Lab 5_SR_rev
docx
keyboard_arrow_up
School
University of Alberta *
*We aren’t endorsed by this school
Course
505
Subject
Statistics
Date
Apr 3, 2024
Type
docx
Pages
12
Uploaded by ChiefRhinoceros2142
1
To receive full credit for Lab 5, submit the Google form https://forms.gle/gwfQuCm2bt8JrMjV7
by 11:20a.m. on April 7, 2022 and the Word document on e-class by Tuesday, April 12, 2022.
Lab 5 Repeated Measures
Repeated Measures: Model and assumptions
Basic repeated measures model expressed using regression
y
=
β
0
+
∑
i
=
1
T
−
1
β
i
∗
time dummycode
i
+
∑
j
=
1
N
−
1
β
j
+
T
−
1
∗
subject dummy code
j
+
∑
i
=
1
T
−
1
∑
j
=
1
N
−
1
β
for eachterm
∗
time dummy code
i
∗
subject
Degree of freedom: there are total N*T data points, intercept costs 1 df, time costs T-1 df, subject
costs N-1 df, interaction costs (N-1)*(T-1)=N*T-N-T+1 df, error has 0 df. We cannot separate error terms from the interaction term. SS works the same as before. But it is important to note that even though repeated measure models look similar to regression models we learned before, they are not exactly the same. This is because the subject factor is considered as a random factor
rather than a fixed factor. This influences how the F ratio is calculated for each factor. This is why a repeated measures model is considered a mixed design (it has a random factor (subject) and a fixed factor (time)).
Note that SPSS uses a multivariate approach (each time point is considered a DV) to solve the repeated measure. But the univariate approach (from the lecture) is equivalent to the multivariate approach if the sphericity assumption is met. However, for now, we will not focus on the multivariate approach, which is discussed in EDPY 605.
Assumptions
Homogeneity of variance covariance matrix: different groups have the same population variance covariance matrix.
Sphericity: The simple way to define sphericity is that the variances of the differences between any two time points are the same. This definition is not always correct, but for simplicity, we just want to state it this way. Intuitively, if we suspect change between time 1 and time 2 predicts change between time 2 and time 3, we know the assumption of sphericity is probably violated.
Normality assumption: repeated measure in SPSS actually assumes that the DVs have a multivariate normal distribution, which means any linear combinations of the DVs must be normally distributed (explained in EDPY 605).
2
Exercise 1: One-way Within Subjects Design
A researcher wants to examine the effect of a psychotherapy on participants’ happiness. The researcher measures participants’ happiness before the treatment, immediately after the treatment, and 1 month after the treatment. Open exercise 1.sav, click Analyze, General Linear Model, Repeated Measures, in the “Within-Subject Factor Name” box, type time, in the “number of levels” box, type 3, Add, click Define, move pre, post, and follow up to the within-subjects variables (time), click Plots, move time to the horizontal axis box, add, continue, click EM Means, move time to “Display Means for” box, check “Compare main effects”, Continue, Options, check Descriptive statistics, Estimates of effect size, continue, ok.
Mauchly's Test of Sphericity
a
Measure: MEASURE_1 Within Subjects Effect
Mauchly's
W
Approx. Chi-
Square
df
Sig.
Epsilon
b
Greenhouse-
Geisser
Huynh-
Feldt
Lower-bound
time
.958
.775
2
.679
.960
1.000
.500
Tests the null hypothesis that the error covariance matrix of the orthonormalized transformed dependent variables is
proportional to an identity matrix.
a. Design: Intercept Within Subjects Design: time
b. May be used to adjust the degrees of freedom for the averaged tests of significance. Corrected tests are displayed in the Tests of Within-Subjects Effects table.
A.
Interpret Mauchly’s Test of Sphericity. Has the assumption of sphericity been violated? Report the results. [Hint: Google how to do this. A correct answer must include both an interpretation and the corresponding statistics.]
No, the assumption of sphericity was met, χ2 (2) = 0.775, p = .679.
if not violated then you do not need to look at greenhouse-geisser
B.
Which website did you use to help you interpret this test?
https://statistics.laerd.com/statistical-guides/sphericity-statistical-guide.php
3
Tests of Within-Subjects Effects
Measure: MEASURE_1 Source
Type III Sum
of Squares
df
Mean Square
F
Sig.
