Compare and contrast R and Python as computational environments for analytics
Compare and contrast R and Python as computational environments for analytics

Both R and Python are the current torch-bearers of machine learning brigade. Both these languages can be used to initiate and modify algorithms in machine learning like classification, regression, clustering, neural networks and algorithms in artificial intelligence. Both of these languages have a different approach toward solving a given problem. Whether building a machine learning model or exploring the data to get some useful insights, both play an important role in understanding the data and extract facts from them.
The above graph indicates how one language is popular than other. Python is known to be most people and industries prefer python in most cases.
Comparing and contrasting methods:
Library repository
R has an exhaustive repository of libraries that are regularly updated in CRAN which is Comprehensive R Archive Network. It includes dplyr, mice, ggplot2 and many more. Python includes a pip package index that has all the libraries for Python. They include matplotlib, pandas, seaborn and many more. R has a more active user base which contributes to updating its huge database of 10,000 packages. Even Python includes a user community that updates its packages regularly but it is a bit complicated process compared to R.
Data handling
R has the power of doing data analysis without loading any package in its memory. There are many packages that are available in R that can be utilized to study data and get insights from them. In python, we need packages like numpy and pandas for creating a data frame and processing the data. Both languages are strong enough to carry out data computations and tasks which make it easy for users to make the most of data.
Step by step
Solved in 4 steps with 1 images

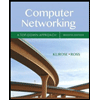
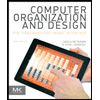
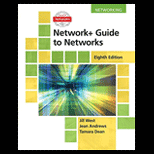
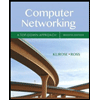
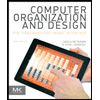
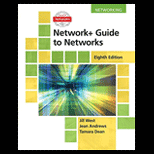
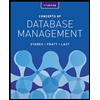
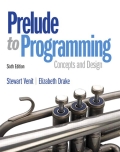
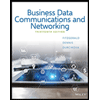