3. Fit a logistic regression model using these variables. Use DRINK as the dependent variable and CASES and SEX as independent variables. Also include as an independent variable the appropriate interaction term. Some variable creation commands Create 0/1 indicators of drinker and female gender • generate drink01=. (294 missing values generated) replace drink01=1 if drink==1 (234 real changes made) • replace drink01=0 if drink--2 (60 real changes made) • label define drinkf 0 "0=nondrinker" 1 "1=drinker" • label values drink01 drinkf • generate female-. (294 missing values generated) • replace female=0 if sex==1 (111 real changes made) • replace female=1 if sex==2 (183 real changes made) . label define sexf o "0=male" 1 "1=female" . label values female sexf .* Create a new variable called FEM_CASE that is the interaction of FEMALE and CASES • generate fem_case=female*cases * Use the command LOGISTIC if you want output to include ODDS RATIOS Use the command LOGIT if you want the output to include BETAS and SEs * LOGISTIC OUTCOME PREDICTOR PREDICTOR etc.. logit drink01 cases female fem_case
3. Fit a logistic regression model using these variables. Use DRINK as the dependent variable and CASES and SEX as independent variables. Also include as an independent variable the appropriate interaction term. Some variable creation commands Create 0/1 indicators of drinker and female gender • generate drink01=. (294 missing values generated) replace drink01=1 if drink==1 (234 real changes made) • replace drink01=0 if drink--2 (60 real changes made) • label define drinkf 0 "0=nondrinker" 1 "1=drinker" • label values drink01 drinkf • generate female-. (294 missing values generated) • replace female=0 if sex==1 (111 real changes made) • replace female=1 if sex==2 (183 real changes made) . label define sexf o "0=male" 1 "1=female" . label values female sexf .* Create a new variable called FEM_CASE that is the interaction of FEMALE and CASES • generate fem_case=female*cases * Use the command LOGISTIC if you want output to include ODDS RATIOS Use the command LOGIT if you want the output to include BETAS and SEs * LOGISTIC OUTCOME PREDICTOR PREDICTOR etc.. logit drink01 cases female fem_case
Computer Networking: A Top-Down Approach (7th Edition)
7th Edition
ISBN:9780133594140
Author:James Kurose, Keith Ross
Publisher:James Kurose, Keith Ross
Chapter1: Computer Networks And The Internet
Section: Chapter Questions
Problem R1RQ: What is the difference between a host and an end system? List several different types of end...
Related questions
Question
![3. Fit a logistic regression model using these variables. Use DRINK as the dependent variable and CASES
and SEX as independent variables. Also include as an independent variable the appropriate interaction
term.
Some variable creation commands
.* Create 0/1 indicators of drinker and female gender
• generate drink01-.
(294 missing values generated)
. replace drink01=1 if drink=1
(234 real changes made)
. replace drink01=0 if drink==2
(60 real changes made)
• label define drinkf o "0=nondrinker" 1 "1-drinker"
label values drink0l drinkf
• generate female-.
(294
values generated)
• replace female=0 if sex==1
(111 real changes made)
• replace female=1 if sex--2
(183 real changes made)
. label define sexf o "0=male" 1 "1=female"
. label values female sexf
.*Create a new variable called FEM CASE that is the interaction of FEMALE and CASES
• generate fem_case=female*cases
* Use the command LOGISTIC if you want output to include ODDS RATIOS
* Use the command LOGIT if you want the output to include BETAS and SEs
* LOGISTIC OUTCOME PREDICTOR PREDICTOR etc..
logit drink01 cases female fem_case
Logistic regression
Number of obs
294
LR chi2 (3)
5.62
Prob > chi2
0.1318
Log likelihood = -145.95772
Pseudo R2
0.0189
drink01 |
Coef.
Std. Err.
P>|z|
[95% Conf. Interval]
cases |
-.4405564
.8413815
-0.52
0.601
-2.089634
1.208521
female |
fem caseI
cons |
-.7743296
.3455196
-2.24
0.025
-1.451536
-.0971237
-.9387877
1.262453
.9386327
.9578851
0.98
0.327
2.816053
1.826851
.2879632
6.34
0.000
2.391248](/v2/_next/image?url=https%3A%2F%2Fcontent.bartleby.com%2Fqna-images%2Fquestion%2Ff77451d0-d39d-4736-b66b-3ced0a5a2bf4%2Fff2de8df-f561-45e8-af02-bec20f424a79%2Fc4fh89h_processed.jpeg&w=3840&q=75)
Transcribed Image Text:3. Fit a logistic regression model using these variables. Use DRINK as the dependent variable and CASES
and SEX as independent variables. Also include as an independent variable the appropriate interaction
term.
