Rhodes_Jaylen_Week2Assignment
pdf
keyboard_arrow_up
School
University of Louisville *
*We aren’t endorsed by this school
Course
632
Subject
Statistics
Date
Apr 3, 2024
Type
Pages
4
Uploaded by CountKnowledgeArmadillo48
Jaylen Rhodes
Week 2 Assignment
EM 632
1.
Find the P-value of the test statistic Z0 = 2.50 for the following hypothesis where
variance is known. H0: μ =100 and H1: μ > 100.
Z0 = 2.50 using the table, that is approximately 0.9938
P(1-Z0) = 1 - P(Z0) = 1 - 0.9938 = 0.0062
2.
What does it mean to have a process that is considered to be in statistical
control?
A process that is considered to be in statistical control is one where the
variation in its output is consistent, predictable, and stable over time.
3.
Referencing Equation 5.1 in the text and in the Chapter 5 Slides, how will a
control chart respond if larger values of L are selected? What if smaller values of
L are selected?
Higher values of L allows for more variation at a given value of σw before
process is stopped to search for assignable cause whereas smaller values
of L allows for less variation at a given value of σw.
4.
When sampling subgroups from a process, should the subgroup size and
sampling frequency be such that variation due to assignable cause is found
within subgroups or between them? Why?
When sampling subgroups from a process in statistical process control
(SPC), it's essential to design the subgroup size and sampling frequency to
capture variation due to assignable causes within subgroups rather than
between them. This is because it allows for detecting issues earlier,
stablizing processes and minimizing impact of external factors and lastly, it
allows for better decision making.
5.
If the time order of production has not been recorded in a set of data from a
process, is it possible to detect the presence of assignable causes? Why or why
not?
If the time order of production isn't recorded in a dataset, it's hard to detect
assignable causes, which are specific reasons for variations in the
process. This is because there’s no timeline meaning we can’t determine if
variations happen at random or not for example. It’s hard to compare to the
Normal or the baseline, its generally just hard to find causes as well.
6.
A machine is used to fill containers with a liquid product. Fill volume can be
assumed to benormally distributed. A random set of ten containers is selected
and the net contents (oz)are measured. See data presented in #6 tab of the
Excel file.
a.
Suppose the manufacturer wants to be sure that the net contents mean
exceeds 12oz. What conclusions can be drawn from the data (use α =
0.01)?
Using a one-sample t-test given sample mean is 12.03, population mean
is 12, sample standard deviation is 0.03162 and sample size is 10 with
significance level of 0.01 and 9 degrees of freedom which gives critical
value 2.8214:
H_0: μ ≤ 12 (Mean fill volume is less than or equal to 12 oz).
H_1: μ > 12 (Mean fill volume exceeds 12 oz).
t would come out to be 4.7428 and since this is larger than the
critical value of 2.8214, we reject the null hypothesis and this means
we can conclude that there is sufficient evidence to suggest that the
net content mean exceeds 12 oz at the 0.01 significance level.
b.
Construct a 95% two-sided confidence interval on the mean fill volume.
Well using minitab and the t-table for 95% confidence and 9 degrees of
freedom, we get a critical value of 2.262. The confidence interval becomes
x_bar
∓
t_crit * (s / sqrt(n)) = 12.03
∓
2.262 * (0.03162 / sqrt(10))
The 95% confidence interval is then (11.99612, 12.06388) ounces.
c.
Construct a normal probability plot. Does the assumption of normality
seem appropriate for the fill volume data?
The assumption of normality does seem appropriate for the fill volume data as it
forms a straight line in the normal probability plot meaning the data is normally
distributed.
7.
Two processes are used to produce forgings used in an aircraft wing assembly.
Of 200 forgings selected from process 1, 10 do not conform to the strength
specifications, whereas of 300 forgings selected from process 2, 20 are
nonconforming.
a.
Estimate the fraction nonconforming for each process.
