Edited SAS Output (PROC REG) for Regression of WGT on HGT Source Model Error Corrected Total OF Sum of Squares Mean Square 1 588.9225232 588 9225232 299327477 299 3274760 868.2500000 10 11 A SAY SESSYSSE "Partial Fstatistics will be discussed in Chapter 9 on hypothesis testing R-Square Coeff Var Root MSE 0.663014 8.718857 5.471083 Fstatistic for overaltet F Value Pr>F 19.67 0.0013 8.8 Numerical Examples 14 WOT Mean overall test (continued) 62.75000 Model 2 WGT = Bo + B₂AGE + E Edited SAS Output (PROC REG) for Regression of WGT on AGE Source Model Error Corrected Total OF Parameter Intercept AGE 1 10 11 R-Square 0.502618 Sum of Squares 526 3028571 361.8571429 888.2500000 Coeff Var 9.566305 Mean Square F Value 526 3028571 14.55 36.1857143 Root MSE 6015456 Estimate Standard Error Value PDX 30.57142857 8.61370526 3.64285714 095511512 3.55 0.0053 3.81 0.0034 WGT Mean 62.75000 Pr>F 0.0034 Model 3 WGT=B₁ + B₂(AGE)² + E Edited SAS Output (PROC REG) for Regressi Cigle 2013 Compage L 150 Source Model Error Corrected Total OF Sum of Squa 521.9320 366.3179 888 2500 Coeff Var 9.645292 10 11 R-Square 0.587506 Al Mayed in Chapter 8 Multiple Regression Analysis: General C Parameter Estimate Stan
Edited SAS Output (PROC REG) for Regression of WGT on HGT Source Model Error Corrected Total OF Sum of Squares Mean Square 1 588.9225232 588 9225232 299327477 299 3274760 868.2500000 10 11 A SAY SESSYSSE "Partial Fstatistics will be discussed in Chapter 9 on hypothesis testing R-Square Coeff Var Root MSE 0.663014 8.718857 5.471083 Fstatistic for overaltet F Value Pr>F 19.67 0.0013 8.8 Numerical Examples 14 WOT Mean overall test (continued) 62.75000 Model 2 WGT = Bo + B₂AGE + E Edited SAS Output (PROC REG) for Regression of WGT on AGE Source Model Error Corrected Total OF Parameter Intercept AGE 1 10 11 R-Square 0.502618 Sum of Squares 526 3028571 361.8571429 888.2500000 Coeff Var 9.566305 Mean Square F Value 526 3028571 14.55 36.1857143 Root MSE 6015456 Estimate Standard Error Value PDX 30.57142857 8.61370526 3.64285714 095511512 3.55 0.0053 3.81 0.0034 WGT Mean 62.75000 Pr>F 0.0034 Model 3 WGT=B₁ + B₂(AGE)² + E Edited SAS Output (PROC REG) for Regressi Cigle 2013 Compage L 150 Source Model Error Corrected Total OF Sum of Squa 521.9320 366.3179 888 2500 Coeff Var 9.645292 10 11 R-Square 0.587506 Al Mayed in Chapter 8 Multiple Regression Analysis: General C Parameter Estimate Stan
MATLAB: An Introduction with Applications
6th Edition
ISBN:9781119256830
Author:Amos Gilat
Publisher:Amos Gilat
Chapter1: Starting With Matlab
Section: Chapter Questions
Problem 1P
Related questions
Question
Answer d-f

