120 110 100 90 80 70 0.0 0.5 1.0 Sample -1.0 -2.0 O O 60 -1.5 O O Scatterplot for average O 80 -1.0 O 100 -0.5 Body Weight (kg) O Q-Q Plot O 120 0.0 0.5 Theoretical Quantiles O 1.0 T 140 1.5 O stdres 0.0 0.5 1.0 -1.0 -2.0 stdres 1.0 0.5 -0.5 0.0 -1.0 -2.0 -1.5 Std residuals versus explanatory variable O 60 O 80 O 80 O O 90 Body Weight (kg) Std residuals versus fits O 100 O T 100 fits O 120 110 120 140 O T 130
120 110 100 90 80 70 0.0 0.5 1.0 Sample -1.0 -2.0 O O 60 -1.5 O O Scatterplot for average O 80 -1.0 O 100 -0.5 Body Weight (kg) O Q-Q Plot O 120 0.0 0.5 Theoretical Quantiles O 1.0 T 140 1.5 O stdres 0.0 0.5 1.0 -1.0 -2.0 stdres 1.0 0.5 -0.5 0.0 -1.0 -2.0 -1.5 Std residuals versus explanatory variable O 60 O 80 O 80 O O 90 Body Weight (kg) Std residuals versus fits O 100 O T 100 fits O 120 110 120 140 O T 130
Algebra & Trigonometry with Analytic Geometry
13th Edition
ISBN:9781133382119
Author:Swokowski
Publisher:Swokowski
Chapter5: Inverse, Exponential, And Logarithmic Functions
Section5.6: Exponential And Logarithmic Equations
Problem 6E
Related questions
Question
Could you please explain what the standard residuals in the given data imply, in context to the topic (strengths dependency on body weight). Also what the Shapiro test implies in context.

Transcribed Image Text:100 110 120
Bench press standards per class of body weight (kg) - Average
Sample Quantiles
06
80
70
0.0 0.5 1.0
-1.0
-2.0
O
O
60
Scatterplot for average
O
80
-1.5 -1.0
0
100
-0.5
Body Weight (kg)
O
Q-Q Plot
O
120
0.0 0.5
Theoretical Quantiles
O
O
1.0
140
O
1.5
O
stdres
0.0 0.5 1.0
stdres
-1.0
-2.0
1.0
0.5
0.0
-0.5
-1.5 -1.0
-2.0
Std residuals versus explanatory variable
O
T
09
o
80
O
O
80
90
O
O
Body Weight (kg)
O
Std residuals versus fits
O
100
100
O
fits
O
120
110
O
120
140
130
O

Transcribed Image Text:Residuals:
Min
-10.531 -4.989
Coefficients:
10 Median
3Q
1.869 5.267
(Intercept)
`Body Weight (kg) `
Signif. codes: 0 *** 0.001
`Body Weight (kg) `
Residuals
Estimate Std. Error t value Pr (>|t|)
42.62755
6.209 0.000157
0.62692
8.402 1.49e-05 ***
7
Residual standard error: 7.077 on 9 degrees of freedom
Multiple R-squared: 0.8869, Adjusted R-squared:
F-statistic: 70.6 on 1 and 9 DF, p-value: 1.492e-05
8
> abline (Regression.model, col=4, lwd=3)
> anova (Regression.model)
Analysis of Variance Table
6.86517
0.07461
1**/
Response: Bench press standards per class of body weight (kg) - Average
Sum Sq Mean Sq F value
Pr (>F)
2
Max
7.950
Signif. codes:
1**/
0 *** 0.001
> stdres<- rstandard (Regression.model)
> print (stdres)
1
9
Df
1 3536.0 3536.0 70.601 1.492e-05 ***
9 450.8
50.1
0.01 * 0.05 ¹.0.1 1
3
data: stdres
W = 0.91857, p-value = 0.3069
10
Shapiro-Wilk normality test
-1.4864868 -1.0645996 -0.5043759 0.2843252 0.6513863 1.1083291
1.1786018
0.8744
0.01 1*1 0.05. 0.1 1
4
0.9248828 0.5985440 -0.3245869 -2.0230776
11
5
> plot (Body Weight (kg)`, stdres, main="Std residuals versus
explanatory variable")
> fits<- fitted (Regression.model)
> plot (fits, stdres, main="Std residuals versus fits")
> qqnorm (stdres, main="Q-Q Plot")
>qqline (stdres)
> shapiro.test (stdres)
6
Expert Solution

This question has been solved!
Explore an expertly crafted, step-by-step solution for a thorough understanding of key concepts.
This is a popular solution!
Trending now
This is a popular solution!
Step by step
Solved in 3 steps with 24 images

Recommended textbooks for you
Algebra & Trigonometry with Analytic Geometry
Algebra
ISBN:
9781133382119
Author:
Swokowski
Publisher:
Cengage
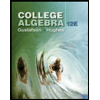
College Algebra (MindTap Course List)
Algebra
ISBN:
9781305652231
Author:
R. David Gustafson, Jeff Hughes
Publisher:
Cengage Learning
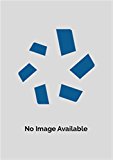
Big Ideas Math A Bridge To Success Algebra 1: Stu…
Algebra
ISBN:
9781680331141
Author:
HOUGHTON MIFFLIN HARCOURT
Publisher:
Houghton Mifflin Harcourt
Algebra & Trigonometry with Analytic Geometry
Algebra
ISBN:
9781133382119
Author:
Swokowski
Publisher:
Cengage
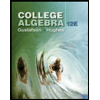
College Algebra (MindTap Course List)
Algebra
ISBN:
9781305652231
Author:
R. David Gustafson, Jeff Hughes
Publisher:
Cengage Learning
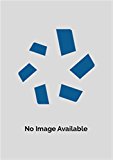
Big Ideas Math A Bridge To Success Algebra 1: Stu…
Algebra
ISBN:
9781680331141
Author:
HOUGHTON MIFFLIN HARCOURT
Publisher:
Houghton Mifflin Harcourt