2. (a) Let f be defined for all (x, y) by fƒ (x, y) = x'+y' -3xy. Show that (0, 0) and (1, 1) are the only stationary points, and compute the quadratic form in (9) for f at the stationary points. (b) Check the definiteness of the quadratic form at the stationary points.
2. (a) Let f be defined for all (x, y) by fƒ (x, y) = x'+y' -3xy. Show that (0, 0) and (1, 1) are the only stationary points, and compute the quadratic form in (9) for f at the stationary points. (b) Check the definiteness of the quadratic form at the stationary points.
Advanced Engineering Mathematics
10th Edition
ISBN:9780470458365
Author:Erwin Kreyszig
Publisher:Erwin Kreyszig
Chapter2: Second-order Linear Odes
Section: Chapter Questions
Problem 1RQ
Related questions
Topic Video
Question
Can you help with question 2?
Notes (1)-(3) for question 2c are attached for reference.
![SECTION 3.3/ EQUALITY CONSTRAINTS: THE LAGRANGE PROBLEM
115
ROB
MS FOR SECTION 3 2
SM 1. The function
f(x1, x2, x3) = x} + x3 + 3x3
Extx + Ex1x7+ 7x]x –
defined on R has ohly one stationary point. Show that it is a local minimum point.
2. (a) Let f be defined for all (x, y) by ƒ (x, y) = x+y³-3xy. Show that (0, 0) and (1, 1) are the
only stationary points, and compute the quadratic form in (9) for ƒ at the stationary points.
(b) Check the definiteness of the quadratic form at the stationary points.
(c) Classify the stationary points by using (1)(3).
SM 3. Classify the stationary points of
(a) f(x, y, z) = x² +x?y + y²z+z? – 4z
(b) f(x1, x2, x3, X4) = 20x2 + 48x3 + 6x4 + 8x,.x2 – 4x} – 12x3 – x; – 4x3
HARDER PROBLEMS
4. Suppose f(x, y) has only one stationary point (x*, y*) which is a local minimum point. Is
(x*, y*) necessarily a global minimum point? It may be surprising that the answer is no. Prove
this by examining the function defined for all (x, y) by f (x, y) = (1 +y)³x²+y². (Hint: Look
at f(x, –2) as x → .)
3.3 Equality Constraints: The Lagrange Problem
A general optimization problem with equality constraints is of the form
max (min) f (x1, ..., Xn) subject to
1q = ("x'· · 'Ix)18
(u > w)
(1)
"q = ("x' · 'x) 48
where the b; are constants. We assume that m < n because otherwise there are usually no
degrees of freedom.
In vector formulation, the problem is
12:5
4/18](/v2/_next/image?url=https%3A%2F%2Fcontent.bartleby.com%2Fqna-images%2Fquestion%2F3143ece0-b2b2-417b-825f-ab4e468fd03e%2F8ef81423-3e25-4060-899d-8e0122d11124%2Fv8b3hxj_processed.jpeg&w=3840&q=75)
Transcribed Image Text:SECTION 3.3/ EQUALITY CONSTRAINTS: THE LAGRANGE PROBLEM
115
ROB
MS FOR SECTION 3 2
SM 1. The function
f(x1, x2, x3) = x} + x3 + 3x3
Extx + Ex1x7+ 7x]x –
defined on R has ohly one stationary point. Show that it is a local minimum point.
2. (a) Let f be defined for all (x, y) by ƒ (x, y) = x+y³-3xy. Show that (0, 0) and (1, 1) are the
only stationary points, and compute the quadratic form in (9) for ƒ at the stationary points.
(b) Check the definiteness of the quadratic form at the stationary points.
(c) Classify the stationary points by using (1)(3).
SM 3. Classify the stationary points of
(a) f(x, y, z) = x² +x?y + y²z+z? – 4z
(b) f(x1, x2, x3, X4) = 20x2 + 48x3 + 6x4 + 8x,.x2 – 4x} – 12x3 – x; – 4x3
HARDER PROBLEMS
4. Suppose f(x, y) has only one stationary point (x*, y*) which is a local minimum point. Is
(x*, y*) necessarily a global minimum point? It may be surprising that the answer is no. Prove
this by examining the function defined for all (x, y) by f (x, y) = (1 +y)³x²+y². (Hint: Look
at f(x, –2) as x → .)
3.3 Equality Constraints: The Lagrange Problem
A general optimization problem with equality constraints is of the form
max (min) f (x1, ..., Xn) subject to
1q = ("x'· · 'Ix)18
(u > w)
(1)
"q = ("x' · 'x) 48
where the b; are constants. We assume that m < n because otherwise there are usually no
degrees of freedom.
In vector formulation, the problem is
12:5
4/18

