6 4 3 2 x LoserRegret auctions o NoFeedback auctions N 0 .1 2 88 3 ಪಂದ್ಯಂತ ಹಂತವನ 5 ratio A 6 A. Ratan, Y. Wen/Economics Letters 143 (2016) 114-117 7 8 9 Fig. 1. Empirical distribution of the bid/value ratio by type of auction. (Salo and Weber, 1995)-are not relevant for bidding in our design.² Our results do not suggest any significant differences based on an- ticipated regret across treatments.³ 2. The experiment We use FP auctions in which human participants bid against pre-programmed computers; this allows that objective probabili- ties of winning conditional on bids can be derived. The experiment was run at the Monash Laboratory for Experimental Economics at Monash University. Students in undergraduate and master's level courses in various disciplines participated in the experiment. Each participant was randomly assigned to a treatment (see Appendix A for instructions). 2.1. LoserRegret auctions The sequence of events in a session corresponding to LoserRe- gret auctions were as follows: thro 1. Initial instructions described the showup fee (7 AUD) and the rate at which experimental currency-ECU was converted to money (AUD). The following instructions were communicated: (a) there will be 10 rounds in a session. (b) In each round, a sessio participants would be bidding a FP auction against three uter biddere: e36 pre-programmed computer bidders: each computer opponent would submit bid by drawing randomly and independently from the set (0.75, 1.5, 2.25,.. 75), and each number had an equal chance of being drawn. Participants could be as- signed values drawn from the set of integers: {1, 2, 3,..., 100). (c) Participants could bid discrete integers up to their values and could not see the bids submitted by rival bidders at the time of bidding. (d) the highest bidder would win the auction and pay 10: 2 Our study has developed contemporaneously with Katuščák et al. (2015) in which treatment conditions similar to ours, have been studied. 3 Thus, we are equating feedback with regret as in FO. Although recent papers have attempted to generalize the regret theory (e.g. Saran and Serrano, 2014), the theory as applied to auctions (FO, EWK) does not specify the circumstances under which regret may or may not be anticipated. 4 These bids correspond to 75% of the values that could have been drawn for computer bidders from the set (1, 2, 3..... 100) in each auction using alternative procedures. Thus, in our design computer bidders submit risk-neutral Nash bids without subjects being explicitly instructed about the correspondence between computer bids and value. Thus, we are able to circumvent the "anchoring" confound which may have influenced bidding if a bidding rule which described computer bids as a fraction of their values was used. 115 a price equal to her bid. Ties would be resolved in favor of the human bidder: if the bid submitted by the human agent was among the highest bids, then he would be the winner, (e) after bids were submitted for 10 rounds, auction outcomes would be revealed such that participants would get to know their earn- ings based on auction outcomes and one of the 10 rounds would a be colect be selected randomly for final payment. 2. A short quiz was administered to evaluate participants' under- Sugar standing of the instructions and participants practiced bidding n three unpaid rounde in three unpaid rounds. rounde www 3. In the bidding rounds that had payoff consequences a set of values was created by pre-selecting 10 ECU values from the set (1, 2... 100). The set of 10 values selected was (31, 37, 43, 49, 55, 61, 67, 73, 79, 85). This set was fixed for each partici- pant. Values were assigned to participants (in each round) by drawing randomly without replacement from this set. 2.2. Treatment differences The sequence of events for NoFeedback auctions was similar to those above, except for the following design differences. The bidders were instructed that after bids have been submitted for all rounds the computer will display whether they won the auction or not, their earnings based on auction outcomes, and a. LoserRegret auctions: the highest (winning) bid for that auction. b. NoFeedback auctions: any other information regarding the bids of the other bidders will not be shown.5 Table 1 summarizes treatment differences. 3. Results A total of 40 and 38 participants were assigned to Loser Regret and NoFeedback auctions, respectively. The actual mean payoff for a participant inclusive of the participation fee was about AUD 23. The summary statistics for bids at various value draws arereported in Table 2. Treatment differences First, we explore treatment differences at various value draws. As described in Table 2, although the means of the bids are slightly larger at most value draws in LoserRegret auctions, the p values for the Wilcoxon rank-sum test and the Kolmogorov-Smirnov test for equality of of distribution of bids, sugg suggest that the hypothesis of equality of distribution of bids cannot be rejected at any value draw except for ECU value = 67 at 10% level. Second, we calculate the bid-value ratio for each bid-value pair. In Fig. 1, the cumulative distribution functions (CDFs) of the bid-value ratios for LoserRegret auctions and NoFeedback auctions are plotted. The CDF of the bid-value ratio for LoserRegret lies below the corresponding CDF for NoFeedback auctions for bid-value ratio less than 0.8; however for bid-value ratio larger than 0.8, the CDFs for these treatments tend to overlap. This figure indicates slightly more aggressive bidding in LoserRegret auctions. We further explore treatment differences by estimating the following: Yi.r = a + BR+ ni.r (1) where i = 1, 2, ..., N; r = 1, 2,..., 10, and R = 1 for LoserRegret auctions, 0 otherwise and nir are errors. The dependent variable yir equals the bid or the bid-value ratio in various specifications. If yir = bidir value-fixed effects were added. If yir = bidir (Vi.r = 5 These differences are consistent with the feedback (treatment) conditions reported in FO.