Partial Eta
Squared
time
Sphericity Assumed
9151.300
2
4575.650
143.773
.000
.883
Greenhouse-Geisser
9151.300
1.919
4768.520
143.773
.000
.883
Huynh-Feldt
9151.300
2.000
4575.650
143.773
.000
.883
Lower-bound
9151.300
1.000
9151.300
143.773
.000
.883
Error(time)
Sphericity Assumed
1209.367
38
31.825
Greenhouse-Geisser
1209.367
36.463
33.167
Huynh-Feldt
1209.367
38.000
31.825
Lower-bound
1209.367
19.000
63.651
C.
Does time have a significant effect on participants’ happiness?
Yes, time has a significant effect on participants’ happiness, F(2, 38) = 143.733, p < .001, ηp2 = .883.
Your preview ends here
Eager to read complete document? Join bartleby learn and gain access to the full version
- Access to all documents
- Unlimited textbook solutions
- 24/7 expert homework help
4
3 time points, treatment is in the middle, pre, treatment (when it’s the highest, showing effect), post(higher than pre)
Pairwise Comparisons
Measure: MEASURE_1 (I) time
(J) time
Mean Difference
(I-J)
Std. Error
Sig.
b
95% Confidence Interval for
Difference
b
Lower Bound
Upper Bound
1
2
-30.250
*
1.599
.000
-33.597
-26.903
3
-15.350
*
1.913
.000
-19.354
-11.346
2
1
30.250
*
1.599
.000
26.903
33.597
3
14.900
*
1.825
.000
11.080
18.720
3
1
15.350
*
1.913
.000
11.346
19.354
2
-14.900
*
1.825
.000
-18.720
-11.080
Based on estimated marginal means
*. The mean difference is significant at the .05 level.
b. Adjustment for multiple comparisons: Least Significant Difference (equivalent to no adjustments).
D.
Based on the post hoc analysis, how do the three time points differ from each other? [Hint: Take a look at the plot and identify the corresponding values in the Pairwise Comparisons output.]
There was a statistically significant difference in participants’ happiness between pre-treatment and immediately after the treatment (p < .001). The mean difference is 30.250. There was a statistically significant difference in participants’ happiness between pre-treatment and 1 month after the treatment (p < .001). The mean difference is 15.350. There was a statistically significant difference in participants’ happiness between immediately after the treatment and 1 month after the treatment (p < .001). The mean difference is 14.900.
Exercise 2: Two-way Within Subjects Design
This example comes from the lecture 9 notes. We examine the effect of time and type of novel on a dependent variable. [In the lecture, Seyma said she would refer to the DV as “number of books.”]
Open ‘exercise 2.sav’, click Analyze, General Linear Model, Repeated Measures, write “type”
in the within-subject factor name box, type 2 in the number of levels box, click add, type month in the within-subject factor name box, type 3 in the number of levels box, click add, define, move all the variables to the “within-subjects variables” box, click plots, move month to the horizontal axis box, move type to the separate lines box, add, continue, click EM Means,
move type, month, and type*month to the “display means for” box, check “compare main effects”, Continue, Options, check Descriptive statistics, estimates of effect size, continue, paste, after the line ‘/EMMEANS=TABLES(type*month)’ add ‘COMPARE (type)
5
ADJ(LSD)’, run the code.
Descriptive Statistics
Mean
Std. Deviation
N
Science Fiction month 1
2.4000
1.67332
5
Science Fiction month 2
3.8000
.83666
5
Science Fiction month 3
6.4000
1.14018
5
Mystery month 1
5.0000
1.87083
5
Mystery month 2
5.0000
2.23607
5
Mystery month 3
2.2000
1.64317
5
Mauchly's Test of Sphericity
a
Measure: MEASURE_1 Within Subjects Effect
Mauchly's
W
Approx. Chi-
Square
df
Sig.
Epsilon
b
Greenhouse-
Geisser
Huynh-
Feldt
Lower-bound
type
1.000
.000
0
.
1.000
1.000
1.000
month
.796
.684
2
.710
.831
1.000
.500
type * month
.252
4.135
2
.127
.572
.651
.500
Tests the null hypothesis that the error covariance matrix of the orthonormalized transformed dependent variables is
proportional to an identity matrix.
a. Design: Intercept Within Subjects Design: type + month + type * month
b. May be used to adjust the degrees of freedom for the averaged tests of significance. Corrected tests are displayed in the Tests of Within-Subjects Effects table.
