Consider the lasso criterion as seen in the lectures L= ½YTY - BTXTY +16TXTXB+X||B||1. In this problem, you will consider the case with only one explanatory variable. The columns X and Y are centered so there is no intercept in the model. The parameter ẞ is a scalar and so are the quantities XTX,XTY and YTY. These scalars have values XTX = 2.5, XTY = -0.5 and YTY = 2.5. A) Write the ordinary least squares estimate B = -0.2 B) Using the given quantities and the result of B, write the lasso criterion as a function of ẞ and A (both scalars). The criterion is L 1.25 +0.58 +1.2582 - AB = C) By doing derivative of L and solving the corresponding system, determine the lasso coefficient as a function of X. That is BL = BL(A)=(-0.5)/2.5 = 0.4 - 0.2 D) The value of A at which the path (A) shrinks to zero is A=0.5 Consider the lasso criterion as seen in the lectures L = YTY - BTXTY+8TXTXB+A||||1. In this problem, you will consider the case with only one explanatory variable. The columns X and Y are centered so there is no intercept in the model. The parameter ẞ is a scalar and so are the quantities XTX,XTY and YTY. These scalars have values XTX 2.5, XTY = -0.5 and YTY = 2.5. = A) Write the ordinary least squares estimate B = B) Using the given quantities and the result of B, write the lasso criterion as a function of ẞ and A (both scalars). The criterion is L C) By doing derivative of L and solving the corresponding system, determine the lasso coefficient as a function of A. That is BL = BL (A)= D) The value of X at which the path (A) shrinks to zero is >= =
Consider the lasso criterion as seen in the lectures L= ½YTY - BTXTY +16TXTXB+X||B||1. In this problem, you will consider the case with only one explanatory variable. The columns X and Y are centered so there is no intercept in the model. The parameter ẞ is a scalar and so are the quantities XTX,XTY and YTY. These scalars have values XTX = 2.5, XTY = -0.5 and YTY = 2.5. A) Write the ordinary least squares estimate B = -0.2 B) Using the given quantities and the result of B, write the lasso criterion as a function of ẞ and A (both scalars). The criterion is L 1.25 +0.58 +1.2582 - AB = C) By doing derivative of L and solving the corresponding system, determine the lasso coefficient as a function of X. That is BL = BL(A)=(-0.5)/2.5 = 0.4 - 0.2 D) The value of A at which the path (A) shrinks to zero is A=0.5 Consider the lasso criterion as seen in the lectures L = YTY - BTXTY+8TXTXB+A||||1. In this problem, you will consider the case with only one explanatory variable. The columns X and Y are centered so there is no intercept in the model. The parameter ẞ is a scalar and so are the quantities XTX,XTY and YTY. These scalars have values XTX 2.5, XTY = -0.5 and YTY = 2.5. = A) Write the ordinary least squares estimate B = B) Using the given quantities and the result of B, write the lasso criterion as a function of ẞ and A (both scalars). The criterion is L C) By doing derivative of L and solving the corresponding system, determine the lasso coefficient as a function of A. That is BL = BL (A)= D) The value of X at which the path (A) shrinks to zero is >= =
Algebra & Trigonometry with Analytic Geometry
13th Edition
ISBN:9781133382119
Author:Swokowski
Publisher:Swokowski
Chapter2: Equations And Inequalities
Section2.3: Quadratic Equations
Problem 16E
Related questions
Question
these are the questions and solutions. Please explain and show the method of the solutions

Transcribed Image Text:Consider the lasso criterion as seen in the lectures L= ½YTY - BTXTY +16TXTXB+X||B||1. In this
problem, you will consider the case with only one explanatory variable. The columns X and Y are centered
so there is no intercept in the model.
The parameter ẞ is a scalar and so are the quantities XTX,XTY and YTY. These scalars have values
XTX = 2.5, XTY = -0.5 and YTY = 2.5.
A) Write the ordinary least squares estimate B
=
-0.2
B) Using the given quantities and the result of B, write the lasso criterion as a function of ẞ and A (both
scalars). The criterion is L 1.25 +0.58 +1.2582 - AB
=
C) By doing derivative of L and solving the corresponding system, determine the lasso coefficient as a
function of X. That is BL = BL(A)=(-0.5)/2.5 = 0.4 - 0.2
D) The value of A at which the path (A) shrinks to zero is A=0.5

Transcribed Image Text:Consider the lasso criterion as seen in the lectures L = YTY - BTXTY+8TXTXB+A||||1. In this
problem, you will consider the case with only one explanatory variable. The columns X and Y are centered
so there is no intercept in the model.
The parameter ẞ is a scalar and so are the quantities XTX,XTY and YTY. These scalars have values
XTX 2.5, XTY = -0.5 and YTY = 2.5.
=
A) Write the ordinary least squares estimate B =
B) Using the given quantities and the result of B, write the lasso criterion as a function of ẞ and A (both
scalars). The criterion is L
C) By doing derivative of L and solving the corresponding system, determine the lasso coefficient as a
function of A. That is BL = BL (A)=
D) The value of X at which the path (A) shrinks to zero is >=
=
Expert Solution

This question has been solved!
Explore an expertly crafted, step-by-step solution for a thorough understanding of key concepts.
Step by step
Solved in 2 steps with 5 images

Recommended textbooks for you
Algebra & Trigonometry with Analytic Geometry
Algebra
ISBN:
9781133382119
Author:
Swokowski
Publisher:
Cengage
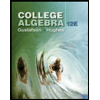
College Algebra (MindTap Course List)
Algebra
ISBN:
9781305652231
Author:
R. David Gustafson, Jeff Hughes
Publisher:
Cengage Learning
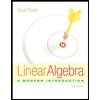
Linear Algebra: A Modern Introduction
Algebra
ISBN:
9781285463247
Author:
David Poole
Publisher:
Cengage Learning
Algebra & Trigonometry with Analytic Geometry
Algebra
ISBN:
9781133382119
Author:
Swokowski
Publisher:
Cengage
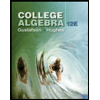
College Algebra (MindTap Course List)
Algebra
ISBN:
9781305652231
Author:
R. David Gustafson, Jeff Hughes
Publisher:
Cengage Learning
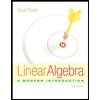
Linear Algebra: A Modern Introduction
Algebra
ISBN:
9781285463247
Author:
David Poole
Publisher:
Cengage Learning
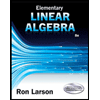
Elementary Linear Algebra (MindTap Course List)
Algebra
ISBN:
9781305658004
Author:
Ron Larson
Publisher:
Cengage Learning
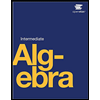