Context for remaining questions Natural Language Processing (NLP) is the field of computer science that is concerned with using computers to make sense of language as it is spoken naturally. One of the most commonly used formulas is "tf-idf". We use tf-idf to quantify how important a particular word -- also called a term in this context -- is to the document it appears in. "tf" stands for "term frequency". We calculate it by taking the number of times a term appears in the document, and dividing it by the total term count (just like the word count of an essay), like so: tf = (number of times the term appeared in the document)/(total word count for the document) "idf" stands for "inverse document frequency". Document frequency is simply the number of documents the term appeared in at least once in your entire document collection. A document collection could be a collection of books or articles, or all of the webpages returned by a search result, or all the reviews on a single product on Amazon, etc. This term helps us lower the importance of words that are so common in this collection that it's meaningless that they are present in a document. For instance, most documents will contain common words like "the" or "and" many times. That doesn't mean that the document is about those words. Document frequency is calculated like so: df = (number of documents the term appears in at least once)/(total number of documents in the collection) To get inverse document frequency, you just divide one by the document frequency like so: idf = 1/df = (total number of documents in the collection)/(number of documents the term appears in at least once) Finally, to calculate tf-idf, you multiply tf by idf. This is mathematically identical to dividing tf by df. Question 2 a) Write code that opens the file "term_data.txt" and loads data into the following variables, in this order: termCount = number of times the term appeared in the document length = total word count for the document docCount = number of documents the term appears in at least once totalDocs = total number of documents in the collection Hint: You will need to include the right header file to complete this question. b) Continue by adding code that calculates tf, idf, and tf-idf, and prints all three to the console.
Context for remaining questions Natural Language Processing (NLP) is the field of computer science that is concerned with using computers to make sense of language as it is spoken naturally. One of the most commonly used formulas is "tf-idf". We use tf-idf to quantify how important a particular word -- also called a term in this context -- is to the document it appears in. "tf" stands for "term frequency". We calculate it by taking the number of times a term appears in the document, and dividing it by the total term count (just like the word count of an essay), like so: tf = (number of times the term appeared in the document)/(total word count for the document) "idf" stands for "inverse document frequency". Document frequency is simply the number of documents the term appeared in at least once in your entire document collection. A document collection could be a collection of books or articles, or all of the webpages returned by a search result, or all the reviews on a single product on Amazon, etc. This term helps us lower the importance of words that are so common in this collection that it's meaningless that they are present in a document. For instance, most documents will contain common words like "the" or "and" many times. That doesn't mean that the document is about those words. Document frequency is calculated like so: df = (number of documents the term appears in at least once)/(total number of documents in the collection) To get inverse document frequency, you just divide one by the document frequency like so: idf = 1/df = (total number of documents in the collection)/(number of documents the term appears in at least once) Finally, to calculate tf-idf, you multiply tf by idf. This is mathematically identical to dividing tf by df. Question 2 a) Write code that opens the file "term_data.txt" and loads data into the following variables, in this order: termCount = number of times the term appeared in the document length = total word count for the document docCount = number of documents the term appears in at least once totalDocs = total number of documents in the collection Hint: You will need to include the right header file to complete this question. b) Continue by adding code that calculates tf, idf, and tf-idf, and prints all three to the console.
Database System Concepts
7th Edition
ISBN:9780078022159
Author:Abraham Silberschatz Professor, Henry F. Korth, S. Sudarshan
Publisher:Abraham Silberschatz Professor, Henry F. Korth, S. Sudarshan
Chapter1: Introduction
Section: Chapter Questions
Problem 1PE
Related questions
Question
100%
i added the term_data.txt screenshot so that it would be easier for you to see it then make the txt file from your own PC

