pdf
keyboard_arrow_up
School
University of California, Los Angeles *
*We aren’t endorsed by this school
Course
LS7B
Subject
Statistics
Date
Feb 20, 2024
Type
Pages
24
Uploaded by CoachNeutronKouprey66
Table of Contents
1. Objectives
2. Introduction
3. Emergence of Scientific Methodology
4. Statistics
5. Descriptive Statistics
6. Inferential Statistics
7. The Memory Interference Test (MIT)
8. Appendix: An Example of Comparing Two Groups Using Resampling
1. Objectives
To introduce and use the scientific method.
To introduce and practice using simple statistics.
To learn how to write scientific reports.
2. Introduction
Science is a practice of gaining knowledge of nature. In order to do so, a series of methods are
designed to gather, analyze, and interpret the information about nature. These methods have not
always been the same through time. Even in modern days, different practices are found in different
disciplines by different scientists. Although it may be difficult to have all of those who practice science
to agree on one single method based on which scientific knowledge is obtained, there are still a few
common characteristics in their methods that are generally agreed on by those who are in the
practice. In this lab, you are going to learn a few techniques used by many scientists who follow them
to learn about nature.
Lab Manual - Lab A - Scientific Method and the Memory Interference
Test (MIT)
Return to top
Return to top
3. Emergence of Scientific Methodology
Modern methodology to pursue science was established in the seventeenth century in Western
Europe. About four hundred years ago a new experimental method of investigation into the natural
world emerged. The major players in this revolutionary change in thinking and practice included
Francis Bacon (1561- 1626) and Rene Descartes (1596-1650). Since then much of the scientific
methodology has been modified. Today there are two important emphases in practicing science: (1)
the hypothetico-deductive approach and (2) the falsificationist procedure.
The hypothetico-deductive approach (
Figures A.1 & A.2
)
: The hypothetico-deductive approach is a
series of steps that, as long as none of the steps is flawed, leads to a robust conclusion about a
particular problem. It begins with observations of events or patterns, followed by suggestions for the
general causes and nature of the observed events and patterns. However, without further testing of
the model, inaccuracies would render the suggestions unreliable. Consequently, after the initial
observations of and reasoning about the general nature of observed phenomena, a scientific method
demands that a hypothetico-deductive approach be employed. The hypothetico-deductive approach
,
proposed by Karl Popper (1902-1994), an influential science philosopher, requires a specific
hypothesis
(H1), i.e., a prediction of an effect or a difference, to be constructed to explain a particular
aspect of the observed phenomenon. Furthermore, this hypothesis must be tested, either by carrying
out appropriate experiments or making specific observations. Only after the results of these
experiments have been measured and tested statistically
can we determine whether the hypothesis
(prediction) is or is not supported by the data and, therefore, deduce
something about the
phenomenon.
Return to top
Figure A.1. A scientific method that incorporates the hypothetico-deductive approach and
falsificationist procedure.
If the hypothesis was supported, something positive is now known about that phenomenon and other
aspects can be examined by constructing and testing other hypotheses. If the hypothesis was
rejected, something else is known about that phenomenon, albeit something negative. At the same
time other hypotheses should also be constructed and tested. As you can see, via the hypothetico-
deductive approach, it is possible to go on learning about things forever. Consequently, there is
always the possibility that a new hypothesis and test will show a previous piece of "knowledge" to be
false. This self-correcting mechanism is an important aspect of the scientific method.
The falsificationist procedure
: The falsificationist procedure is a simple way of increasing the power of
conclusions deduced using the hypothetico-deductive approach. It merely involves taking the
prediction (hypothesis) of an effect (H1 above) and creating a null hypothesis
. For the purpose of this
course, we will state that a null hypothesis (H0) predicts no effect or no difference between two or
more tested samples. The reason for doing this is that hypotheses can be disproved much more
easily than they can be proved.
When we are formulating statistically testable hypotheses, they need to meet certain criteria. A good
hypothesis is one that is both specific and testable.
Specific:
Your preview ends here
Eager to read complete document? Join bartleby learn and gain access to the full version
- Access to all documents
- Unlimited textbook solutions
- 24/7 expert homework help
What groups are being compared?
What measure is being used to compare them?
Testable:
Will you be able to reject/retain your null hypothesis after conducting the experiment?
In the lab section this week, you will participate in an activity where you look at a series of statistical
hypotheses and evaluate them.
Figure A.2. Why is scientific writing so critical?
4. Statistics
As stated previously, it is almost never feasible to make all of the possible measurements that might
prove a hypothesis. In addition, in natural populations, there often is considerable variation (consider
the human species). Instead we take measurements from some individuals in a population (a
Return to top
sample) and use those measurements to draw conclusions about the larger population using
statistical methods.
Statistics are often divided into two types: descriptive and inferential statistics. Descriptive statistics
(e.g., mean and median) describe the pattern (i.e., distribution
) in observed groups of measurements
(i.e., samples
). Inferential statistics, in contrast, can be used to draw conclusions about the whole
population(s) based on the smaller sample datasets, including testing hypotheses. For example, in
this lab, we’ll be comparing two groups and testing the null hypothesis that there is no difference
between them.
