Assume that a plasma TV company is working at a 3-sigma level of quality in terms of each of100 component parts in each TV it manufactures. Because of the high price associated with theseTV sets, the company defines a product defect as any unit with one or more defective components.(That is, a good-quality output is defined as a TV set with zero defective parts.) On average, whatis the probability (rounded to 3 decimal places, e.g., 0.3331 = 0.333) of producing a unit with zerodefects. [Note: You will need to use the NORMDIST function in Excel to find the two-tailed Z valuecorresponding to the specified sigma level. This probability value is the probability associated asingle good-quality component part, under a 3-sigma performance level. Use the following formula:=(NORM.DIST(Sigma value,0,1,TRUE)*2)-1, where “sigma value” is the quality level assumed.For this exercise, you should replace “sigma value” with “3” because we are looking at a 3-sigmalevel of quality. To calculate the probability of producing a defect-free unit with n components (e.g.,n = 100), you will need to raise the calculated probability value associated with a single componentto the n-th power (e.g., if the probability for a good-quality single component is 0.90, then the probability that a given unit with 100 components would be error-free is 0.90^100 (i.e., 0.90 raised to the100th power).]
Continuous Probability Distributions
Probability distributions are of two types, which are continuous probability distributions and discrete probability distributions. A continuous probability distribution contains an infinite number of values. For example, if time is infinite: you could count from 0 to a trillion seconds, billion seconds, so on indefinitely. A discrete probability distribution consists of only a countable set of possible values.
Normal Distribution
Suppose we had to design a bathroom weighing scale, how would we decide what should be the range of the weighing machine? Would we take the highest recorded human weight in history and use that as the upper limit for our weighing scale? This may not be a great idea as the sensitivity of the scale would get reduced if the range is too large. At the same time, if we keep the upper limit too low, it may not be usable for a large percentage of the population!
Assume that a plasma TV company is working at a 3-sigma level of quality in terms of each of
100 component parts in each TV it manufactures. Because of the high price associated with these
TV sets, the company defines a product defect as any unit with one or more defective components.
(That is, a good-quality output is defined as a TV set with zero defective parts.) On average, what
is the
defects. [Note: You will need to use the NORMDIST
corresponding to the specified sigma level. This probability value is the probability associated a
single good-quality component part, under a 3-sigma performance level. Use the following formula:
=(NORM.DIST(Sigma value,0,1,TRUE)*2)-1, where “sigma value” is the quality level assumed.
For this exercise, you should replace “sigma value” with “3” because we are looking at a 3-sigma
level of quality. To calculate the probability of producing a defect-free unit with n components (e.g.,
n = 100), you will need to raise the calculated probability value associated with a single component
to the n-th power (e.g., if the probability for a good-quality single component is 0.90, then the probability that a given unit with 100 components would be error-free is 0.90^100 (i.e., 0.90 raised to the
100th power).]

Trending now
This is a popular solution!
Step by step
Solved in 2 steps with 2 images

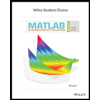
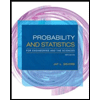
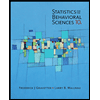
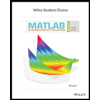
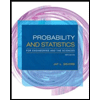
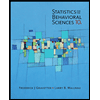
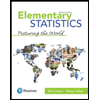
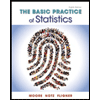
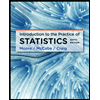