Transcribed Image Text:term_data - Notepad
File Edit
View
12 745 1459 1000000

Transcribed Image Text:Context for remaining questions
Natural Language Processing (NLP) is the field of computer science that is concerned with using computers to make sense of language as it is spoken naturally. One of the most commonly used formulas is "tf-idf". We use tf-idf to quantify how
important a particular word -- also called a term in this context -- is to the document it appears in.
"tf" stands for "term frequency". We calculate it by taking the number of times a term appears in the document, and dividing it by the total term count (just like the word count of an essay), like so:
tf = (number of times the term appeared in the document)/(total word count for the document)
"idf" stands for "inverse document frequency". Document frequency is simply the number of documents the term appeared in at least once in your entire document collection. A document collection could be a collection of books or articles, or all
of the webpages returned by a search result, or all the reviews on a single product on Amazon, etc. This term helps us lower the importance of words that are so common in this collection that it's meaningless that they are present in a document.
For instance, most documents will contain common words like "the" or "and" many times. That doesn't mean that the document is about those words.
Document frequency is calculated like so:
df = (number of documents the term appears in at least once)/(total number of documents in the collection)
To get inverse document frequency, you just divide one by the document frequency like so:
idf = 1/df = (total number of documents in the collection)/(number of documents the term appears in at least once)
Finally, to calculate tf-idf, you multiply tf by idf. This is mathematically identical to dividing tf by df.
Question 2
a) Write code that opens the file "term_data.bit" and loads data into the following variables, in this order:
termCount = number of times the term appeared in the document
length = total word count for the document
docCount = number of documents the term appears in at least once
totalDocs = total number of documents in the collection
Hint: You will need to include the right header file to complete this question.
b) Continue by adding code that calculates tf, idf, and tf-idf, and prints all three to the console.
Expert Solution

This question has been solved!
Explore an expertly crafted, step-by-step solution for a thorough understanding of key concepts.
Step by step
Solved in 3 steps with 2 images

Knowledge Booster
Learn more about
Need a deep-dive on the concept behind this application? Look no further. Learn more about this topic, computer-science and related others by exploring similar questions and additional content below.Recommended textbooks for you
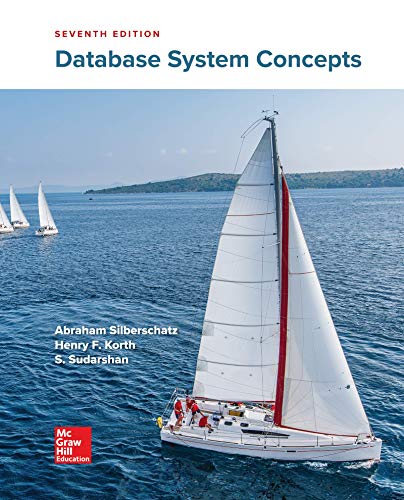
Database System Concepts
Computer Science
ISBN:
9780078022159
Author:
Abraham Silberschatz Professor, Henry F. Korth, S. Sudarshan
Publisher:
McGraw-Hill Education
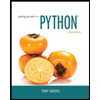
Starting Out with Python (4th Edition)
Computer Science
ISBN:
9780134444321
Author:
Tony Gaddis
Publisher:
PEARSON
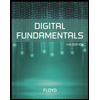
Digital Fundamentals (11th Edition)
Computer Science
ISBN:
9780132737968
Author:
Thomas L. Floyd
Publisher:
PEARSON
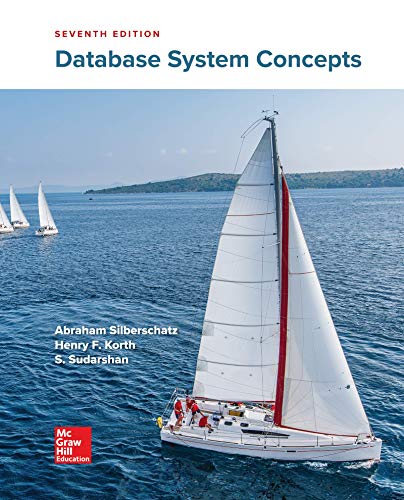
Database System Concepts
Computer Science
ISBN:
9780078022159
Author:
Abraham Silberschatz Professor, Henry F. Korth, S. Sudarshan
Publisher:
McGraw-Hill Education
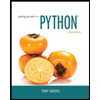
Starting Out with Python (4th Edition)
Computer Science
ISBN:
9780134444321
Author:
Tony Gaddis
Publisher:
PEARSON
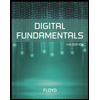
Digital Fundamentals (11th Edition)
Computer Science
ISBN:
9780132737968
Author:
Thomas L. Floyd
Publisher:
PEARSON
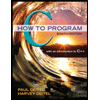
C How to Program (8th Edition)
Computer Science
ISBN:
9780133976892
Author:
Paul J. Deitel, Harvey Deitel
Publisher:
PEARSON
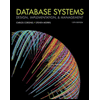
Database Systems: Design, Implementation, & Manag…
Computer Science
ISBN:
9781337627900
Author:
Carlos Coronel, Steven Morris
Publisher:
Cengage Learning
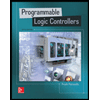
Programmable Logic Controllers
Computer Science
ISBN:
9780073373843
Author:
Frank D. Petruzella
Publisher:
McGraw-Hill Education