14. Use the JMP output on page 8 to answer question 14. 1. Which model is the "best" at predicting response, y? Justification: 2. Using the parameter estimates in the JMP output, is there a problem with multicollinearity? YES NO CANNOT ASSESS Justification: What other method(s) may be used to assess multicollinearity? c. Examine Plots A and B in Figure 4. What could have happened to the data used to create Plot A to now produce Plot B? Assuming LR assumptions, which plot is the more desired residual plot? d. Assuming an MLR model meets the required assumptions, sketch an appropriate histogram and normal probability plot of its residuals. Model Number RSquare RMSE Cp x2 1 0.9566 19.1669 2.0888 x3 1 0.7637 44.7511 82.6080 x1 1 0.0257 90.8632 390.5179 x2,x3 2 0.9604 18.8612 2.5432 х1,x2 2 0.9578 19.4661 3.6213 х1,х3 2 0.7637 46.0439 84.5881 х1,x2,х3 3 0.9617 19.1198 4.0 Figure 2: Table of Possible Predictive Models Parameter Estimates Term Estimate Std Error t Ratio Prob> It| VIF Intercept 9.6002837 38.54101 0.25 0.8067 х1 -1.216878 1.188857 -1.02 0.3223 1.0660932 x2 5.3978072 0.56296 9.59 <.0001* 3.9067774 x3 -1.374817 1.720724 -0.80 0.4368 15.600942 x4 1.3419933 0.789778 1.70 0.1099 13.067958 Figure 3: Parameter Estimates for x1, x2, x3, and x4
14. Use the JMP output on page 8 to answer question 14. 1. Which model is the "best" at predicting response, y? Justification: 2. Using the parameter estimates in the JMP output, is there a problem with multicollinearity? YES NO CANNOT ASSESS Justification: What other method(s) may be used to assess multicollinearity? c. Examine Plots A and B in Figure 4. What could have happened to the data used to create Plot A to now produce Plot B? Assuming LR assumptions, which plot is the more desired residual plot? d. Assuming an MLR model meets the required assumptions, sketch an appropriate histogram and normal probability plot of its residuals. Model Number RSquare RMSE Cp x2 1 0.9566 19.1669 2.0888 x3 1 0.7637 44.7511 82.6080 x1 1 0.0257 90.8632 390.5179 x2,x3 2 0.9604 18.8612 2.5432 х1,x2 2 0.9578 19.4661 3.6213 х1,х3 2 0.7637 46.0439 84.5881 х1,x2,х3 3 0.9617 19.1198 4.0 Figure 2: Table of Possible Predictive Models Parameter Estimates Term Estimate Std Error t Ratio Prob> It| VIF Intercept 9.6002837 38.54101 0.25 0.8067 х1 -1.216878 1.188857 -1.02 0.3223 1.0660932 x2 5.3978072 0.56296 9.59 <.0001* 3.9067774 x3 -1.374817 1.720724 -0.80 0.4368 15.600942 x4 1.3419933 0.789778 1.70 0.1099 13.067958 Figure 3: Parameter Estimates for x1, x2, x3, and x4
MATLAB: An Introduction with Applications
6th Edition
ISBN:9781119256830
Author:Amos Gilat
Publisher:Amos Gilat
Chapter1: Starting With Matlab
Section: Chapter Questions
Problem 1P
Related questions
Question
please show work

Transcribed Image Text:14. Use the JMP output on page 8 to answer question 14.
1. Which model is the "best" at predicting response, y?
Justification:
2. Using the parameter estimates in the JMP output, is there a
problem with multicollinearity?
YES NO CANNOT ASSESS
Justification:
What other method(s) may be used to assess multicollinearity?
c. Examine Plots A and B in Figure 4. What could have happened to
the data used to create Plot A to now produce Plot B?
Assuming LR assumptions, which plot is the more desired residual
plot?
d. Assuming an MLR model meets the required assumptions, sketch
an appropriate histogram and normal probability plot of its
residuals.
Model
Number RSquare
RMSE
Cp
x2
1
0.9566 19.1669
2.0888
x3
1
0.7637 44.7511
82.6080
x1
0.0257 90.8632
390.5179
x2,x3
2
0.9604 18.8612
2.5432
3.6213
х1,x2
х1,x3
2
0.9578 19.4661
2
0.7637 46.0439
84.5881
х1,х2,х3
3
0.9617 19.1198
4.0000
Figure 2: Table of Possible Predictive Models
Parameter Estimates
Term
Estimate Std Error t Ratio Prob>|t|
VIF
Intercept 9.6002837 38.54101
0.25
0.8067
x1
-1.216878
1.188857
-1.02
0.3223
1.0660932
x2
5.3978072
0.56296
9.59
<.0001* 3.9067774
x3
-1.374817
1.720724
-0.80
0.4368
15.600942
х4
1.3419933 0.789778
1.70
0.1099
13.067958
Figure 3: Parameter Estimates for x1, x2, x3, and x4
8.
6-
6-
Positive
4.
4-
Negative
-6 Negative
-6-
-8
30.0
32.5
35.0
-8
30.0
32.5
35.0
Figure 4: Studentized Residual Plots A (Left) and B (Right)
SLR Residual in Y
QR Residual in Y
Expert Solution

This question has been solved!
Explore an expertly crafted, step-by-step solution for a thorough understanding of key concepts.
This is a popular solution!
Trending now
This is a popular solution!
Step by step
Solved in 2 steps with 1 images

Knowledge Booster
Learn more about
Need a deep-dive on the concept behind this application? Look no further. Learn more about this topic, statistics and related others by exploring similar questions and additional content below.Recommended textbooks for you
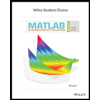
MATLAB: An Introduction with Applications
Statistics
ISBN:
9781119256830
Author:
Amos Gilat
Publisher:
John Wiley & Sons Inc
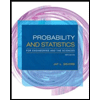
Probability and Statistics for Engineering and th…
Statistics
ISBN:
9781305251809
Author:
Jay L. Devore
Publisher:
Cengage Learning
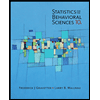
Statistics for The Behavioral Sciences (MindTap C…
Statistics
ISBN:
9781305504912
Author:
Frederick J Gravetter, Larry B. Wallnau
Publisher:
Cengage Learning
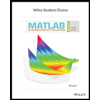
MATLAB: An Introduction with Applications
Statistics
ISBN:
9781119256830
Author:
Amos Gilat
Publisher:
John Wiley & Sons Inc
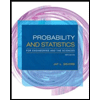
Probability and Statistics for Engineering and th…
Statistics
ISBN:
9781305251809
Author:
Jay L. Devore
Publisher:
Cengage Learning
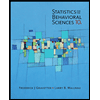
Statistics for The Behavioral Sciences (MindTap C…
Statistics
ISBN:
9781305504912
Author:
Frederick J Gravetter, Larry B. Wallnau
Publisher:
Cengage Learning
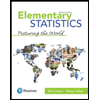
Elementary Statistics: Picturing the World (7th E…
Statistics
ISBN:
9780134683416
Author:
Ron Larson, Betsy Farber
Publisher:
PEARSON
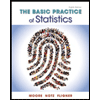
The Basic Practice of Statistics
Statistics
ISBN:
9781319042578
Author:
David S. Moore, William I. Notz, Michael A. Fligner
Publisher:
W. H. Freeman
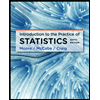
Introduction to the Practice of Statistics
Statistics
ISBN:
9781319013387
Author:
David S. Moore, George P. McCabe, Bruce A. Craig
Publisher:
W. H. Freeman