6 4 3 2 x LoserRegret auctions o NoFeedback auctions N 0 .1 2 88 3 ಪಂದ್ಯಂತ ಹಂತವನ 5 ratio A 6 A. Ratan, Y. Wen/Economics Letters 143 (2016) 114-117 7 8 9 Fig. 1. Empirical distribution of the bid/value ratio by type of auction. (Salo and Weber, 1995)-are not relevant for bidding in our design.² Our results do not suggest any significant differences based on an- ticipated regret across treatments.³ 2. The experiment We use FP auctions in which human participants bid against pre-programmed computers; this allows that objective probabili- ties of winning conditional on bids can be derived. The experiment was run at the Monash Laboratory for Experimental Economics at Monash University. Students in undergraduate and master's level courses in various disciplines participated in the experiment. Each participant was randomly assigned to a treatment (see Appendix A for instructions). 2.1. LoserRegret auctions The sequence of events in a session corresponding to LoserRe- gret auctions were as follows: thro 1. Initial instructions described the showup fee (7 AUD) and the rate at which experimental currency-ECU was converted to money (AUD). The following instructions were communicated: (a) there will be 10 rounds in a session. (b) In each round, a sessio participants would be bidding a FP auction against three uter biddere: e36 pre-programmed computer bidders: each computer opponent would submit bid by drawing randomly and independently from the set (0.75, 1.5, 2.25,.. 75), and each number had an equal chance of being drawn. Participants could be as- signed values drawn from the set of integers: {1, 2, 3,..., 100). (c) Participants could bid discrete integers up to their values and could not see the bids submitted by rival bidders at the time of bidding. (d) the highest bidder would win the auction and pay 10: 2 Our study has developed contemporaneously with Katuščák et al. (2015) in which treatment conditions similar to ours, have been studied. 3 Thus, we are equating feedback with regret as in FO. Although recent papers have attempted to generalize the regret theory (e.g. Saran and Serrano, 2014), the theory as applied to auctions (FO, EWK) does not specify the circumstances under which regret may or may not be anticipated. 4 These bids correspond to 75% of the values that could have been drawn for computer bidders from the set (1, 2, 3..... 100) in each auction using alternative procedures. Thus, in our design computer bidders submit risk-neutral Nash bids without subjects being explicitly instructed about the correspondence between computer bids and value. Thus, we are able to circumvent the "anchoring" confound which may have influenced bidding if a bidding rule which described computer bids as a fraction of their values was used. 115 a price equal to her bid. Ties would be resolved in favor of the human bidder: if the bid submitted by the human agent was among the highest bids, then he would be the winner, (e) after bids were submitted for 10 rounds, auction outcomes would be revealed such that participants would get to know their earn- ings based on auction outcomes and one of the 10 rounds would a be colect be selected randomly for final payment. 2. A short quiz was administered to evaluate participants' under- Sugar standing of the instructions and participants practiced bidding n three unpaid rounde in three unpaid rounds. rounde www 3. In the bidding rounds that had payoff consequences a set of values was created by pre-selecting 10 ECU values from the set (1, 2... 100). The set of 10 values selected was (31, 37, 43, 49, 55, 61, 67, 73, 79, 85). This set was fixed for each partici- pant. Values were assigned to participants (in each round) by drawing randomly without replacement from this set. 2.2. Treatment differences The sequence of events for NoFeedback auctions was similar to those above, except for the following design differences. The bidders were instructed that after bids have been submitted for all rounds the computer will display whether they won the auction or not, their earnings based on auction outcomes, and a. LoserRegret auctions: the highest (winning) bid for that auction. b. NoFeedback auctions: any other information regarding the bids of the other bidders will not be shown.5 Table 1 summarizes treatment differences. 