1. Define an instance of Sklearn's Pipeline class which applies the standardization class and then the AddBias class. Store the output into feature_pipe. 1. Hint: Recall the Pipeline class takes in a list of tuples where each tuple contains two elements: string and a class instance. 2. Hint: You can set the first element of each tuple to whatever string you would like. 2. Call the fit_transform() method for our feature_pipe instance and pass our x_trn data to be fitted and then transformed. Store the output into x_trn_clean. 3. Call the transform() method for our feature_pipe instance and pass our x_v1d data to be transformed. Store the output into X_vld_clean. 4. Call the transform() method for our feature_pipe instance and pass our x_tst data to be transformed. Store the output into X tst clean
1. Define an instance of Sklearn's Pipeline class which applies the standardization class and then the AddBias class. Store the output into feature_pipe. 1. Hint: Recall the Pipeline class takes in a list of tuples where each tuple contains two elements: string and a class instance. 2. Hint: You can set the first element of each tuple to whatever string you would like. 2. Call the fit_transform() method for our feature_pipe instance and pass our x_trn data to be fitted and then transformed. Store the output into x_trn_clean. 3. Call the transform() method for our feature_pipe instance and pass our x_v1d data to be transformed. Store the output into X_vld_clean. 4. Call the transform() method for our feature_pipe instance and pass our x_tst data to be transformed. Store the output into X tst clean
Database System Concepts
7th Edition
ISBN:9780078022159
Author:Abraham Silberschatz Professor, Henry F. Korth, S. Sudarshan
Publisher:Abraham Silberschatz Professor, Henry F. Korth, S. Sudarshan
Chapter1: Introduction
Section: Chapter Questions
Problem 1PE
Related questions
Question
def feature_pipeline(
X_trn: pd.DataFrame,
X_vld: pd.DataFrame,
X_tst: pd.DataFrame,
) -> List[pd.DataFrame]:
""" Creates column transformers and pipelines to apply data cleaning and
transfornations to the input features of our data.
Args:
X_trn: train features
X_vld: validation features
X_tst: test features
"""
# TODO 1
feature_pipe =
# TODO 2
X_trn_clean =
# TODO 3
X_vld_clean =
# TODO 4
X_tst_clean =
return X_trn_clean, X_vld_clean, X_tst_clean
defTEST_feature_pipeline():
# Apply feature and label splitting
X, y = feature_label_split(iris_df, label_name='class')
# Apply train, validation and test set splitting
X_trn, y_trn, X_vld, y_vld, X_tst, y_tst = train_valid_test_split(X, y)
# Apply feature cleaning AFTER splitting
X_trn, X_vld, X_tst = feature_pipeline(X_trn, X_vld, X_tst)
print(f"X_trn shape: {X_trn.shape}")
print(f"X_trn type: {type(X_trn)}")
print(f"X_vld shape: {X_vld.shape}")
print(f"X_vld type: {type(X_vld)}")
print(f"X_tst shape: {X_tst.shape}")
print(f"X_tst type: {type(X_tst)}")
display(X_trn)
todo_check([
(np.all(np.isclose(X_trn.describe().loc['mean'],[1,0,0,0,0])),"'X_trn' has the wrong mean values"),
(np.all(np.isclose(X_trn.iloc[:3,4],[0.77996804,0.3865691,-0.2690958], rtol=.01)),"'X_trn' has incorrect values"),
(np.all(np.isclose(X_vld.iloc[:3,4],[-0.2690958,0.12430314,-1.31815965], rtol=.01)),"'X_vld' has incorrect values"),
(np.all(np.isclose(X_tst.iloc[:3,4],[-0.00682984,-1.18702667,1.43563294], rtol=.01)),"'X_tst' has incorrect values"),
])
TEST_feature_pipeline()
garbage_collect(['TEST_feature_pipeline'])

