a. A smaller MSE means greater precision, thus, a minimum MSE estimator is preferred because it is the most precise. b. A minimum MSE estimator may not always exist. c. E(Y/D)=E(Y) d. The conditional distribution of any other statistics T=T(X) given S=s does not depend on the value of the parameter, for any given value of the statistic. e. If the ratio f(x)/g(SI) is independent of e. f. P(ACB)=P(A) P(B) g. The set of statistic (S₁, S2S may be jointly sufficient for single parameter 8. h. The random sample is jointly sufficient.
a. A smaller MSE means greater precision, thus, a minimum MSE estimator is preferred because it is the most precise. b. A minimum MSE estimator may not always exist. c. E(Y/D)=E(Y) d. The conditional distribution of any other statistics T=T(X) given S=s does not depend on the value of the parameter, for any given value of the statistic. e. If the ratio f(x)/g(SI) is independent of e. f. P(ACB)=P(A) P(B) g. The set of statistic (S₁, S2S may be jointly sufficient for single parameter 8. h. The random sample is jointly sufficient.
MATLAB: An Introduction with Applications
6th Edition
ISBN:9781119256830
Author:Amos Gilat
Publisher:Amos Gilat
Chapter1: Starting With Matlab
Section: Chapter Questions
Problem 1P
Related questions
Question
Answer 6,7,8,9 and 10. You can choose your answer in next picture and if there is no answer just right FALSE.
![Choices:
a. A smaller MSE means greater precision, thus, a minimum MSE estimator is preferred because it is
the most precise.
b. A minimum MSE estimator may not always exist.
c. E(Y/D)=E(Y)
d. The conditional distribution of any other statistics T=T(X) given S=s does not depend on the
value of the parameter, for any given value of the statistic.
e. If the ratio f(x)/g(SI) is independent of 8.
f. P(ACB)=P(A) P(B)
g. The set of statistic (S₁, S...S} may be jointly sufficient for single parameter e.
h. The random sample is jointly sufficient.
i. Fisher-Neyman Factorization Theorem
j. There may exist several sufficient statistics for but we want to choose that one which results in the
greatest data reduction.
k. A statistic T=T(X) whose distribution does not depend on the parameter 8.
I. Has no value for the estimation of when it is used in conjunction with other statistics
m. E[g(T)] =0 implies P{g(T)} =1.
n. Maximum Likelihood Estimator
o. UMVUE
p. CRLB
q. If T(X) is complete and minimal sufficient statistic, the T(X) is independent of every ancillary
statistic.
r. Getting the product of every iid distribution
s. Method of Moments Estimator
t. S=S(X) = [d(x₁)
u. Likelihood Principle
v. MSE
w. MLEs may not always exist and may not be unique, although often they are.
x. MLE may be found without using derivatives
y. Cramer Rao Lower Bound
z. Lehmann-Scheffe Theorem](/v2/_next/image?url=https%3A%2F%2Fcontent.bartleby.com%2Fqna-images%2Fquestion%2F71b6bd3c-4f64-4d2c-99e4-db0ab80b40bc%2F962f932e-c797-4a9e-a287-ea5b8868dc97%2Fms22kf8_processed.jpeg&w=3840&q=75)
Transcribed Image Text:Choices:
a. A smaller MSE means greater precision, thus, a minimum MSE estimator is preferred because it is
the most precise.
b. A minimum MSE estimator may not always exist.
c. E(Y/D)=E(Y)
d. The conditional distribution of any other statistics T=T(X) given S=s does not depend on the
value of the parameter, for any given value of the statistic.
e. If the ratio f(x)/g(SI) is independent of 8.
f. P(ACB)=P(A) P(B)
g. The set of statistic (S₁, S...S} may be jointly sufficient for single parameter e.
h. The random sample is jointly sufficient.
i. Fisher-Neyman Factorization Theorem
j. There may exist several sufficient statistics for but we want to choose that one which results in the
greatest data reduction.
k. A statistic T=T(X) whose distribution does not depend on the parameter 8.
I. Has no value for the estimation of when it is used in conjunction with other statistics
m. E[g(T)] =0 implies P{g(T)} =1.
n. Maximum Likelihood Estimator
o. UMVUE
p. CRLB
q. If T(X) is complete and minimal sufficient statistic, the T(X) is independent of every ancillary
statistic.
r. Getting the product of every iid distribution
s. Method of Moments Estimator
t. S=S(X) = [d(x₁)
u. Likelihood Principle
v. MSE
w. MLEs may not always exist and may not be unique, although often they are.
x. MLE may be found without using derivatives
y. Cramer Rao Lower Bound
z. Lehmann-Scheffe Theorem

Transcribed Image Text:1. Equivariance
2. Independent
3. Complete Sufficient Statistics
4. Ancillary Statistics
5. Basu Theorem
6. Minimal Sufficient Statistics
7. Likelihood Function
8. Uniformly Minimum Variance Unbiased Estimator
9. Estimator
10. Sufficient Statistics
Expert Solution

This question has been solved!
Explore an expertly crafted, step-by-step solution for a thorough understanding of key concepts.
Step by step
Solved in 2 steps

Recommended textbooks for you
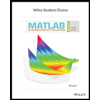
MATLAB: An Introduction with Applications
Statistics
ISBN:
9781119256830
Author:
Amos Gilat
Publisher:
John Wiley & Sons Inc
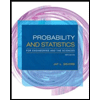
Probability and Statistics for Engineering and th…
Statistics
ISBN:
9781305251809
Author:
Jay L. Devore
Publisher:
Cengage Learning
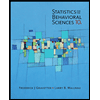
Statistics for The Behavioral Sciences (MindTap C…
Statistics
ISBN:
9781305504912
Author:
Frederick J Gravetter, Larry B. Wallnau
Publisher:
Cengage Learning
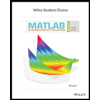
MATLAB: An Introduction with Applications
Statistics
ISBN:
9781119256830
Author:
Amos Gilat
Publisher:
John Wiley & Sons Inc
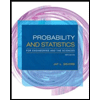
Probability and Statistics for Engineering and th…
Statistics
ISBN:
9781305251809
Author:
Jay L. Devore
Publisher:
Cengage Learning
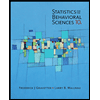
Statistics for The Behavioral Sciences (MindTap C…
Statistics
ISBN:
9781305504912
Author:
Frederick J Gravetter, Larry B. Wallnau
Publisher:
Cengage Learning
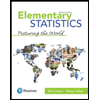
Elementary Statistics: Picturing the World (7th E…
Statistics
ISBN:
9780134683416
Author:
Ron Larson, Betsy Farber
Publisher:
PEARSON
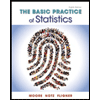
The Basic Practice of Statistics
Statistics
ISBN:
9781319042578
Author:
David S. Moore, William I. Notz, Michael A. Fligner
Publisher:
W. H. Freeman
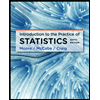
Introduction to the Practice of Statistics
Statistics
ISBN:
9781319013387
Author:
David S. Moore, George P. McCabe, Bruce A. Craig
Publisher:
W. H. Freeman