Brief descriptions are provided below to help you to understand these statistics. However, for LS23L,
you are not required to memorize the formulas.
Definitions
Several definitions will help you to understand how statistics are calculated, how they relate to your
measurements, and what they really mean.
Population:
the entire collection of measurements on which the researcher intends to draw
conclusions (e.g., adult weight of human population in South America, or height of eucalyptus trees in
Los Angeles County).
Sample:
the set of measurements (X
, X
, X
, … X ) actually made (e.g., sampling daily dietary
calories of one thousand individuals from each capital of a South American country; or sampling
height of fifty eucalyptus trees in each LA neighborhood).
5. Descriptive Statistics
There are a few terms in statistics commonly used to describe the set of measurements in order to
show their characteristics. These terms, called parameters, can show the central tendency or can be
described as a measure of variability. However, due to the fact that it is impossible to obtain all the
measurements of one particular variable, the true parameter is usually not available. As a result, an
estimate of a parameter is produced to serve as a description of these measurements. An estimate of
a parameter is called a statistic. The following explains three statistics that measure the central
tendency and two statistics that describe the level of variability of a set of measurements. We are
going to incorporate these statistics into the lab report.
Mean
1
2
3
i
Return to top
One of the statistics that measures the central tendency of a variable is the mean. The mean is more
commonly known as the “arithmetic average.” The mean
of a sample (X
̄
) is calculated as the sum of
all measurements in the sample divided by the sample size (
n
). However, the mean is only a good
estimate of the central tendency of a set of data if the data’s distribution is bell-shaped (symmetric
single-humped with thin tails).
Mean = X
̄
= (X
+X
+X
+...+X ) / n = ∑X
/ n
When is it OK to use the mean?
Rule of Thumb
When is it OK to use the mean to describe a data set? We can set out a rule of thumb. Let’s say that
a distribution is “bell-shaped” if it is:
symmetric
single
humped
thin tailed
Then our rule of thumb is that when a distribution is bell-shaped, the use of the mean value to
describe the data is OK.
Median
The median is a measure of central tendency that works even if the data doesn’t fit these
requirements, so it is often a better measure than the mean. The median is the measurement located
at the middle of the ordered set of data. In other words, there are just as many observations larger
than the median as there are smaller. If the sample size is odd, the median is the middle
measurement of the ordered series. If the sample size is even, the median is the average between
the two middle measurements. For example,
Series A:
1.5, 3.7, 3.9, 4.5, 6.3, 7.1, 8.0, 8.8, 9.4
Series B:
1.5, 3.7, 3.9, 4.5, 6.3, 7.1, 8.0, 8.8, 9.4, 10.5
The median for Series A is 6.3 and the median for Series B is (6.3 + 7.1) ÷ 2 = 6.7
1
2
3
i
i
Your preview ends here
Eager to read complete document? Join bartleby learn and gain access to the full version
- Access to all documents
- Unlimited textbook solutions
- 24/7 expert homework help
Mode
The mode is defined as the measurement of relatively great concentrations in a set of data. For
example,
Series C:
3, 4, 4, 4, 4, 5, 5, 6, 8, 9
Series D:
4, 5, 6, 6, 6, 6, 7, 8, 9, 10, 10, 10, 10, 10, 11, 12
In Series C, data concentrate at the value 4, thus the mode is 4. In Series D, there are two modes
(hence "bimodal"): 6 and 10, respectively. For a symmetrical unimodal distribution (e.g., bell-shaped),
the mean, median, and mode will be close to each other. These measures of central tendency will
have different values if the data is not bell-shaped (e.g., bimodal or skewed).
Standard Deviation
One of the best-known measures of variability is the standard deviation, which is the square root of
the average deviation of each value from the mean. However, if the data is not bell-shaped, both the
mean and the standard deviation have limited utility since they do not accurately represent the
dataset.
Median Absolute Deviation
A better measure of variability or spread is the Median Absolute Deviation (MAD), which uses the
concepts of absolute value and median rather than squaring and mean and thus works for all data
without any requirements.
MAD of data set {
X
, X
, . . ., X
}
= median { |X
- m|, |X
- m|, . . .,|X
- m|
} where m is
the median of the set {X
, X
, . . ., X
}.
For example, let’s find the MAD of series A. Series A:
1.5, 3.7, 3.9, 4.5, 6.3, 7.1, 8.0, 8.8, 9.4
We found above that the median of Series A is 6.3. The absolute value of the difference between
each value and the median (i.e., distance) is 4.8, 2.6, 2.4, 1.8, 0, 0.8, 1.7, 2.5, 3.1. The median of
these distances is 2.4, so the MAD is 2.4.
6. Inferential Statistics
1
2
n
1 2 n
1
2
n
Return to top
So far, we have only discussed a few statistics to describe a group of data. However, the essence of
a statistical analysis is to answer a question objectively by conducting a statistical test. A statistical
test is made between two or more sets of samples in order to compare, for example, if they are from
the same population. In this lab, we are only going to explore one of the commonly used statistical
tests. You are not expected to become an expert on statistics, since it takes much more than one
course to master this discipline. The purpose of this lab is to introduce you to these objective
methods modern scientists use to answer their questions.