Transcribed Image Text:Complete the TODO by finishing the feature_pipeline () function.
1. Define an instance of Sklearn's Pipeline class which applies the standardization class and then the AddBias class. Store the output
into feature_pipe.
1. Hint: Recall the Pipeline class takes in a list of tuples where each tuple contains two elements: string and a class instance.
2. Hint: You can set the first element of each tuple to whatever string you would like.
2. Call the fit_transform() method for our feature_pipe instance and pass our x_trn data to be fitted and then transformed.Store the
output into x_trn_clean.
3. Call the transform() method for our feature_pipe instance and pass our x_vld data to be transformed. Store the output into
X_vld_clean.
4. Call the transform() method for our feature_pipe instance and pass our x_tst data to be transformed. Store the output into
X_tst_clean.
Expert Solution

This question has been solved!
Explore an expertly crafted, step-by-step solution for a thorough understanding of key concepts.
This is a popular solution!
Trending now
This is a popular solution!
Step by step
Solved in 2 steps

Knowledge Booster
Learn more about
Need a deep-dive on the concept behind this application? Look no further. Learn more about this topic, computer-science and related others by exploring similar questions and additional content below.Recommended textbooks for you
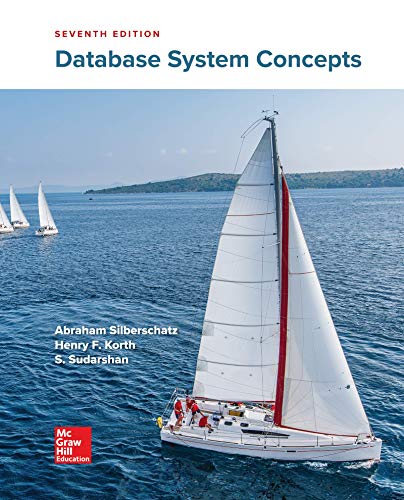
Database System Concepts
Computer Science
ISBN:
9780078022159
Author:
Abraham Silberschatz Professor, Henry F. Korth, S. Sudarshan
Publisher:
McGraw-Hill Education
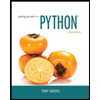
Starting Out with Python (4th Edition)
Computer Science
ISBN:
9780134444321
Author:
Tony Gaddis
Publisher:
PEARSON
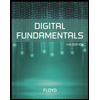
Digital Fundamentals (11th Edition)
Computer Science
ISBN:
9780132737968
Author:
Thomas L. Floyd
Publisher:
PEARSON
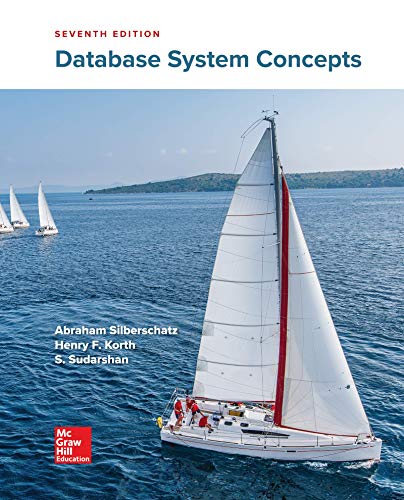
Database System Concepts
Computer Science
ISBN:
9780078022159
Author:
Abraham Silberschatz Professor, Henry F. Korth, S. Sudarshan
Publisher:
McGraw-Hill Education
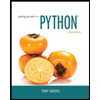
Starting Out with Python (4th Edition)
Computer Science
ISBN:
9780134444321
Author:
Tony Gaddis
Publisher:
PEARSON
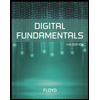
Digital Fundamentals (11th Edition)
Computer Science
ISBN:
9780132737968
Author:
Thomas L. Floyd
Publisher:
PEARSON
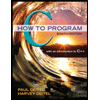
C How to Program (8th Edition)
Computer Science
ISBN:
9780133976892
Author:
Paul J. Deitel, Harvey Deitel
Publisher:
PEARSON
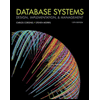
Database Systems: Design, Implementation, & Manag…
Computer Science
ISBN:
9781337627900
Author:
Carlos Coronel, Steven Morris
Publisher:
Cengage Learning
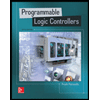
Programmable Logic Controllers
Computer Science
ISBN:
9780073373843
Author:
Frank D. Petruzella
Publisher:
McGraw-Hill Education