6. Calculate the p-value for this test. p = 0.475687 7. Based on the above p-value, we have extremely strong evidence against the null hypothesis.
6. Calculate the p-value for this test. p = 0.475687 7. Based on the above p-value, we have extremely strong evidence against the null hypothesis.
MATLAB: An Introduction with Applications
6th Edition
ISBN:9781119256830
Author:Amos Gilat
Publisher:Amos Gilat
Chapter1: Starting With Matlab
Section: Chapter Questions
Problem 1P
Related questions
Question
I need help with 6 and 7

Transcribed Image Text:---
### Blood Type and COVID-19 Susceptibility
**Introduction:**
A group of researchers in Wuhan, China, investigated the relationship between contracting the novel coronavirus and patients' blood type. The population in Wuhan has a blood type distribution as shown in the table below. The researchers categorized 375 patients who had contracted coronavirus by blood type.
**Population and Patient Distribution:**
| Blood Type | Population Percentage | COVID-19 Patients |
|------------|-----------------------|-------------------|
| Type A | 33% | 115 |
| Type B | 24% | 101 |
| Type AB | 9% | 41 |
| Type O | 34% | 118 |
| **Total** | **100%** | **375** |
**Instructions:**
Round all calculated values in this problem to 4 decimal places.
---
**1. Calculate Expected Values**
Calculate the expected values for the hypothesis test. Enter these values in the table below:
| Blood Type | Expected Value |
|------------|----------------|
| Type A | 123.75 |
| Type B | 90 |
| Type AB | 33.75 |
| Type O | 127.5 |
**2. Hypothesis Testing**
The researchers wonder if, in Wuhan, COVID-19 patients have a different distribution of blood type than the general population of Wuhan. Express this research question in terms of a null and alternative hypothesis.
- **Null Hypothesis (H₀):** The distribution of blood type of COVID-19 patients is **the same as** the population. Any observed difference **is** due to chance.
- **Alternative Hypothesis (H₁):** The distribution of blood type of COVID-19 patients is **different from** the population. Any observed difference **is not** due to chance.
---
To proceed with the analysis, calculate the expected frequencies assuming the null hypothesis is true, then compare them with the observed frequencies using a chi-square test.
This data is critical for understanding any significant differences between the blood type distribution of COVID-19 patients and the general population, which can help in medical research and public health decisions.
Continue to practice with similar datasets to enhance your statistical analysis skills.
---
![### Hypothesis Testing for Blood Type Distribution in COVID-19 Patients in Wuhan
**2. Null and Alternative Hypotheses**
The researchers question if the distribution of blood types in COVID-19 patients in Wuhan is different from that of the general population of Wuhan. This is expressed in terms of the following hypotheses:
- **Null Hypothesis (\(H_0\))**: The distribution of blood type of COVID-19 patients is **the same as** the population. Any observed difference is **due to** chance.
- **Alternative Hypothesis (\(H_A\))**: The distribution of blood type of COVID-19 patients is **different from** the population. Any observed difference is **not due to** chance.
**3. Analysis of Contributions to the Test Statistic**
The research examines which blood type, A or O, contributes more to the test statistic. Based on the input:
- **Blood type O** is identified as contributing more to the test statistic.
**4. Calculating the Test Statistic**
The calculated chi-squared (\(\chi^2\)) test statistic value is:
\[ \chi^2 = 4.2283 \]
**5. Degrees of Freedom**
The degrees of freedom (df) for this test is given as:
\[ d_f = 3 \]
**6. Calculating the p-Value**
The p-value corresponding to the test statistic is:
\[ p = 0.47568 \]
**7. Conclusion Based on the p-Value**
With the given p-value, we state that:
- The evidence against the null hypothesis is **extremely strong**.
In conclusion, these statistical results are useful for determining whether the observed blood type distribution in COVID-19 patients is significantly different from that of the general population in Wuhan, providing critical insights into potential associations between blood type and COVID-19 infection.](/v2/_next/image?url=https%3A%2F%2Fcontent.bartleby.com%2Fqna-images%2Fquestion%2F9896e9f0-21a4-41f5-9cfe-a9d2b9678883%2Fafe23b69-48e8-4371-9157-aece3acf149a%2Ft8mtt0f_processed.png&w=3840&q=75)
Transcribed Image Text:### Hypothesis Testing for Blood Type Distribution in COVID-19 Patients in Wuhan
**2. Null and Alternative Hypotheses**
The researchers question if the distribution of blood types in COVID-19 patients in Wuhan is different from that of the general population of Wuhan. This is expressed in terms of the following hypotheses:
- **Null Hypothesis (\(H_0\))**: The distribution of blood type of COVID-19 patients is **the same as** the population. Any observed difference is **due to** chance.
- **Alternative Hypothesis (\(H_A\))**: The distribution of blood type of COVID-19 patients is **different from** the population. Any observed difference is **not due to** chance.
**3. Analysis of Contributions to the Test Statistic**
The research examines which blood type, A or O, contributes more to the test statistic. Based on the input:
- **Blood type O** is identified as contributing more to the test statistic.
**4. Calculating the Test Statistic**
The calculated chi-squared (\(\chi^2\)) test statistic value is:
\[ \chi^2 = 4.2283 \]
**5. Degrees of Freedom**
The degrees of freedom (df) for this test is given as:
\[ d_f = 3 \]
**6. Calculating the p-Value**
The p-value corresponding to the test statistic is:
\[ p = 0.47568 \]
**7. Conclusion Based on the p-Value**
With the given p-value, we state that:
- The evidence against the null hypothesis is **extremely strong**.
In conclusion, these statistical results are useful for determining whether the observed blood type distribution in COVID-19 patients is significantly different from that of the general population in Wuhan, providing critical insights into potential associations between blood type and COVID-19 infection.
Expert Solution