E.
Has the sphericity assumption been satisfied?
Yes because p is > .05
X
2
(2)=4.135, p=.127
6
Tests of Within-Subjects Effects
Measure: MEASURE_1 Source
Type III Sum
of Squares
df
Mean
Square
F
Sig.
Partial Eta
Squared
type
Sphericity Assumed
.133
1
.133
.016
.906
.004
Greenhouse-
Geisser
.133
1.000
.133
.016
.906
.004
Huynh-Feldt
.133
1.000
.133
.016
.906
.004
Lower-bound
.133
1.000
.133
.016
.906
.004
Error(type)
Sphericity Assumed
33.533
4
8.383
Greenhouse-
Geisser
33.533
4.000
8.383
Huynh-Feldt
33.533
4.000
8.383
Lower-bound
33.533
4.000
8.383
month
Sphericity Assumed
2.867
2
1.433
2.774
.122
.410
Greenhouse-
Geisser
2.867
1.661
1.726
2.774
.136
.410
Huynh-Feldt
2.867
2.000
1.433
2.774
.122
.410
Lower-bound
2.867
1.000
2.867
2.774
.171
.410
Error(month)
Sphericity Assumed
4.133
8
.517
Greenhouse-
Geisser
4.133
6.645
.622
Huynh-Feldt
4.133
8.000
.517
Lower-bound
4.133
4.000
1.033
type * month
Sphericity Assumed
64.467
2
32.233
26.135
.000
.867
Greenhouse-
Geisser
64.467
1.144
56.344
26.135
.004
.867
Huynh-Feldt
64.467
1.303
49.479
26.135
.003
.867
Lower-bound
64.467
1.000
64.467
26.135
.007
.867
Error(type*month
)
Sphericity Assumed
9.867
8
1.233
Greenhouse-
Geisser
9.867
4.577
2.156
Huynh-Feldt
9.867
5.212
1.893
Lower-bound
9.867
4.000
2.467
Your preview ends here
Eager to read complete document? Join bartleby learn and gain access to the full version
- Access to all documents
- Unlimited textbook solutions
- 24/7 expert homework help
7
F.
Report the results based on the above table.
There wasn’t a significant effect for either type or month, but there was an interaction effect Type*Month: (F(2)=26.135, p<.001)
Type: (F(1)=.016, p=.906)
Month: (F(2)=2.774,p=.122)
Pairwise Comparisons
Measure: MEASURE_1 month
(I) type
(J) type
Mean Difference
(I-J)
Std. Error
Sig.
b
95% Confidence Interval for
Difference
b
Lower Bound
Upper Bound
1
1
2
-2.600
1.503
.159
-6.774
1.574
2
1
2.600
1.503
.159
-1.574
6.774
2
1
2
-1.200
1.158
.358
-4.414
2.014
2
1
1.200
1.158
.358
-2.014
4.414
3
1
2
4.200
*
.860
.008
1.812
6.588
2
1
-4.200
*
.860
.008
-6.588
-1.812
Based on estimated marginal means
*. The mean difference is significant at the .05 level.
b. Adjustment for multiple comparisons: Least Significant Difference (equivalent to no adjustments).
G.
Report and interpret the post hoc analysis results.
8
Month 3 was significant with Type I & J (p=.008)
9
Exercise 3: Repeated Measures with a Between Group Factor
Open Exercise 3.sav, click Analyze, General Linear Model, Repeated Measures, type time in the within-subject factor name box, type 2 in the number of level box, add, define, move t1 and t2 in the within subjects variables box, move group to the between-subjects factor(s) box, click Plots, move time to the horizontal axis box, add, move time to the separate lines box, continue, click EM Means, move group, time, group*time to the Display means for box, Continue, Options, check homogeneity tests, estimates of effect size, Continue, click paste, after line “/EMMEANS=TABLES(Group*time)” add “compare (Group) ADJ(LSD)”, run the code.
Box's Test of
Equality of
Covariance
Matrices
a
Box's M
2.470
F
.724
df1
3
df2
58320.000
Sig.