Transcribed Image Text:oy%20Knut%20Sydsaeter,Peter%20Hammond,Atle%20Seierstad, Arne%20Strom%20(z-lib.org).pdf
We next study conditions that allow the stationary points of a function of n variables to be
classified as local maximum points, local minimum points, and saddle points. First recall the
second-order conditions for local extreme points for functions of two variables. If f (x, y)
is a C2 function with (x*, y*) as an interior stationary point, then
12)
| SH(**, y*) f**, y*)|
| *, y°) f*, y" |
fi(*, y") > 0 &
>0 = local min. at (x*, y*) (1)
| K**, y") f(**, y") |
|f(**, y) f*, y*) |
| S(**, y") fi(**, y*) |
|**, y*) f**, y") |
>0 = local max. at (x*, y*) (2)
<0 = (x*, y*) is a saddle point
(3)
In order to generalize these results to functions of n variables, we need to consider the n
leading principal minors of the Hessian matrix f"(x) = (f#(x))nxn:
(x)f (x) f
(x) (x) S
(x) f
(x)
k = 1,..., n
= (x)a
(t)
(x) S
THEOREM 3 2.1 (LOCAL SECOND-ORDER CONDITIONS).
(x)l (x)
Suppose that f (x) = ƒ(x1, .., Xn) is defined on a set S in R" and that x* is an
interior stationary point. Assume also that f is C² in an open ball around x*. Let
Dr(x) be defined by (4). Then:
(a) Dr(x*) > 0, k = 1,...,n
x* is a local minimum point.
(b) (-1)* DE(x*) > 0, k = 1,..., n
x* is a local maximum point.
(c) Dn(x*) #0 and neither (a) nor (b) is satisfied
x* is a saddle point.
Show
12:51 PM
4/18/202
Expert Solution

This question has been solved!
Explore an expertly crafted, step-by-step solution for a thorough understanding of key concepts.
Step by step
Solved in 4 steps

Knowledge Booster
Learn more about
Need a deep-dive on the concept behind this application? Look no further. Learn more about this topic, advanced-math and related others by exploring similar questions and additional content below.Recommended textbooks for you
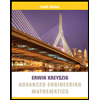
Advanced Engineering Mathematics
Advanced Math
ISBN:
9780470458365
Author:
Erwin Kreyszig
Publisher:
Wiley, John & Sons, Incorporated
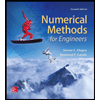
Numerical Methods for Engineers
Advanced Math
ISBN:
9780073397924
Author:
Steven C. Chapra Dr., Raymond P. Canale
Publisher:
McGraw-Hill Education
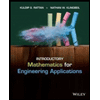
Introductory Mathematics for Engineering Applicat…
Advanced Math
ISBN:
9781118141809
Author:
Nathan Klingbeil
Publisher:
WILEY
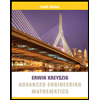
Advanced Engineering Mathematics
Advanced Math
ISBN:
9780470458365
Author:
Erwin Kreyszig
Publisher:
Wiley, John & Sons, Incorporated
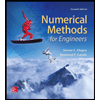
Numerical Methods for Engineers
Advanced Math
ISBN:
9780073397924
Author:
Steven C. Chapra Dr., Raymond P. Canale
Publisher:
McGraw-Hill Education
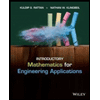
Introductory Mathematics for Engineering Applicat…
Advanced Math
ISBN:
9781118141809
Author:
Nathan Klingbeil
Publisher:
WILEY
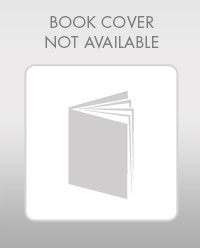
Mathematics For Machine Technology
Advanced Math
ISBN:
9781337798310
Author:
Peterson, John.
Publisher:
Cengage Learning,
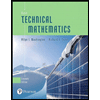
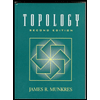