Transcribed Image Text:### Educational Content on Regression Models
#### Overview of Regression Analysis
Regression analysis is a powerful statistical method that allows examining the relationship between two or more variables. In these examples, we focus on modeling the weight (WGT) using height (HGT) and age (AGE) as predictors.
---
#### Model 1: Simple Linear Regression of WGT on HGT
**Model:** \( WGT = \beta_0 + \beta_1HGT + E \)
- **Source** | **DF** | **Sum of Squares** | **Mean Square** | **F Value** | **Pr > F**
- Model | 1 | 698.5921523 | 698.5921523 | 19.87 | 0.0007
- Error | 10 | 351.2922727 | 35.1292273
- Corrected Total | 11 | 1049.884424
- **R-Square:** 0.665194
- **Coeff Var:** 8.718657
- **Root MSE:** 5.929377
- **WGT Mean:** 67.75000
- **Parameter Estimates:**
- Intercept | Estimate: 37.45172135 | Standard Error: 23.1278460 | t Value: 1.62 | Pr > |t|: 0.1374
- HGT | Estimate: 1.07225306 | Standard Error: 0.24137006 | t Value: 4.45 | Pr > |t|: 0.0013
*Note: Partial F-statistics will be discussed in Chapter 9 on hypothesis testing.*
---
#### Model 2: Simple Linear Regression of WGT on AGE
**Model:** \( WGT = \beta_0 + \beta_1AGE + E \)
- Source | DF | Sum of Squares | Mean Square | F Value | Pr > F
- Model | 1 | 508.3568721 | 508.3568721 | 14.55 | 0.0034
- Error | 10 | 351.5277839 | 35.1527784
- Corrected Total | 11 | 888.2800000
- R-Square: 0.652816
- Coeff Var: 8.053660

Transcribed Image Text:1. Consider the numerical examples given in Section 8.8 of Chapter 8, involving assessment of the relationship of the independent variables HGT, AGE, and (AGE)² to the dependent variable WGT. Suppose that HGT is the independent variable of primary concern, so interest lies in evaluating the relationship of HGT to WGT, controlling for the possible confounding effects of AGE and (AGE)².
a. Assuming that no interaction of any kind exists, state an appropriate regression model to use as the baseline (i.e., standard) for decisions about confounding.
b. Using an appropriate regression coefficient given in part (a) as your measure of association, determine whether confounding exists due to AGE and/or (AGE)².
c. Can (AGE)² be dropped from your initial model in part (a) because it is not needed to control adequately for confounding? Explain your answer (using a regression coefficient as your measure of association).
d. Should (AGE)² be retained in the final model for the sake of precision? Explain.
e. In light of both confounding and precision, what should be your final model? Why?
f. How would you modify your initial model in part (a) to allow for assessing interactions?
g. Regarding your answer to part (f), how would you test for interaction?
Expert Solution

This question has been solved!
Explore an expertly crafted, step-by-step solution for a thorough understanding of key concepts.
This is a popular solution!
Trending now
This is a popular solution!
Step by step
Solved in 5 steps with 6 images

Recommended textbooks for you
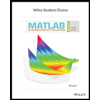
MATLAB: An Introduction with Applications
Statistics
ISBN:
9781119256830
Author:
Amos Gilat
Publisher:
John Wiley & Sons Inc
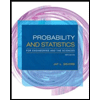
Probability and Statistics for Engineering and th…
Statistics
ISBN:
9781305251809
Author:
Jay L. Devore
Publisher:
Cengage Learning
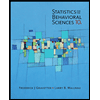
Statistics for The Behavioral Sciences (MindTap C…
Statistics
ISBN:
9781305504912
Author:
Frederick J Gravetter, Larry B. Wallnau
Publisher:
Cengage Learning
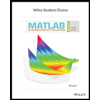
MATLAB: An Introduction with Applications
Statistics
ISBN:
9781119256830
Author:
Amos Gilat
Publisher:
John Wiley & Sons Inc
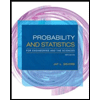
Probability and Statistics for Engineering and th…
Statistics
ISBN:
9781305251809
Author:
Jay L. Devore
Publisher:
Cengage Learning
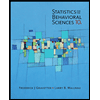
Statistics for The Behavioral Sciences (MindTap C…
Statistics
ISBN:
9781305504912
Author:
Frederick J Gravetter, Larry B. Wallnau
Publisher:
Cengage Learning
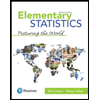
Elementary Statistics: Picturing the World (7th E…
Statistics
ISBN:
9780134683416
Author:
Ron Larson, Betsy Farber
Publisher:
PEARSON
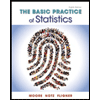
The Basic Practice of Statistics
Statistics
ISBN:
9781319042578
Author:
David S. Moore, William I. Notz, Michael A. Fligner
Publisher:
W. H. Freeman
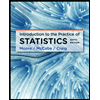
Introduction to the Practice of Statistics
Statistics
ISBN:
9781319013387
Author:
David S. Moore, George P. McCabe, Bruce A. Craig
Publisher:
W. H. Freeman