.537
Tests the null hypothesis that the observed covariance matrices of the dependent variables are equal across groups.
a. Design: Intercept + Group Within Subjects Design: time
H. For homogeneity of variance covariance matrix assumption, we examine the Box’ M test. It tests the null hypothesis that the observed covariance matrices of the dependent variables are equal across groups. Is the homogeneity of variance covariance matrix assumption violated?
The test was nonsignificant (p=.537) therefore the assumption is not violated
Your preview ends here
Eager to read complete document? Join bartleby learn and gain access to the full version
- Access to all documents
- Unlimited textbook solutions
- 24/7 expert homework help
10
Mauchly's Test of Sphericity
a
Measure: MEASURE_1 Within Subjects Effect
Mauchly's
W
Approx. Chi-
Square
df
Sig.
Epsilon
b
Greenhouse-
Geisser
Huynh-
Feldt
Lower-bound
time
1.000
.000
0
.
1.000
1.000
1.000
Tests the null hypothesis that the error covariance matrix of the orthonormalized transformed dependent variables is
proportional to an identity matrix.
a. Design: Intercept + Group Within Subjects Design: time
b. May be used to adjust the degrees of freedom for the averaged tests of significance. Corrected tests are displayed in the Tests of Within-Subjects Effects table.
I.
Why is the p-value not available?
b/c only two measures
Tests of Within-Subjects Effects
Measure: MEASURE_1 Source
Type III Sum of
Squares
df
Mean Square
F
Sig.
time
Sphericity Assumed
1570.093
1
1570.093
89.014
.000
Greenhouse-Geisser
1570.093
1.000
1570.093
89.014
.000
Huynh-Feldt
1570.093
1.000
1570.093
89.014
.000
Lower-bound
1570.093
1.000
1570.093
89.014
.000
time * Group
Sphericity Assumed
645.696
1
645.696
36.607
.000
Greenhouse-Geisser
645.696
1.000
645.696
36.607
.000
Huynh-Feldt
645.696
1.000
645.696
36.607
.000
Lower-bound
645.696
1.000
645.696
36.607
.000
Error(time)
Sphericity Assumed
317.498
18
17.639
Greenhouse-Geisser
317.498
18.000
17.639
Huynh-Feldt
317.498
18.000
17.639
Lower-bound
317.498
18.000
17.639
11
Tests of Between-Subjects Effects
Measure: MEASURE_1 Transformed Variable: Average Source
Type III Sum of
Squares
df
Mean Square
F
Sig.
Intercept
45910.905
1
45910.905
1405.713
.000
Group
1222.719
1
1222.719
37.438
.000
Error
587.884
18
32.660
J.
Report and interpret the main and interaction effects.
Time has significant main effect (F(1)=89..014, p<.001)
The interaction effects were significant (F(1)=36.607, p<.001)
12
Pairwise Comparisons
Measure: MEASURE_1 time
(I) Group
(J) Group
Mean Difference
(I-J)
Std. Error
Sig.
b
95% Confidence Interval for
Difference
b
Lower Bound
Upper Bound
1
.00
1.00
-3.022
2.146
.176
-7.531
1.487
1.00
.00
3.022
2.146
.176
-1.487
7.531
2
.00
1.00
-19.093
*
2.335
.000
-23.999
-14.187
1.00
.00
19.093
*
2.335
.000
14.187
23.999
Based on estimated marginal means
*. The mean difference is significant at the .05 level.
b. Adjustment for multiple comparisons: Least Significant Difference (equivalent to no adjustments).
K.
Report and interpret the post hoc analysis results.
Time point 2 was significant (p<.001)
Your preview ends here
Eager to read complete document? Join bartleby learn and gain access to the full version
- Access to all documents
- Unlimited textbook solutions
- 24/7 expert homework help
Related Documents
Related Questions
Can you please help with 4.30 sulfur, the ocean and the sun? Only part A which is make a scatter plot that shows how DMS responds to SRD.
arrow_forward
The ols() method in statsmodels module is used to fit a multiple regression model using “Quality” as the response variable and “Speed” and “Angle” as the predictor variables. The output is shown below. A text version is available. What is the correct regression equation based on this output? What is the coefficient of determination? Select one.
arrow_forward
Which of the variables is the indepenent variable and dependent variable for the following question.