Some variable creation commands
.* Create 0/1 indicators of drinker and female gender
• generate drink01-.
(294 missing values generated)
. replace drink01=1 if drink=1
(234 real changes made)
. replace drink01=0 if drink==2
(60 real changes made)
• label define drinkf o "0=nondrinker" 1 "1-drinker"
label values drink0l drinkf
• generate female-.
(294
values generated)
• replace female=0 if sex==1
(111 real changes made)
• replace female=1 if sex--2
(183 real changes made)
. label define sexf o "0=male" 1 "1=female"
. label values female sexf
.*Create a new variable called FEM CASE that is the interaction of FEMALE and CASES
• generate fem_case=female*cases
* Use the command LOGISTIC if you want output to include ODDS RATIOS
* Use the command LOGIT if you want the output to include BETAS and SEs
* LOGISTIC OUTCOME PREDICTOR PREDICTOR etc..
logit drink01 cases female fem_case
Logistic regression
Number of obs
294
LR chi2 (3)
5.62
Prob > chi2
0.1318
Log likelihood = -145.95772
Pseudo R2
0.0189
drink01 |
Coef.
Std. Err.
P>|z|
[95% Conf. Interval]
cases |
-.4405564
.8413815
-0.52
0.601
-2.089634
1.208521
female |
fem caseI
cons |
-.7743296
.3455196
-2.24
0.025
-1.451536
-.0971237
-.9387877
1.262453
.9386327
.9578851
0.98
0.327
2.816053
1.826851
.2879632
6.34
0.000
2.391248
Expert Solution

This question has been solved!
Explore an expertly crafted, step-by-step solution for a thorough understanding of key concepts.
Step by step
Solved in 2 steps with 1 images

Recommended textbooks for you
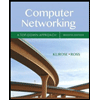
Computer Networking: A Top-Down Approach (7th Edi…
Computer Engineering
ISBN:
9780133594140
Author:
James Kurose, Keith Ross
Publisher:
PEARSON
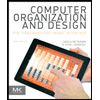
Computer Organization and Design MIPS Edition, Fi…
Computer Engineering
ISBN:
9780124077263
Author:
David A. Patterson, John L. Hennessy
Publisher:
Elsevier Science
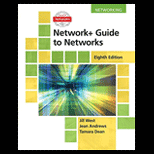
Network+ Guide to Networks (MindTap Course List)
Computer Engineering
ISBN:
9781337569330
Author:
Jill West, Tamara Dean, Jean Andrews
Publisher:
Cengage Learning
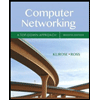
Computer Networking: A Top-Down Approach (7th Edi…
Computer Engineering
ISBN:
9780133594140
Author:
James Kurose, Keith Ross
Publisher:
PEARSON
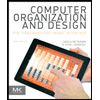
Computer Organization and Design MIPS Edition, Fi…
Computer Engineering
ISBN:
9780124077263
Author:
David A. Patterson, John L. Hennessy
Publisher:
Elsevier Science
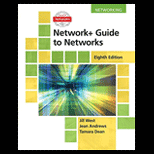
Network+ Guide to Networks (MindTap Course List)
Computer Engineering
ISBN:
9781337569330
Author:
Jill West, Tamara Dean, Jean Andrews
Publisher:
Cengage Learning
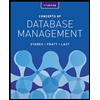
Concepts of Database Management
Computer Engineering
ISBN:
9781337093422
Author:
Joy L. Starks, Philip J. Pratt, Mary Z. Last
Publisher:
Cengage Learning
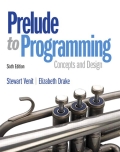
Prelude to Programming
Computer Engineering
ISBN:
9780133750423
Author:
VENIT, Stewart
Publisher:
Pearson Education
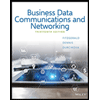
Sc Business Data Communications and Networking, T…
Computer Engineering
ISBN:
9781119368830
Author:
FITZGERALD
Publisher:
WILEY