best predicted adult male value of for an 187 cm all i5 kg .
Continuous Probability Distributions
Probability distributions are of two types, which are continuous probability distributions and discrete probability distributions. A continuous probability distribution contains an infinite number of values. For example, if time is infinite: you could count from 0 to a trillion seconds, billion seconds, so on indefinitely. A discrete probability distribution consists of only a countable set of possible values.
Normal Distribution
Suppose we had to design a bathroom weighing scale, how would we decide what should be the range of the weighing machine? Would we take the highest recorded human weight in history and use that as the upper limit for our weighing scale? This may not be a great idea as the sensitivity of the scale would get reduced if the range is too large. At the same time, if we keep the upper limit too low, it may not be usable for a large percentage of the population!
![**Transcription for Educational Website**
*Title: Understanding Predictive Modeling in Biostatistics*
**Text:**
Heights (cm) and weights (kg) are measured for 100 randomly selected adult males, and range from heights of 130 to 193 cm and weights of 41 to 150 kg. Let the predictor variable \( x \) be the first variable given. The 100 paired measurements yield \(\bar{x} = 168.05\) cm, \(\bar{y} = 81.55\) kg, \( r = 0.223 \), P-value = 0.026, and \(\hat{y} = -102 + 1.17x \). Find the best predicted value of \(\hat{y}\) (weight) given an adult male who is 187 cm tall. Use a 0.10 significance level.
The best predicted value of \(\hat{y}\) for an adult male who is 187 cm tall is \([ \quad ]\) kg.
---
**Explanation of Findings:**
In this study, we are examining the relationship between height and weight among adult males. The predictor variable is height, and we are trying to predict the weight. From the linear regression model, \(\hat{y} = -102 + 1.17x\), we can determine the expected weight for a given height.
Using the given model, we can predict the weight of a male who is 187 cm tall. To do this, substitute 187 for \( x \) in the equation:
\[
\hat{y} = -102 + 1.17(187)
\]
Evaluate this equation to find the predicted weight.
Significance is tested at the 0.10 level, and given the P-value = 0.026, we see a statistically significant relationship between the variables within this model.
---
**Conclusion:**
For an adult male 187 cm tall, substitute into the regression model to predict weight, considering the statistical significance. This process demonstrates the application of biostatistical methods in predicting health-related metrics.](/v2/_next/image?url=https%3A%2F%2Fcontent.bartleby.com%2Fqna-images%2Fquestion%2F68a1b863-bec7-4997-8d33-dc81d338a946%2F1065a09d-f861-4278-943b-29f58e03a3c8%2Fg4grzxg_processed.jpeg&w=3840&q=75)

Regression analysis:
Regression analysis estimates the relationship among variables. That is, it estimates the relationship between one dependent variable and one or more independent variables.
The general form of first-order regression model is y-cap = β 0 + β 1 x + ε, Where, the variable y is the dependent variable that is to be modelled or predicted, the variable x is the independent variable that is used to predict the dependent variable, and ε is the error term.
Step by step
Solved in 2 steps

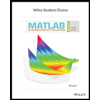
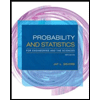
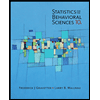
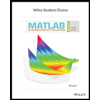
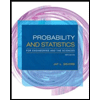
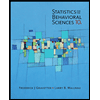
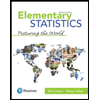
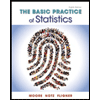
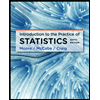