fit a simple linear regression model to predict latitudes using average monthly range
lat= latitudes
range= the average monthly range between mean montly maximum and minimum temperatures for a selected set of US cities.
arrow_forward
Consider the accompanying data set of dependent and independent variables
a. Perform a general stopwise regression using a 0.05 for the p-value to enter and to remove independent variables from the regression model
b. Perform a residual analysis for the model developed in part a to verify that the regression conditions are met
Click the icon to view the data
a. Use technology to perform the general stepwise regression What is the resulting regression equation? Note that the coefficient is 0 for any variable that was removed or not significant
-0.69 (050), (050)+(018)
- X
(Round to two decimal places as needed)
•
Data Table:
y
63
43
51
49
40
42
23
37
30
27
20
31
FR
74
63
78
3534
52
44
47
35
17
15
20
17
Print
X₂
21
259.
15
9
38
18
17
5
40
27
30
33
x₂
22
aadosa 2NNG
29
20
17
13
17
8
15
10
10
Done
1
arrow_forward
please do not provide solution in image format thank you!
arrow_forward
Hello there, can you please help me out with this problem? Write clearly and thank you!
arrow_forward
I couldn't include the options for the graphs because it only allows me to add two pictures.
arrow_forward
Using concept of regression, prove that the efficiency ratio could be predicted by tank temperature. Refer to the image below.
Note:please show how to calculate manually without using excel. Thanks
arrow_forward
The estimated regression equation for a model involving two independent variables and 10 observations follows.
Y=25.7067 + 0.2795x1 + 0.7337x2
A. Interpret b1 and b2 in this estimated trgression equation.
B1 = ?
B2 = ?
Thank you
arrow_forward
Given the relatively small sample size, we can characterize the left-hand plot as a random cloud of points in a rough horizontal band and the right-hand plot as points that lie reasonably close to the 45° line. Briefly describe what this tells us about the simple linear regression model assumptions in this case.
The largest absolute standardized residual for this simple linear regression analysis is 1.298, while the highest leverage is 0.430. Briefly describe what this tells us about potentially influential observations in this analysis.
arrow_forward
please include how I would solve in desmos if possible thank you!
A regression was run to determine if there is a relationship between hours of study per week (xx) and the final exam scores (yy).The results of the regression were:
y=ax+b a=5.491 b=37.79 r2=0.806404 r=0.898
Use this to predict the final exam score of a student who studies 7.5 hours per week, and please round your answer to a whole number.
arrow_forward
SEE MORE QUESTIONS
Recommended textbooks for you
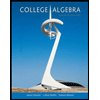
College Algebra
Algebra
ISBN:9781305115545
Author:James Stewart, Lothar Redlin, Saleem Watson
Publisher:Cengage Learning
Algebra & Trigonometry with Analytic Geometry
Algebra
ISBN:9781133382119
Author:Swokowski
Publisher:Cengage
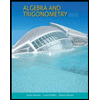
Algebra and Trigonometry (MindTap Course List)
Algebra
ISBN:9781305071742
Author:James Stewart, Lothar Redlin, Saleem Watson
Publisher:Cengage Learning
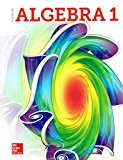
Glencoe Algebra 1, Student Edition, 9780079039897...
Algebra
ISBN:9780079039897
Author:Carter
Publisher:McGraw Hill
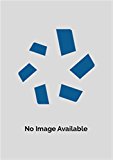
Big Ideas Math A Bridge To Success Algebra 1: Stu...
