4. Gradient descent. Gradient descent is one of the most popular algorithms in data science and by far the most common way to optimise neural networks. A function is minimised by iteratively moving a little bit in the direction of negative gradient. For the two-dimensional case, the step of iteration is given by the formula (**+²) = (*) – - € ▼ ƒ (xXn, Yn). Yn+1, In general, & does not have to be a constant, but in this question, for demonstrative purposes, we set = 0.1. Let f(x, y) = 3.5x² - 4xy +6.5y² and ro and yo be any real numbers. (a) For all x, y R compute Vf(x, y) and find a matrix A such that (-) = (-) - in terms of xo and yo and powers of A. A Write an expression for (5) Yn - ε V f (x, y). (b) Find the eigenvalues of A. (c) Find one eigenvector corresponding to each eigenvalue.
4. Gradient descent. Gradient descent is one of the most popular algorithms in data science and by far the most common way to optimise neural networks. A function is minimised by iteratively moving a little bit in the direction of negative gradient. For the two-dimensional case, the step of iteration is given by the formula (**+²) = (*) – - € ▼ ƒ (xXn, Yn). Yn+1, In general, & does not have to be a constant, but in this question, for demonstrative purposes, we set = 0.1. Let f(x, y) = 3.5x² - 4xy +6.5y² and ro and yo be any real numbers. (a) For all x, y R compute Vf(x, y) and find a matrix A such that (-) = (-) - in terms of xo and yo and powers of A. A Write an expression for (5) Yn - ε V f (x, y). (b) Find the eigenvalues of A. (c) Find one eigenvector corresponding to each eigenvalue.
Advanced Engineering Mathematics
10th Edition
ISBN:9780470458365
Author:Erwin Kreyszig
Publisher:Erwin Kreyszig
Chapter2: Second-order Linear Odes
Section: Chapter Questions
Problem 1RQ
Related questions
Question

Transcribed Image Text:4. Gradient descent.
Gradient descent is one of the most popular algorithms in data science and by far the most
common way to optimise neural networks. A function is minimised by iteratively moving
a little bit in the direction of negative gradient. For the two-dimensional case, the step of
iteration is given by the formula
Xn+1
Yn+1
n+¹) = (xn) - € V ƒ (Xxnx Y₁).
E
Yn
In general, & does not have to be a constant, but in this question, for demonstrative purposes,
we set = 0.1. Let f(x, y) = 3.5x² - 4xy +6.5y² and xo and yo be any real numbers.
(a) For all x, y ER compute Vf(x, y) and find a matrix A such that
Τη
Yn
A
¹ (*) = (*)
in terms of xo and yo and powers of A.
- ε ▼ f(x, y).
Write an expression for
(b) Find the eigenvalues of A.
(c) Find one eigenvector corresponding to each eigenvalue.
(d) Find matrices P and D such that D is diagonal and A = PDP-¹.
(e) Find matrices Dn, P-1 and An. Find formulas for an and yn.
(f) Suppose to = yo = 1. Find the smallest N EN such that |(
YN
< 0.05,
)
IN-1
YN-1,
N
(g) Sketch the region R consisting of those (ro, yo) such that N ≥ 0, YN ≥ 0 and
|(x)| ≤
> 0.05,
where N is the number found in part (f). Write an equation for the boundary of R.
Which points of the boundary belongs to R and which do not?
≤0.05.
Expert Solution

This question has been solved!
Explore an expertly crafted, step-by-step solution for a thorough understanding of key concepts.
This is a popular solution!
Trending now
This is a popular solution!
Step by step
Solved in 5 steps with 13 images

Recommended textbooks for you
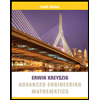
Advanced Engineering Mathematics
Advanced Math
ISBN:
9780470458365
Author:
Erwin Kreyszig
Publisher:
Wiley, John & Sons, Incorporated
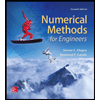
Numerical Methods for Engineers
Advanced Math
ISBN:
9780073397924
Author:
Steven C. Chapra Dr., Raymond P. Canale
Publisher:
McGraw-Hill Education
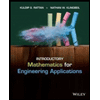
Introductory Mathematics for Engineering Applicat…
Advanced Math
ISBN:
9781118141809
Author:
Nathan Klingbeil
Publisher:
WILEY
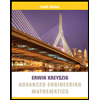
Advanced Engineering Mathematics
Advanced Math
ISBN:
9780470458365
Author:
Erwin Kreyszig
Publisher:
Wiley, John & Sons, Incorporated
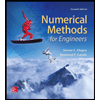
Numerical Methods for Engineers
Advanced Math
ISBN:
9780073397924
Author:
Steven C. Chapra Dr., Raymond P. Canale
Publisher:
McGraw-Hill Education
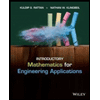
Introductory Mathematics for Engineering Applicat…
Advanced Math
ISBN:
9781118141809
Author:
Nathan Klingbeil
Publisher:
WILEY
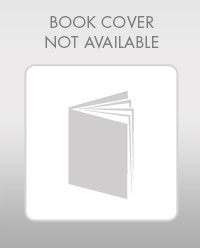
Mathematics For Machine Technology
Advanced Math
ISBN:
9781337798310
Author:
Peterson, John.
Publisher:
Cengage Learning,
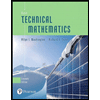
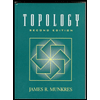