3. Results A total of 40 and 38 participants were assigned to Loser Regret and NoFeedback auctions, respectively. The actual mean payoff for a participant inclusive of the participation fee was about AUD 23. The summary statistics for bids at various value draws arereported in Table 2. Treatment differences First, we explore treatment differences at various value draws. As described in Table 2, although the means of the bids are slightly larger at most value draws in LoserRegret auctions, the p values for the Wilcoxon rank-sum test and the Kolmogorov-Smirnov test for equality of of distribution of bids, sugg suggest that the hypothesis of equality of distribution of bids cannot be rejected at any value draw except for ECU value = 67 at 10% level. Second, we calculate the bid-value ratio for each bid-value pair. In Fig. 1, the cumulative distribution functions (CDFs) of the bid-value ratios for LoserRegret auctions and NoFeedback auctions are plotted. The CDF of the bid-value ratio for LoserRegret lies below the corresponding CDF for NoFeedback auctions for bid-value ratio less than 0.8; however for bid-value ratio larger than 0.8, the CDFs for these treatments tend to overlap. This figure indicates slightly more aggressive bidding in LoserRegret auctions. We further explore treatment differences by estimating the following: Yi.r = a + BR+ ni.r (1) where i = 1, 2, ..., N; r = 1, 2,..., 10, and R = 1 for LoserRegret auctions, 0 otherwise and nir are errors. The dependent variable yir equals the bid or the bid-value ratio in various specifications. If yir = bidir value-fixed effects were added. If yir = bidir (Vi.r = 5 These differences are consistent with the feedback (treatment) conditions reported in FO.
Chapter1: Making Economics Decisions
Section: Chapter Questions
Problem 1QTC
Related questions
Question
Please briefly explain the results with explanation and clear points.
Question - List the main results ?

Transcribed Image Text:វ៉
Co
(0
5
3
2
O
x LoserRegret auctions
o NoFeedback auctions
IM
0
.1
000
2
BO
3
4
.5
ratio
.6
A. Ratan, Y. Wen/Economics Letters 143 (2016) 114-117
.7
8 .9
1
Fig. 1. Empirical distribution of the bid/value ratio by type of auction.
(Salo and Weber, 1995)-are not relevant for bidding in our design.²
Our results do not suggest any significant differences based on an-
ticipated regret across treatments.³
2. The experiment
We use FP auctions in which human participants bid against
pre-programmed computers; this allows that objective probabili-
ties of winning conditional on bids can be derived. The experiment
was run at the Monash Laboratory for Experimental Economics at
Monash University. Students in undergraduate and master's level
courses in various disciplines participated in the experiment. Each
participant was randomly assigned to a treatment (see Appendix A
for instructions).
2.1. LoserRegret auctions
The sequence of events in a session corresponding to LoserRe-
gret auctions were as follows:
1. Initial instructions described the showup fee (7 AUD) and the
rate at which experimental currency-ECU was converted to
money (AUD). The following instructions were communicated:
(a) there will be 10 rounds in a session. (b) In each round,
participants would be bidding in a FP auction against three
pre-programmed computer bidders: each computer opponent
would submit a bid by drawing randomly and independently
from the set (0.75, 1.5, 2.25,...,75), and each number had
an equal chance of being drawn. Participants could be as-
signed values drawn from the set of integers: {1, 2, 3, ..., 100).
(c) Participants could bid discrete integers up to their values and
could not see the bids submitted by rival bidders at the time of
bidding, (d) the highest bidder would win the auction and pay
2 Our study has developed contemporaneously with Katuščák et al. (2015) in
which treatment conditions similar to ours, have been studied.
3 Thus, we are equating feedback with regret as in FO. Although recent papers
have attempted to generalize the regret theory (e.g. Saran and Serrano, 2014), the
theory as applied to auctions (FO, EWK) does not specify the circumstances under
which regret may or may not be anticipated.
4 These bids correspond to 75% of the values that could have been drawn for
computer bidders from the set (1, 2, 3, ..., 100) in each auction using alternative
procedures. Thus, in our design computer bidders submit risk-neutral Nash bids
without subjects being explicitly instructed about the correspondence between
computer bids and value. Thus, we are able to circumvent the "anchoring" confound
which may have influenced bidding if a bidding rule which described computer bids
as a fraction of their values was used.
115
a price equal to her bid. Ties would be resolved in favor of the
human bidder: if the bid submitted by the human agent was
among the highest bids, then he would be the winner, (e) after
bids were submitted for 10 rounds, auction outcomes would be
revealed such that participants would get to know their earn-
ings based on auction outcomes and one of the 10 rounds would
be selected randomly for final payment.
2. A short quiz was administered to evaluate participants' under-
standing of the instructions and participants practiced bidding
in three unpaid rounds.
