Consider an automated audit log analysis tool. This tool could be used to distinguish “suspicious activities” from normal user behaviour on a system for some organisations. (This tool could be used for many more purposes as well.) Discuss in detail the pros and cons of the automated audit log analysis tool?
Consider an automated audit log analysis tool. This tool could be used to distinguish “suspicious activities” from normal user behaviour on a system for some organisations. (This tool could be used for many more purposes as well.)
Discuss in detail the pros and cons of the automated audit log analysis tool?

IT departments are under constant pressure to reduce downtime and improve performance. Traditionally, administrators used logs to determine the root cause of a problem, but logs have morphed into much more for modern data centers.
Logs generally contain much more information than a monitoring tool—which is often limited to metrics—to help troubleshoot IT issues. However, log files can be large - sometimes hundreds of thousands of lines and several GB. The average administrator's desktop can't even open a file of this size, let alone find value in it. Logs have essentially become a form of big data.
Several vendors, such as SolarWinds Loggly and Splunk, have AI-based log analysis and aggregation tools that use machine learning to offer deep insights into data center infrastructure and applications. These tools review logs from a variety of sources, such as servers, network devices, and applications, to create a more complete picture of what has happened or might happen in the IT environment. This ability to find the root cause of problems and prevent new ones is ideal for companies where poor performance or outages can quickly anger customers and cause them to look to other vendors.
Beware of hidden costs
However, audit log analysis tools come with both visible and hidden costs: the cost of the software itself and the fact that these products are highly dependent on hardware. These tools require a large amount of processor power to work effectively, which can quickly drain computing resources from other tasks. IT teams can schedule tasks after business hours to avoid this problem, but then the data collected may be out of date and therefore less useful.
Step by step
Solved in 5 steps

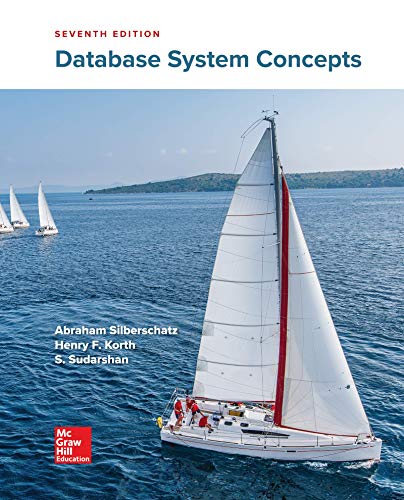
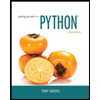
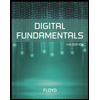
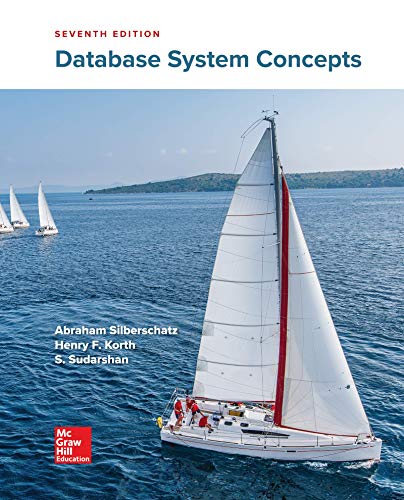
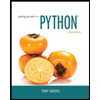
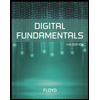
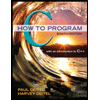
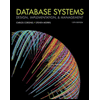
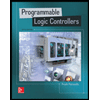