Process 1:
n_1 = 200, x_1 = 10 so
p_hat_1 = x_1 / n_1 = 10 / 200 = 0.05
Process 2:
n_2 = 300, x_2 = 20 so
p_hat_2 = x_2 / n_2 = 20 / 300 = 0.0667
b.
Test the hypothesis that the two processes have identical fractions
nonconforming(α = 0.05).
p_hat = x_1 + x_2 / n_1 + n_2 = 10 + 20 / 200 + 300 = 30 / 500 = 0.06
H_0: The fractions nonconforming for the two processes are equal.
H_1: The fractions nonconforming for the two processes are not equal.
z = (p_hat_1 - p_hat_2) / sqrt(p_hat(1-p_hat)(1 /n_1 + 1/n_2))
z = (0.05 - 0.0667) / sqrt(0.06(1-0.06)(1/200 + 1/300))
z = -0.0167 / sqrt(0.06(0.94)(1/200 + 1/300))
z = -0.0167 / sqrt(0.06(0.94)(1/120))
z = -0.0167 / sqrt(0.00049902) = -0.7496
The critical value for a two-tailed test with α = 0.05 is 1.96. Since the
absolute value of the test statistic -0.7496 < 1.96, we fail to reject the
null hypothesis. This means the fractions nonconforming for the two
processes are equal.
c.
Construct a 90% confidence interval on the difference in fraction
nonconforming between the two processes.
z_α/2 = 1.645
(p_hat_1 - p_hat_2)
∓
z_α/2 * sqrt((p_hat_1(1 - p_hat_1)/n_1) +
(p_hat_2(1 - p_hat_2)/n_2))
(0.05 - 0.0667)
∓
1.645 * sqrt((0.05(1-0.05)/200) + (0.0667(1-0.0667)/300))
-0.0167
∓
1.645 * sqrt(0.0004452667)
-0.0167
∓
1.645 * 0.0211
-0.0167
∓
0.0347
The 90% confidence interval is then (-0.0514, 0.0180) forgings.
Your preview ends here
Eager to read complete document? Join bartleby learn and gain access to the full version
- Access to all documents
- Unlimited textbook solutions
- 24/7 expert homework help
8.
Suppose we wish to test the hypotheses of H0: μ = 15 and H1: μ ≠ 15 where we
know that σ2 = 9.0. If the true mean is really 20, what sample size must be used
to ensure theprobability of type II error is no greater than 0.10? Assume that α =
0.05.
Given, σ2 = 9.0, σ = 3.0, H0: μ0 = 15, H1: μ1 = 20, α = 0.05, and β ≤ 0.10
We need to find sample size n such that 1 - β is at least 1 - 0.10 = 0.9
1 - β = 0.9, with z_α/2 = 1.96 gives us a z value of 1.28
z = (μ1 - μ0) / (σ / sqrt(n))
1.28 = (20 - 15) / (3 / sqrt(n)) = 5 / (3*sqrt(n)) = 5*sqrt(n) / 3
1.28 = 5 * sqrt(n) / 3
sqrt(n) = (1.28 * 3) / 5
sqrt(n) = 0.768
n = 0.768^2 = 0.590
Since the sample size n must be a positive integer, we round up to ensure the
Type II error is no greater than 0.10.
Therefore, the sample size n required to ensure the probability of Type II
error is no greater than 0.10 is 1.
Related Documents
Recommended textbooks for you
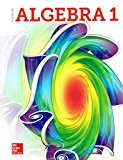
Glencoe Algebra 1, Student Edition, 9780079039897...
Algebra
ISBN:9780079039897
Author:Carter
Publisher:McGraw Hill
Recommended textbooks for you
- Glencoe Algebra 1, Student Edition, 9780079039897...AlgebraISBN:9780079039897Author:CarterPublisher:McGraw Hill
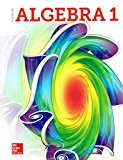
Glencoe Algebra 1, Student Edition, 9780079039897...
Algebra
ISBN:9780079039897
Author:Carter
Publisher:McGraw Hill