Algebra
ISBN:9781680331141
Author:HOUGHTON MIFFLIN HARCOURT
Publisher:Houghton Mifflin Harcourt
Related Questions
- Can you please help with 4.30 sulfur, the ocean and the sun? Only part A which is make a scatter plot that shows how DMS responds to SRD.arrow_forwardThe ols() method in statsmodels module is used to fit a multiple regression model using “Quality” as the response variable and “Speed” and “Angle” as the predictor variables. The output is shown below. A text version is available. What is the correct regression equation based on this output? What is the coefficient of determination? Select one.arrow_forwardWhich of the variables is the indepenent variable and dependent variable for the following question. fit a simple linear regression model to predict latitudes using average monthly range lat= latitudes range= the average monthly range between mean montly maximum and minimum temperatures for a selected set of US cities.arrow_forward
- Consider the accompanying data set of dependent and independent variables a. Perform a general stopwise regression using a 0.05 for the p-value to enter and to remove independent variables from the regression model b. Perform a residual analysis for the model developed in part a to verify that the regression conditions are met Click the icon to view the data a. Use technology to perform the general stepwise regression What is the resulting regression equation? Note that the coefficient is 0 for any variable that was removed or not significant -0.69 (050), (050)+(018) - X (Round to two decimal places as needed) • Data Table: y 63 43 51 49 40 42 23 37 30 27 20 31 FR 74 63 78 3534 52 44 47 35 17 15 20 17 Print X₂ 21 259. 15 9 38 18 17 5 40 27 30 33 x₂ 22 aadosa 2NNG 29 20 17 13 17 8 15 10 10 Done 1arrow_forwardplease do not provide solution in image format thank you!arrow_forwardHello there, can you please help me out with this problem? Write clearly and thank you!arrow_forward
- I couldn't include the options for the graphs because it only allows me to add two pictures.arrow_forwardUsing concept of regression, prove that the efficiency ratio could be predicted by tank temperature. Refer to the image below. Note:please show how to calculate manually without using excel. Thanksarrow_forwardThe estimated regression equation for a model involving two independent variables and 10 observations follows. Y=25.7067 + 0.2795x1 + 0.7337x2 A. Interpret b1 and b2 in this estimated trgression equation. B1 = ? B2 = ? Thank youarrow_forward
- Given the relatively small sample size, we can characterize the left-hand plot as a random cloud of points in a rough horizontal band and the right-hand plot as points that lie reasonably close to the 45° line. Briefly describe what this tells us about the simple linear regression model assumptions in this case. The largest absolute standardized residual for this simple linear regression analysis is 1.298, while the highest leverage is 0.430. Briefly describe what this tells us about potentially influential observations in this analysis.arrow_forwardplease include how I would solve in desmos if possible thank you! A regression was run to determine if there is a relationship between hours of study per week (xx) and the final exam scores (yy).The results of the regression were: y=ax+b a=5.491 b=37.79 r2=0.806404 r=0.898 Use this to predict the final exam score of a student who studies 7.5 hours per week, and please round your answer to a whole number.arrow_forward
arrow_back_ios
arrow_forward_ios
Recommended textbooks for you
- College AlgebraAlgebraISBN:9781305115545Author:James Stewart, Lothar Redlin, Saleem WatsonPublisher:Cengage LearningAlgebra & Trigonometry with Analytic GeometryAlgebraISBN:9781133382119Author:SwokowskiPublisher:CengageAlgebra and Trigonometry (MindTap Course List)AlgebraISBN:9781305071742Author:James Stewart, Lothar Redlin, Saleem WatsonPublisher:Cengage Learning
- Glencoe Algebra 1, Student Edition, 9780079039897...AlgebraISBN:9780079039897Author:CarterPublisher:McGraw HillBig Ideas Math A Bridge To Success Algebra 1: Stu...AlgebraISBN:9781680331141Author:HOUGHTON MIFFLIN HARCOURTPublisher:Houghton Mifflin Harcourt
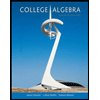
College Algebra
Algebra
ISBN:9781305115545
Author:James Stewart, Lothar Redlin, Saleem Watson
Publisher:Cengage Learning
Algebra & Trigonometry with Analytic Geometry
Algebra
ISBN:9781133382119
Author:Swokowski
Publisher:Cengage
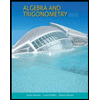
Algebra and Trigonometry (MindTap Course List)
Algebra
ISBN:9781305071742
Author:James Stewart, Lothar Redlin, Saleem Watson
Publisher:Cengage Learning
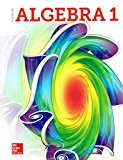
Glencoe Algebra 1, Student Edition, 9780079039897...
Algebra
ISBN:9780079039897
Author:Carter
Publisher:McGraw Hill
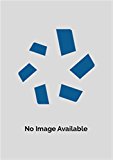
Big Ideas Math A Bridge To Success Algebra 1: Stu...
Algebra
ISBN:9781680331141
Author:HOUGHTON MIFFLIN HARCOURT
Publisher:Houghton Mifflin Harcourt