above 89%. If your accuracy is much lower, then you are
probably doing something wrong.
Comparisons between languages
Investigate, by visual inspection and by computational means, the upos parts of speech
in different treebanks from Universal Dependencies. (Take a few languages based on
your own interests, but no more than about 10. Go for the quality of your submission,
not quantity!) Two examples of specific questions you could address:
• Which of the chosen languages have a rich morphology and which have a poor
morphology?
• How similar are the chosen languages, in terms of bigram models of their parts
of speech?
For the first question, know that you can access the lemma of a token by
token ['lemma']. What can you say about the relation between forms and lemmas
in the case of languages with rich morphology?
2
For the second question, consider that the transition probabilities of two related
languages may be very similar, even though the emission probabilities may be incom-
parable due to the mostly disjoint vocabularies. How could we measure the similarity
between two bigram models trained from corpora?
Feel free to think of further questions to address. It is worth noting that next to the
('universal') upos tags, the Universal Dependencies treebanks sometimes also contain
language-specific (xpos) tags.](/v2/_next/image?url=https%3A%2F%2Fcontent.bartleby.com%2Fqna-images%2Fquestion%2F56b2b3f8-2115-49a7-ae10-602fd1cf0887%2F82f9b13b-5c13-4359-9807-9072566b3145%2F6pfp0z5_processed.png&w=3840&q=75)
Transcribed Image Text:Viterbi algorithm
You will develop a first-order HMM (Hidden Markov Model) for POS (part of speech)
tagging in Python. This involves:
• counting occurrences of one part of speech following another in a training corpus,
• counting occurrences of words together with parts of speech in a training corpus,
• relative frequency estimation with smoothing,
• finding the best sequence of parts of speech for a list of words in the test corpus,
according to a HMM model with smoothed probabilities,
• computing the accuracy, that is, the percentage of parts of speech that is guessed
correctly.
As discussed in the lectures, smoothing is necessary to avoid zero probabilities for
events that were not witnessed in the training corpus. Rather than implementing a
form of smoothing yourself, you can for this assignment take the implementation of
Witten-Bell smoothing in NLTK (among the forms of smoothing in NLTK, this seems
to be the most robust one). An example of use for emission probabilities is in file
smoothing.py; one can similarly apply smoothing to transition probabilities.
Run your application on the English (EWT) training and testing corpora. You
should get an accuracy above 89%. If your accuracy is much lower, then you are
probably doing something wrong.
Comparisons between languages
Investigate, by visual inspection and by computational means, the upos parts of speech
in different treebanks from Universal Dependencies. (Take a few languages based on
your own interests, but no more than about 10. Go for the quality of your submission,
not quantity!) Two examples of specific questions you could address:
• Which of the chosen languages have a rich morphology and which have a poor
morphology?
• How similar are the chosen languages, in terms of bigram models of their parts
of speech?
For the first question, know that you can access the lemma of a token by
token ['lemma']. What can you say about the relation between forms and lemmas
in the case of languages with rich morphology?
2
For the second question, consider that the transition probabilities of two related
languages may be very similar, even though the emission probabilities may be incom-
parable due to the mostly disjoint vocabularies. How could we measure the similarity
between two bigram models trained from corpora?
Feel free to think of further questions to address. It is worth noting that next to the
('universal') upos tags, the Universal Dependencies treebanks sometimes also contain
language-specific (xpos) tags.
Expert Solution

This question has been solved!
Explore an expertly crafted, step-by-step solution for a thorough understanding of key concepts.
Step by step
Solved in 2 steps with 2 images

Recommended textbooks for you
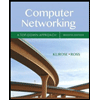
Computer Networking: A Top-Down Approach (7th Edi…
Computer Engineering
ISBN:
9780133594140
Author:
James Kurose, Keith Ross
Publisher:
PEARSON
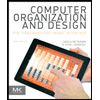
Computer Organization and Design MIPS Edition, Fi…
Computer Engineering
ISBN:
9780124077263
Author:
David A. Patterson, John L. Hennessy
Publisher:
Elsevier Science
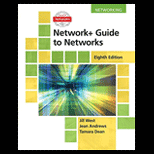
Network+ Guide to Networks (MindTap Course List)
Computer Engineering
ISBN:
9781337569330
Author:
Jill West, Tamara Dean, Jean Andrews
Publisher:
Cengage Learning
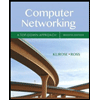
Computer Networking: A Top-Down Approach (7th Edi…
Computer Engineering
ISBN:
9780133594140
Author:
James Kurose, Keith Ross
Publisher:
PEARSON
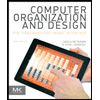
Computer Organization and Design MIPS Edition, Fi…
Computer Engineering
ISBN:
9780124077263
Author:
David A. Patterson, John L. Hennessy
Publisher:
Elsevier Science
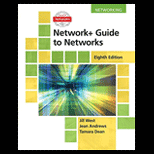
Network+ Guide to Networks (MindTap Course List)
Computer Engineering
ISBN:
9781337569330
Author:
Jill West, Tamara Dean, Jean Andrews
Publisher:
Cengage Learning
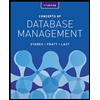
Concepts of Database Management
Computer Engineering
ISBN:
9781337093422
Author:
Joy L. Starks, Philip J. Pratt, Mary Z. Last
Publisher:
Cengage Learning
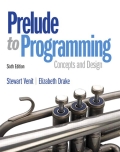
Prelude to Programming
Computer Engineering
ISBN:
9780133750423
Author:
VENIT, Stewart
Publisher:
Pearson Education
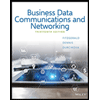
Sc Business Data Communications and Networking, T…
Computer Engineering
ISBN:
9781119368830
Author:
FITZGERALD
Publisher:
WILEY