2.) Use the following data to plot a first order and second order model. I have provided code to generate a second order model, repeat this for a first order model, plot both models and the data on a single plot. Comment on which is the better fit based on the plot. X y 5 15 10 22 15 30 20 31 The following code produces a second-order model, enter this code and then repeat this for a first order model with the appropriate changes made, comment on what each line is doing.
2.) Use the following data to plot a first order and second order model. I have provided code to generate a second order model, repeat this for a first order model, plot both models and the data on a single plot. Comment on which is the better fit based on the plot. X y 5 15 10 22 15 30 20 31 The following code produces a second-order model, enter this code and then repeat this for a first order model with the appropriate changes made, comment on what each line is doing.
Database System Concepts
7th Edition
ISBN:9780078022159
Author:Abraham Silberschatz Professor, Henry F. Korth, S. Sudarshan
Publisher:Abraham Silberschatz Professor, Henry F. Korth, S. Sudarshan
Chapter1: Introduction
Section: Chapter Questions
Problem 1PE
Related questions
Question
Code in python please

Transcribed Image Text:**Exercise 2: Modeling with Data**
**Objective:**
Use the data provided to plot both a first order (linear) and a second order (quadratic) model. Compare the two models and the data on a single plot and determine which model fits the data better.
**Data Table:**
| x | 5 | 10 | 15 | 20 |
|----|----|----|----|----|
| y | 15 | 22 | 30 | 31 |
**Instructions:**
1. **Plot the Models:**
- Use the data to create a plot of the first order model.
- Use the data to create a plot of the second order model.
- Overlay both models on a single graph to compare them with the actual data points.
2. **Model Code:**
- Begin with the code provided to generate a second order model.
- Adjust the code for a first order model.
- Analyze and comment on the function of each line of code.
By visualizing and comparing the outputs, you can better understand which model provides a more accurate representation of the data.
![### Polynomial Regression: Quadratic Equation Model
#### Enter the Data
```python
x = np.array([5, 10, 15, 20])
y = np.array([15, 22, 30, 31])
```
#### Quadratic Equation Model (2nd Order Polynomial)
In this step, we fit a quadratic polynomial (2nd order) to the data.
Equation: \( y = b0 + b1 \times x + b2 \times x^2 \)
```python
b = np.polyfit(x, y, 2)
print("2nd order polynomial parameters:\nb2 =", b[0], "\nb1 =", b[1], "\nb0 =", b[2])
```
#### Plot the Model
We create a smooth line representing the polynomial model.
```python
x1 = np.linspace(5, 20, 100)
pylab.plot(x1, np.polyval(b, x1), c = "r", label = "2nd Order Model")
```
#### Plot the Data
Visualize the original data points and the fitted polynomial model.
```python
pylab.scatter(x, y, c = 'k')
pylab.grid()
pylab.xlabel("x")
pylab.ylabel("y")
pylab.legend()
```
### Explanation of Code
- **Data Entry**: Arrays `x` and `y` store the independent and dependent variables, respectively.
- **Quadratic Fitting**: `np.polyfit(x, y, 2)` computes the coefficients for a 2nd order polynomial that best fits the data.
- **Parameters Display**: The polynomial parameters \( b2, b1, b0 \) are printed to the console.
- **Model Plotting**: Uses `np.polyval()` to evaluate the polynomial at points defined by `x1` for smooth plotting.
- **Graphical Elements**:
- **Data Points**: Displayed as black ('k') scatter points.
- **Model Curve**: Represented in red ('r'), labeled as "2nd Order Model".
- **Grid and Labels**: Enhance the plot with grid lines, and label axes for clarity. A legend differentiates the model curve from data points.](/v2/_next/image?url=https%3A%2F%2Fcontent.bartleby.com%2Fqna-images%2Fquestion%2F1f1c68a1-c113-41cc-b0cf-42f2666e5687%2F038cdb6f-6dc9-4557-be70-8fa12d095d5c%2Fncmch5k_processed.png&w=3840&q=75)
Transcribed Image Text:### Polynomial Regression: Quadratic Equation Model
#### Enter the Data
```python
x = np.array([5, 10, 15, 20])
y = np.array([15, 22, 30, 31])
```
#### Quadratic Equation Model (2nd Order Polynomial)
In this step, we fit a quadratic polynomial (2nd order) to the data.
Equation: \( y = b0 + b1 \times x + b2 \times x^2 \)
```python
b = np.polyfit(x, y, 2)
print("2nd order polynomial parameters:\nb2 =", b[0], "\nb1 =", b[1], "\nb0 =", b[2])
```
#### Plot the Model
We create a smooth line representing the polynomial model.
```python
x1 = np.linspace(5, 20, 100)
pylab.plot(x1, np.polyval(b, x1), c = "r", label = "2nd Order Model")
```
#### Plot the Data
Visualize the original data points and the fitted polynomial model.
```python
pylab.scatter(x, y, c = 'k')
pylab.grid()
pylab.xlabel("x")
pylab.ylabel("y")
pylab.legend()
```
### Explanation of Code
- **Data Entry**: Arrays `x` and `y` store the independent and dependent variables, respectively.
- **Quadratic Fitting**: `np.polyfit(x, y, 2)` computes the coefficients for a 2nd order polynomial that best fits the data.
- **Parameters Display**: The polynomial parameters \( b2, b1, b0 \) are printed to the console.
- **Model Plotting**: Uses `np.polyval()` to evaluate the polynomial at points defined by `x1` for smooth plotting.
- **Graphical Elements**:
- **Data Points**: Displayed as black ('k') scatter points.
- **Model Curve**: Represented in red ('r'), labeled as "2nd Order Model".
- **Grid and Labels**: Enhance the plot with grid lines, and label axes for clarity. A legend differentiates the model curve from data points.
Expert Solution