This question has been solved!
Explore an expertly crafted, step-by-step solution for a thorough understanding of key concepts.
Step by step
Solved in 3 steps with 3 images

Knowledge Booster
Learn more about
Need a deep-dive on the concept behind this application? Look no further. Learn more about this topic, statistics and related others by exploring similar questions and additional content below.Recommended textbooks for you
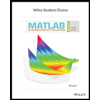
MATLAB: An Introduction with Applications
Statistics
ISBN:
9781119256830
Author:
Amos Gilat
Publisher:
John Wiley & Sons Inc
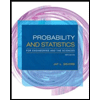
Probability and Statistics for Engineering and th…
Statistics
ISBN:
9781305251809
Author:
Jay L. Devore
Publisher:
Cengage Learning
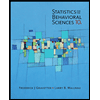
Statistics for The Behavioral Sciences (MindTap C…
Statistics
ISBN:
9781305504912
Author:
Frederick J Gravetter, Larry B. Wallnau
Publisher:
Cengage Learning
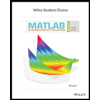
MATLAB: An Introduction with Applications
Statistics
ISBN:
9781119256830
Author:
Amos Gilat
Publisher:
John Wiley & Sons Inc
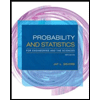
Probability and Statistics for Engineering and th…
Statistics
ISBN:
9781305251809
Author:
Jay L. Devore
Publisher:
Cengage Learning
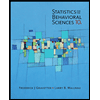
Statistics for The Behavioral Sciences (MindTap C…
Statistics
ISBN:
9781305504912
Author:
Frederick J Gravetter, Larry B. Wallnau
Publisher:
Cengage Learning
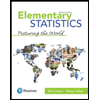
Elementary Statistics: Picturing the World (7th E…
Statistics
ISBN:
9780134683416
Author:
Ron Larson, Betsy Farber
Publisher:
PEARSON
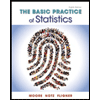
The Basic Practice of Statistics
Statistics
ISBN:
9781319042578
Author:
David S. Moore, William I. Notz, Michael A. Fligner
Publisher:
W. H. Freeman
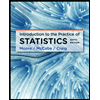
Introduction to the Practice of Statistics
Statistics
ISBN:
9781319013387
Author:
David S. Moore, George P. McCabe, Bruce A. Craig
Publisher:
W. H. Freeman