2g) Decision Tree Classification Define a function train_DT() which takes as input x and Y and return s a Decision Tree model trained on the given X , Y . Inputs: • X: np.ndarray, training samples (predictors), • Y: np.ndarray, training labels (outcomes), Output: a trained classifier clf Hint: There are 2 steps involved in this function: Initializing an Decision Tree classifier: clf = DecisionTreeClassifier(...) • Training the classifier: clf.fit(X, Y) I # YOUR CODE HERE raise NotImplementedError(). I assert callable(train_DT)
2g) Decision Tree Classification Define a function train_DT() which takes as input x and Y and return s a Decision Tree model trained on the given X , Y . Inputs: • X: np.ndarray, training samples (predictors), • Y: np.ndarray, training labels (outcomes), Output: a trained classifier clf Hint: There are 2 steps involved in this function: Initializing an Decision Tree classifier: clf = DecisionTreeClassifier(...) • Training the classifier: clf.fit(X, Y) I # YOUR CODE HERE raise NotImplementedError(). I assert callable(train_DT)
Database System Concepts
7th Edition
ISBN:9780078022159
Author:Abraham Silberschatz Professor, Henry F. Korth, S. Sudarshan
Publisher:Abraham Silberschatz Professor, Henry F. Korth, S. Sudarshan
Chapter1: Introduction
Section: Chapter Questions
Problem 1PE
Related questions
Question
100%
Use Python
![2g) Decision Tree Classification
Define a function train_DT() which takes as input x and Y and return s a Decision Tree model trained on the given
X , Y .
Inputs:
X: np.ndarray, training samples (predictors),
Y: np.ndarray, training labels (outcomes),
Output: a trained classifier clf
Hint: There are 2 steps involved in this function:
•Initializing an Decision Tree classifier: clf =
Training the classifier: clf.fit(X, Y)
DecisionTreeClassifier(...)
I # YOUR CODE HERE
raise NotImplementedError()
I assert callable(train_DT)
2h) Classification #1: Using Only Subject
Let's try to classify the email conversation using only the subject field of the dataframe only.
Using the function train_DT() , train a decision tree classifier on subject_train_X (as your predictor) and
category_train_Y (as your outcome) and save the model as subject_clf.
I # YOUR CODE HERE
raise NotImplementedError()
I assert isinstance(subject_clf, DecisionTreeClassifier)
assert hasattr(subject_clf, "predict")
Now we will use the function classification_report to print out the performance of the classifier on the training set:
I # Your classifier should observe an accuracy of around 96%.
subject_predicted_train_Y
print(classification_report(category_train_Y, subject_predicted_train_Y))
subject_clf.predict(subject_train_X)
And now, let's check the performance of the trained classifier on the test set:
I # You should observe an accuracy of around 79%.
subject_predicted_test_Y
print(classification_report(category_test_Y, subject_predicted_test_Y))
subject_clf.predict(subject_test_X)
I assert subject_predicted_train_Y.shape
assert subject_predicted_test_Y.shape ==
(14984,)
(3746,)
==
precision, recall,
assert np.isclose(precision[0], 0.99, 0.05)
= precision_recall_fscore_support(category_train_Y, subject_predicted_train_](/v2/_next/image?url=https%3A%2F%2Fcontent.bartleby.com%2Fqna-images%2Fquestion%2F3200e892-7b83-4670-8aa5-c4c84f2a6adb%2Fe035c677-4204-434b-84aa-0a38921d3ba6%2F2gnimnr_processed.png&w=3840&q=75)
Transcribed Image Text:2g) Decision Tree Classification
Define a function train_DT() which takes as input x and Y and return s a Decision Tree model trained on the given
X , Y .
Inputs:
X: np.ndarray, training samples (predictors),
Y: np.ndarray, training labels (outcomes),
Output: a trained classifier clf
Hint: There are 2 steps involved in this function:
•Initializing an Decision Tree classifier: clf =
Training the classifier: clf.fit(X, Y)
DecisionTreeClassifier(...)
I # YOUR CODE HERE
raise NotImplementedError()
I assert callable(train_DT)
2h) Classification #1: Using Only Subject
Let's try to classify the email conversation using only the subject field of the dataframe only.
Using the function train_DT() , train a decision tree classifier on subject_train_X (as your predictor) and
category_train_Y (as your outcome) and save the model as subject_clf.