This question has been solved!
Explore an expertly crafted, step-by-step solution for a thorough understanding of key concepts.
This is a popular solution!
Trending now
This is a popular solution!
Step by step
Solved in 4 steps with 3 images

Knowledge Booster
Learn more about
Need a deep-dive on the concept behind this application? Look no further. Learn more about this topic, computer-science and related others by exploring similar questions and additional content below.Recommended textbooks for you
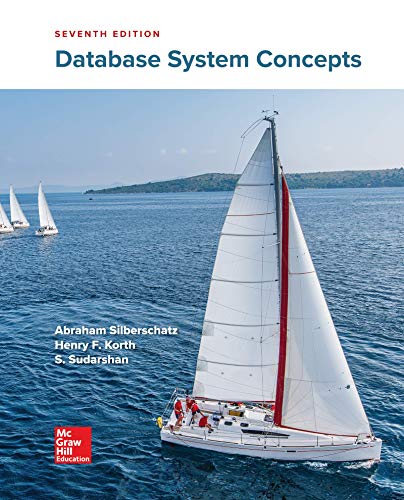
Database System Concepts
Computer Science
ISBN:
9780078022159
Author:
Abraham Silberschatz Professor, Henry F. Korth, S. Sudarshan
Publisher:
McGraw-Hill Education
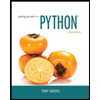
Starting Out with Python (4th Edition)
Computer Science
ISBN:
9780134444321
Author:
Tony Gaddis
Publisher:
PEARSON
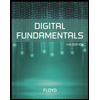
Digital Fundamentals (11th Edition)
Computer Science
ISBN:
9780132737968
Author:
Thomas L. Floyd
Publisher:
PEARSON
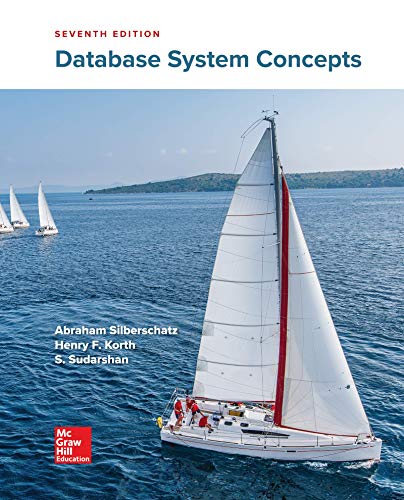
Database System Concepts
Computer Science
ISBN:
9780078022159
Author:
Abraham Silberschatz Professor, Henry F. Korth, S. Sudarshan
Publisher:
McGraw-Hill Education
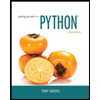
Starting Out with Python (4th Edition)
Computer Science
ISBN:
9780134444321
Author:
Tony Gaddis
Publisher:
PEARSON
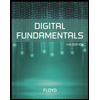
Digital Fundamentals (11th Edition)
Computer Science
ISBN:
9780132737968
Author:
Thomas L. Floyd
Publisher:
PEARSON
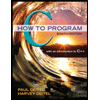
C How to Program (8th Edition)
Computer Science
ISBN:
9780133976892
Author:
Paul J. Deitel, Harvey Deitel
Publisher:
PEARSON
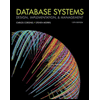
Database Systems: Design, Implementation, & Manag…
Computer Science
ISBN:
9781337627900
Author:
Carlos Coronel, Steven Morris
Publisher:
Cengage Learning
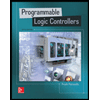
Programmable Logic Controllers
Computer Science
ISBN:
9780073373843
Author:
Frank D. Petruzella
Publisher:
McGraw-Hill Education