Coding questions: Implementation of ordinary least square error, Lasso regularization, and Ridge regularization for linear regression. (Note, you are not to use modules which provide these functions - that would be too easy (no linear_model.LinearRegression, for example) but rather create them yourselves. Due to time constraints, we are concerned more with functionality rather than efficiency. The dataset to use is the diabetes dataset available from the sklearn module (please refer to sklearn.datasets.load_diabetes — scikit-learn 1.4.1 documentation) (https://scikit-learn.org/stable/modules/generated/sklearn.datasets.load_diabetes.html#sklearn.datasets.load_diabetes) You are expected to use Jupyter notebooks/Colab and Python on this assignment. The diabetes dataset has 10 features, and you should use the first feature for the assignment. There are 442 samples in the dataset. Your goal is to take the first forty samples of the dataset to train your model, and the last forty samples are used for prediction. Please use the ordinary least square error to fit a linear regression model. Report the coefficients of the model, the mean squared error on the test data, and plot the output. Please use the Lasso regularization to fit a linear regression model. Report the coefficients of the model, the mean squared error on the test data, and plot the output. Please use the Ridge regularization to fit a linear regression model. Report the coefficients of the model, the mean squared error on the test data, and plot the output. Please plot the Ridge coefficients as a function of the regularization. Helpful tips: Please refer to Linear Regression Example — scikit-learn 1.4.1 documentation Links to an external site. for the coding assignment. https://scikit-learn.org/stable/auto_examples/linear_model/plot_ols.html#sphx-glr-auto-examples-linear-model-plot-ols-py
Coding questions:
Implementation of ordinary least square error, Lasso regularization, and Ridge regularization for linear regression.
(Note, you are not to use modules which provide these functions - that would be too easy (no linear_model.LinearRegression, for example) but rather create them yourselves. Due to time constraints, we are concerned more with functionality rather than efficiency.
The dataset to use is the diabetes dataset available from the sklearn module (please refer to sklearn.datasets.load_diabetes — scikit-learn 1.4.1 documentation) (https://scikit-learn.org/stable/modules/generated/sklearn.datasets.load_diabetes.html#sklearn.datasets.load_diabetes) You are expected to use Jupyter notebooks/Colab and Python on this assignment.
The diabetes dataset has 10 features, and you should use the first feature for the assignment. There are 442 samples in the dataset. Your goal is to take the first forty samples of the dataset to train your model, and the last forty samples are used for prediction.
Please use the ordinary least square error to fit a linear regression model. Report the coefficients of the model, the mean squared error on the test data, and plot the output.
Please use the Lasso regularization to fit a linear regression model. Report the coefficients of the model, the mean squared error on the test data, and plot the output.
Please use the Ridge regularization to fit a linear regression model. Report the coefficients of the model, the mean squared error on the test data, and plot the output.
Please plot the Ridge coefficients as a function of the regularization.
Helpful tips:
Please refer to Linear Regression Example — scikit-learn 1.4.1 documentation Links to an external site. for the coding assignment. https://scikit-learn.org/stable/auto_examples/linear_model/plot_ols.html#sphx-glr-auto-examples-linear-model-plot-ols-py

Step by step
Solved in 2 steps with 5 images

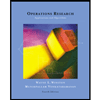
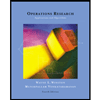
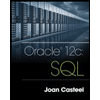
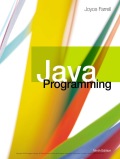