Assume you are giving a dataset that is based on user surveys. You notice that many surveys have missing fields. You also notice that some surveys have fields on a scale of 1 to 5 while others are on a scale of 1 to 10. The survey has mixed information using metric measurements and the imperial system. There are some different fields depending on the country, but they all relate to the location of the participant. You also notice that some values just don’t make sense. What analysis would you do on the data set to come up with a data cleaning plan? What are some normalization and cleaning tasks you could do based on the information provided in this description?
Assume you are giving a dataset that is based on user surveys. You notice that many surveys have missing fields. You also notice that some surveys have fields on a scale of 1 to 5 while others are on a scale of 1 to 10. The survey has mixed information using metric measurements and the imperial system. There are some different fields depending on the country, but they all relate to the location of the participant.
You also notice that some values just don’t make sense.
What analysis would you do on the data set to come up with a data cleaning plan?
What are some normalization and cleaning tasks you could do based on the information provided in this description?

Data cleaning sometimes also known as "Data Scrubbing" or "Data cleansing" is important part for data sets if one wants to create Quality data for decision-making.
Step by step
Solved in 2 steps

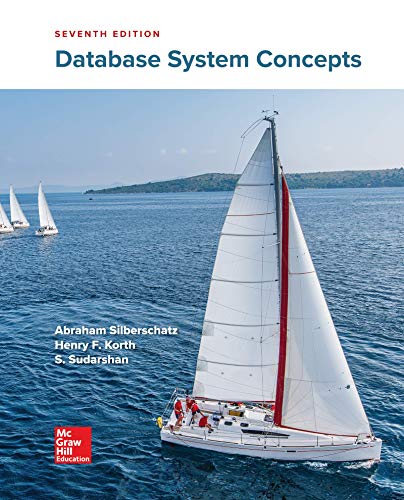
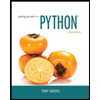
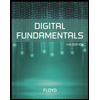
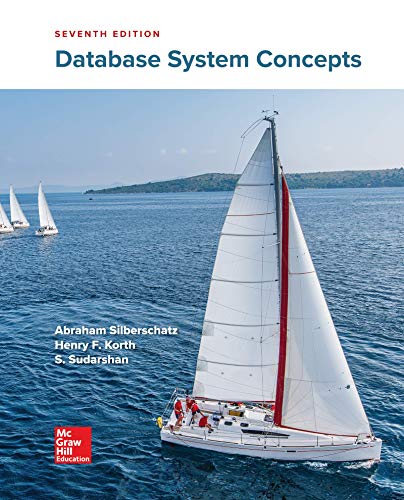
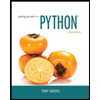
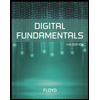
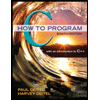
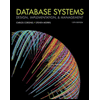
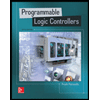