I # YOUR CODE HERE
raise NotImplementedError()
I assert isinstance(subject_clf, DecisionTreeClassifier)
assert hasattr(subject_clf, "predict")
Now we will use the function classification_report to print out the performance of the classifier on the training set:
I # Your classifier should observe an accuracy of around 96%.
subject_predicted_train_Y
print(classification_report(category_train_Y, subject_predicted_train_Y))
subject_clf.predict(subject_train_X)
And now, let's check the performance of the trained classifier on the test set:
I # You should observe an accuracy of around 79%.
subject_predicted_test_Y
print(classification_report(category_test_Y, subject_predicted_test_Y))
subject_clf.predict(subject_test_X)
I assert subject_predicted_train_Y.shape
assert subject_predicted_test_Y.shape ==
(14984,)
(3746,)
==
precision, recall,
assert np.isclose(precision[0], 0.99, 0.05)
= precision_recall_fscore_support(category_train_Y, subject_predicted_train_
Expert Solution

This question has been solved!
Explore an expertly crafted, step-by-step solution for a thorough understanding of key concepts.
This is a popular solution!
Trending now
This is a popular solution!
Step by step
Solved in 2 steps

Knowledge Booster
Learn more about
Need a deep-dive on the concept behind this application? Look no further. Learn more about this topic, computer-science and related others by exploring similar questions and additional content below.Recommended textbooks for you
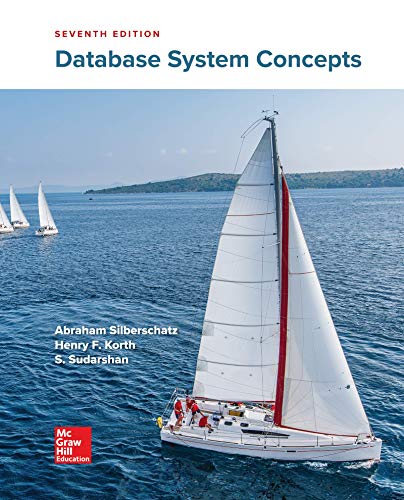
Database System Concepts
Computer Science
ISBN:
9780078022159
Author:
Abraham Silberschatz Professor, Henry F. Korth, S. Sudarshan
Publisher:
McGraw-Hill Education
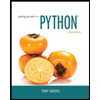
Starting Out with Python (4th Edition)
Computer Science
ISBN:
9780134444321
Author:
Tony Gaddis
Publisher:
PEARSON
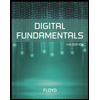
Digital Fundamentals (11th Edition)
Computer Science
ISBN:
9780132737968
Author:
Thomas L. Floyd
Publisher:
PEARSON
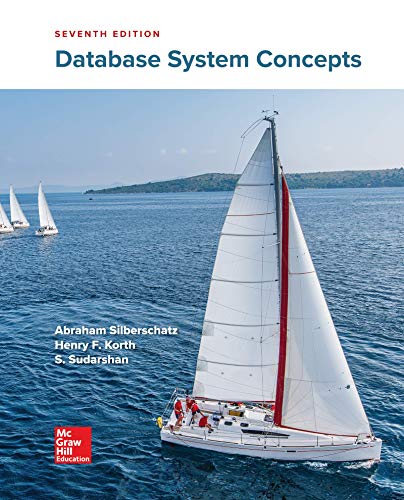
Database System Concepts
Computer Science
ISBN:
9780078022159
Author:
Abraham Silberschatz Professor, Henry F. Korth, S. Sudarshan
Publisher:
McGraw-Hill Education
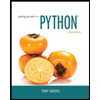
Starting Out with Python (4th Edition)
Computer Science
ISBN:
9780134444321
Author:
Tony Gaddis
Publisher:
PEARSON
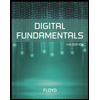
Digital Fundamentals (11th Edition)
Computer Science
ISBN:
9780132737968
Author:
Thomas L. Floyd
Publisher:
PEARSON
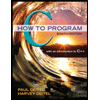
C How to Program (8th Edition)
Computer Science
ISBN:
9780133976892
Author:
Paul J. Deitel, Harvey Deitel
Publisher:
PEARSON
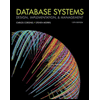
Database Systems: Design, Implementation, & Manag…
Computer Science
ISBN:
9781337627900
Author:
Carlos Coronel, Steven Morris
Publisher:
Cengage Learning
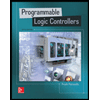
Programmable Logic Controllers
Computer Science
ISBN:
9780073373843
Author:
Frank D. Petruzella
Publisher:
McGraw-Hill Education