3. In the bidding rounds that had payoff consequences a set of
values was created by pre-selecting 10 ECU values from the
set (1, 2... 100). The set of 10 values selected was (31, 37, 43,
49, 55, 61, 67, 73, 79, 85). This set was fixed for each partici-
pant. Values were assigned to participants (in each round) by
drawing randomly without replacement from this set.
2.2. Treatment differences
The sequence of events for NoFeedback auctions was similar
to those above, except for the following design differences. The
bidders were instructed that after bids have been submitted for all
rounds the computer will display whether they won the auction or
not, their earnings based on auction outcomes, and
a. LoserRegret auctions: the highest (winning) bid for that auction.
b. NoFeedback auctions: any other information regarding the bids
of the other bidders will not be shown.5
Table 1 summarizes treatment differences.
3. Results
A total of 40 and 38 participants were assigned to LoserRegret
and NoFeedback auctions, respectively. The actual mean payoff for
a participant inclusive of the participation fee was about AUD 23.
The summary statistics for bids at various value draws arereported
in Table 2.
Treatment differences
First, we explore treatment differences at various value draws.
As described in Table 2, although the means of the bids are slightly
larger at most value draws in LoserRegret auctions, the p values
for the Wilcoxon rank-sum test and the Kolmogorov-Smirnov test
for equality of distribution of bids, suggest that the hypothesis of
equality of distribution of bids cannot be rejected at any value draw
except for ECU value = 67 at 10% level. Second, we calculate the
bid-value ratio for each bid-value pair. In Fig. 1, the cumulative
distribution functions (CDFs) of the bid-value ratios for LoserRegret
the vide
Co
auctions and NoFeedback auctions are plotted. The CDF of the
bid-value ratio for LoserRegret lies below the corresponding CDF
for NoFeedback auctions for bid-value ratio less than 0.8; however
for bid-value ratio larger than 0.8, the CDFs for these treatments
tend to overlap. This figure indicates slightly more aggressive
bidding in LoserRegret auctions.
We further explore treatment differences by estimating the
following:
yir = a + BR+ ni.r
(1)
where i = 1, 2, ..., N; r = 1, 2,..., 10, and R = 1 for LoserRegret
auctions, 0 otherwise and nir are errors. The dependent variable
yir equals the bid or the bid-value ratio in various specifications.
If yir = bidi,r value-fixed effects were added. If yir= bidir (Vi.r =
5 These differences are consistent with the feedback (treatment) conditions
reported in FO.
Expert Solution

This question has been solved!
Explore an expertly crafted, step-by-step solution for a thorough understanding of key concepts.
Step by step
Solved in 4 steps

Knowledge Booster
Learn more about
Need a deep-dive on the concept behind this application? Look no further. Learn more about this topic, economics and related others by exploring similar questions and additional content below.Recommended textbooks for you
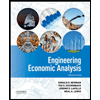
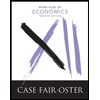
Principles of Economics (12th Edition)
Economics
ISBN:
9780134078779
Author:
Karl E. Case, Ray C. Fair, Sharon E. Oster
Publisher:
PEARSON
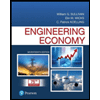
Engineering Economy (17th Edition)
Economics
ISBN:
9780134870069
Author:
William G. Sullivan, Elin M. Wicks, C. Patrick Koelling
Publisher:
PEARSON
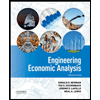
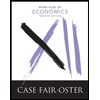
Principles of Economics (12th Edition)
Economics
ISBN:
9780134078779
Author:
Karl E. Case, Ray C. Fair, Sharon E. Oster
Publisher:
PEARSON
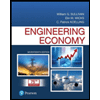
Engineering Economy (17th Edition)
Economics
ISBN:
9780134870069
Author:
William G. Sullivan, Elin M. Wicks, C. Patrick Koelling
Publisher:
PEARSON
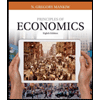
Principles of Economics (MindTap Course List)
Economics
ISBN:
9781305585126
Author:
N. Gregory Mankiw
Publisher:
Cengage Learning
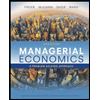
Managerial Economics: A Problem Solving Approach
Economics
ISBN:
9781337106665
Author:
Luke M. Froeb, Brian T. McCann, Michael R. Ward, Mike Shor
Publisher:
Cengage Learning
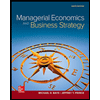
Managerial Economics & Business Strategy (Mcgraw-…
Economics
ISBN:
9781259290619
Author:
Michael Baye, Jeff Prince
Publisher:
McGraw-Hill Education