Consider the case of a simple Markov Decision Process (MDP) with a discount factor gamma = 1. The MDP has three states (x, y, and z), with rewards -1, -2, 0, respectively. State z is considered a terminal state. In states and y there are two possible actions: a₁ and a2. The transition model is as follows: In state x, action a1 moves the agent to state y with probability 0.9 and makes the agent stay put with probability 0.1. In state y, action a1 moves the agent to state with probability 0.9 and makes the agent stay put with probability 0.1. In either state or state y, action a2 moves the agent to state z with probability 0.1 and makes the agent stay put with probability 0.9. Please answer the following questions: Draw a picture of the MDP What can be determined qualitatively about the optimal policy in states x and y? Apply the policy iteration algorithm discuss in class, showing each step in full, to determine the optimal policy and the values of states and y. Assume that the initial policy has action a2 in both states. What happens to policy iteration if instead, the initial policy has action a1 in both states? Does discounting help? Does the optimal policy depend on the discount factor?
Consider the case of a simple Markov Decision Process (MDP) with a discount factor gamma = 1. The MDP has three states (x, y, and z), with rewards -1, -2, 0, respectively. State z is considered a terminal state. In states and y there are two possible actions: a₁ and a2. The transition model is as follows: In state x, action a1 moves the agent to state y with probability 0.9 and makes the agent stay put with probability 0.1. In state y, action a1 moves the agent to state with probability 0.9 and makes the agent stay put with probability 0.1. In either state or state y, action a2 moves the agent to state z with probability 0.1 and makes the agent stay put with probability 0.9. Please answer the following questions: Draw a picture of the MDP What can be determined qualitatively about the optimal policy in states x and y? Apply the policy iteration algorithm discuss in class, showing each step in full, to determine the optimal policy and the values of states and y. Assume that the initial policy has action a2 in both states. What happens to policy iteration if instead, the initial policy has action a1 in both states? Does discounting help? Does the optimal policy depend on the discount factor?
Computer Networking: A Top-Down Approach (7th Edition)
7th Edition
ISBN:9780133594140
Author:James Kurose, Keith Ross
Publisher:James Kurose, Keith Ross
Chapter1: Computer Networks And The Internet
Section: Chapter Questions
Problem R1RQ: What is the difference between a host and an end system? List several different types of end...
Related questions
Question
Consider the case of a simple Markov Decision Process (MDP) with a discount factor gamma = 1. The MDP has three states (x, y, and z), with rewards -1, -2, 0, respectively. State z is considered a terminal state. In states and y there are two possible actions: a₁ and a2.
The transition model is as follows:
- In state x, action a1 moves the agent to state y with probability 0.9 and makes the agent stay put with probability 0.1.
- In state y, action a1 moves the agent to state with probability 0.9 and makes the agent stay put with probability 0.1.
- In either state or state y, action a2 moves the agent to state z with probability 0.1 and makes the agent stay put with probability 0.9.
Please answer the following questions:
- Draw a picture of the MDP
- What can be determined qualitatively about the optimal policy in states x and y?
- Apply the policy iteration
algorithm discuss in class, showing each step in full, to determine the optimal policy and the values of states and y. Assume that the initial policy has action a2 in both states. - What happens to policy iteration if instead, the initial policy has action a1 in both states? Does discounting help? Does the optimal policy depend on the discount factor?
Expert Solution

This question has been solved!
Explore an expertly crafted, step-by-step solution for a thorough understanding of key concepts.
This is a popular solution!
Trending now
This is a popular solution!
Step by step
Solved in 5 steps with 3 images

Recommended textbooks for you
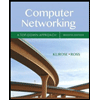
Computer Networking: A Top-Down Approach (7th Edi…
Computer Engineering
ISBN:
9780133594140
Author:
James Kurose, Keith Ross
Publisher:
PEARSON
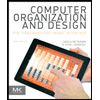
Computer Organization and Design MIPS Edition, Fi…
Computer Engineering
ISBN:
9780124077263
Author:
David A. Patterson, John L. Hennessy
Publisher:
Elsevier Science
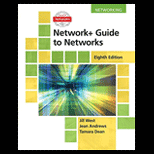
Network+ Guide to Networks (MindTap Course List)
Computer Engineering
ISBN:
9781337569330
Author:
Jill West, Tamara Dean, Jean Andrews
Publisher:
Cengage Learning
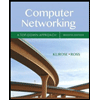
Computer Networking: A Top-Down Approach (7th Edi…
Computer Engineering
ISBN:
9780133594140
Author:
James Kurose, Keith Ross
Publisher:
PEARSON
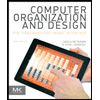
Computer Organization and Design MIPS Edition, Fi…
Computer Engineering
ISBN:
9780124077263
Author:
David A. Patterson, John L. Hennessy
Publisher:
Elsevier Science
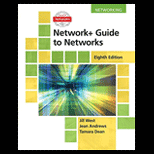
Network+ Guide to Networks (MindTap Course List)
Computer Engineering
ISBN:
9781337569330
Author:
Jill West, Tamara Dean, Jean Andrews
Publisher:
Cengage Learning
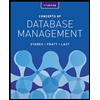
Concepts of Database Management
Computer Engineering
ISBN:
9781337093422
Author:
Joy L. Starks, Philip J. Pratt, Mary Z. Last
Publisher:
Cengage Learning
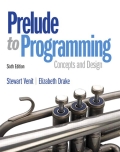
Prelude to Programming
Computer Engineering
ISBN:
9780133750423
Author:
VENIT, Stewart
Publisher:
Pearson Education
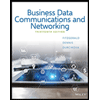
Sc Business Data Communications and Networking, T…
Computer Engineering
ISBN:
9781119368830
Author:
FITZGERALD
Publisher:
WILEY