assifier has a stable learning algorithm if, by changing the tr t data don't change. For instance, the predictions of a decisi change in the training data. This definition depends on the nstable with 103 training examples may be stable with 109 nswers of unstable classifiers trained over multiple training t independent, generally sampled with replacement from the nverting weak classifier (very simple models) to strong ones s between the inputs and the class labels). A weak learner i tributes x; is only slightly correlated with its true class t₁. tter than random, but not much better than random. In b
assifier has a stable learning algorithm if, by changing the tr t data don't change. For instance, the predictions of a decisi change in the training data. This definition depends on the nstable with 103 training examples may be stable with 109 nswers of unstable classifiers trained over multiple training t independent, generally sampled with replacement from the nverting weak classifier (very simple models) to strong ones s between the inputs and the class labels). A weak learner i tributes x; is only slightly correlated with its true class t₁. tter than random, but not much better than random. In b
Database System Concepts
7th Edition
ISBN:9780078022159
Author:Abraham Silberschatz Professor, Henry F. Korth, S. Sudarshan
Publisher:Abraham Silberschatz Professor, Henry F. Korth, S. Sudarshan
Chapter1: Introduction
Section: Chapter Questions
Problem 1PE
Related questions
Question

Transcribed Image Text:Bagging is generally used to help stabilize classifiers with unstable learning algorithms (optimal score search-
ing algorithms). A classifier has a stable learning algorithm if, by changing the training data, the predicted
class labels in the test data don't change. For instance, the predictions of a decision tree might significantly
change with a small change in the training data. This definition depends on the amount of data, of course.
Classifiers that are unstable with 10³ training examples may be stable with 10⁹ examples. Bagging works
by aggregating the answers of unstable classifiers trained over multiple training datasets. These multiple
datasets are often not independent, generally sampled with replacement from the same training data.
Boosting works by converting weak classifier (very simple models) to strong ones (models that can describe
complex relationships between the inputs and the class labels). A weak learner is a classifier whose output
of an test example attributes x; is only slightly correlated with its true class tį. That is, the weak learner
classifies the data better than random, but not much better than random. In boosting, weak learners are
trained sequentially in a way that the current learner gives more emphasis to the examples that past learns
made mistakes on.
1.
Suppose we decide to use a large deep feedforward network as a classifier with a small training
dataset. Assume the network can perfectly fit the training data but we want to make sure it is accurate
in our test data (without having access to the test data). Would you use boosting or bagging to help
improve the classification accuracy? Describe what would be the problem of using the other approach.
Expert Solution

This question has been solved!
Explore an expertly crafted, step-by-step solution for a thorough understanding of key concepts.
This is a popular solution!
Trending now
This is a popular solution!
Step by step
Solved in 3 steps

Knowledge Booster
Learn more about
Need a deep-dive on the concept behind this application? Look no further. Learn more about this topic, computer-science and related others by exploring similar questions and additional content below.Recommended textbooks for you
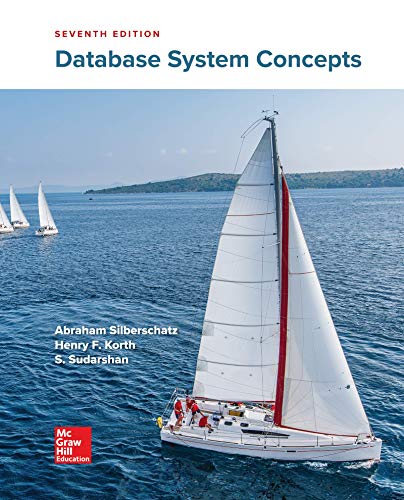
Database System Concepts
Computer Science
ISBN:
9780078022159
Author:
Abraham Silberschatz Professor, Henry F. Korth, S. Sudarshan
Publisher:
McGraw-Hill Education
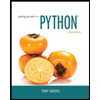
Starting Out with Python (4th Edition)
Computer Science
ISBN:
9780134444321
Author:
Tony Gaddis
Publisher:
PEARSON
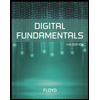
Digital Fundamentals (11th Edition)
Computer Science
ISBN:
9780132737968
Author:
Thomas L. Floyd
Publisher:
PEARSON
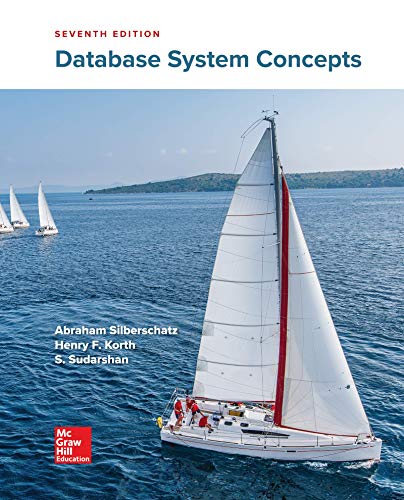
Database System Concepts
Computer Science
ISBN:
9780078022159
Author:
Abraham Silberschatz Professor, Henry F. Korth, S. Sudarshan
Publisher:
McGraw-Hill Education
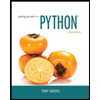
Starting Out with Python (4th Edition)
Computer Science
ISBN:
9780134444321
Author:
Tony Gaddis
Publisher:
PEARSON
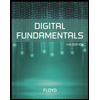
Digital Fundamentals (11th Edition)
Computer Science
ISBN:
9780132737968
Author:
Thomas L. Floyd
Publisher:
PEARSON
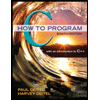
C How to Program (8th Edition)
Computer Science
ISBN:
9780133976892
Author:
Paul J. Deitel, Harvey Deitel
Publisher:
PEARSON
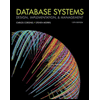
Database Systems: Design, Implementation, & Manag…
Computer Science
ISBN:
9781337627900
Author:
Carlos Coronel, Steven Morris
Publisher:
Cengage Learning
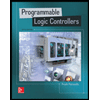
Programmable Logic Controllers
Computer Science
ISBN:
9780073373843
Author:
Frank D. Petruzella
Publisher:
McGraw-Hill Education