Comparing Two Groups Using Resampling
Quite often a scientific study relies on a comparison between two or more sample groups. In order to
talk about differences (or lack of differences) between these groups in a meaningful way, it is
necessary to have a measurement that all scientists recognize and understand - this is where
statistical tests come in handy. Many statistical tests have been developed to allow scientists to
calculate the significance of the differences they see in their data. In this experiment, we will be
comparing the two groups using simulations based on the null hypothesis that resample the original
data (sometimes called bootstrapping). This method works for data with a distribution of any shape,
unlike the better known t-test.
Your preview ends here
Eager to read complete document? Join bartleby learn and gain access to the full version
- Access to all documents
- Unlimited textbook solutions
- 24/7 expert homework help
Figure A.3 (Adopted from LS40).
The Big Box method to determine the statistical significance of the
observed effect size between two groups A and B using resampling.
In this method, we combine the two groups of collected measurements to create a theoretical
population (“Big Box” based on the null hypothesis which states that both sets of data belong to the
same population see Figure A.3
) and then employ computer simulations to randomly draw new
datasets, thus “resampling” the original data. We can then calculate the fraction of thousands of
simulations which are as extreme or more extreme than our observed dataset.
The outcome of this calculation is the well-known p-value: the probability of a result which are as
extreme or more extreme as our observed result happening purely by chance if the null hypothesis is
true. Note that we’re saying “as or more extreme”, not “higher”, so we are counting values on both
sides of our distribution. This is called a two-tailed p-value, which we should calculate if it is
mathematically possible.
Luckily for you, we will provide a computer program that conducts these simulations and calculates
the p-value for you, so you will not be required to do the calculations by hand for this experiment.
Statistical Significance
The p-value is also called the “degree of statistical significance” of your result. By conventional
standards, a p-value of 0.01 or below is considered statistically significant, so we can reject our null
hypothesis, support our experimental hypothesis, and state that our groups are statistically
significantly different.
As you read other research papers please be aware that you may see different cut offs used - for a
long time the standard threshold for significance was often set at 0.05. However, most scientists are
now recommending that we use a more stringent threshold of 0.01 that results in fewer false
positives.
When you start working on your first scientific writing assignment you will apply all this information.
You will first formulate a specific and testable hypothesis and a null hypothesis. Once you select the
applicable parameters in the web interface, the p-value will be automatically calculated. If your p-
value is greater than 1% (0.01), you will retain the null hypothesis and conclude that the two groups
are not significantly different. If, however, the p-value is less than 1%, you will conclude that the two
samples are significantly different.
To learn more about statistics, including simulations, we recommend taking LS 40: Statistics of
Biological Systems and/or reading its textbook Understanding Data: an Experimental Approach to
Statistics
, by Alan Garfinkel and Yina Guo.
Key points to understand for your lab section this week
Statistical hypotheses are formulated in pairs – the hypothesis
predicts a difference
while the
null hypothesis
assumes no difference
.
A statistical hypothesis should be both specific and testable.
We use statistical tests to determine if two groups are statistically different from each other by
calculating the p-value
using resampling.
The p-value is the probability of a result as or more extreme as our observed result happening
purely by chance if the null hypothesis is true.
If the p-value is smaller
than 0.01
, the null hypothesis is rejected
(because there is a very low
probability that the difference is due to chance).
If the p-value is larger
than 0.01, then we fail to reject
the null hypothesis (because there is a
greater probability that the difference is due to chance).
7. The Memory Interference Test (MIT)
Gathering Data for your Scientific Writing Assignment
In your first scientific writing assignment, you will have a chance to apply some of the scientific and
statistical concepts you have just learned about while participating in an actual ongoing research
project. You will be expected to follow the hypothetico-deductive approach by formulating your own
hypothesis and null hypothesis. You will then compare the two groups by resampling the data and
calculate a p-value to determine whether or not your sample groups are significantly different from
each other. You are participating in real research and contributing actual data for possible future
publication.
The current project proposes to assess cognitive functioning of undergraduate students through
computerized measures developed by a neuropsychologist. The Memory Interference Test (MIT) is a
computer program that uses either visual or auditory cues to test the subject's memory. In addition, a
demographic survey asks questions about the subject's mental and physical states at the time of the
test, along with information about his or her age, education level, and background. Subjects can
choose not to answer any questions that make them uncomfortable, and all data remain completely
anonymous. Responses will be sent automatically and electronically to an aggregated database-
specific scores and background data will not be available to anyone. For research purposes,
demographic information about a subgroup will be accessible only if that group is larger than 50. This
restriction protects students' anonymity, while ensuring good research design with an adequate group
size.
Return to top
The MIT has several cognitive measures. Prior to taking any form of the test, subjects are presented
with a pre-test that allows them to adjust to the format of the test. A series of ten items flashes on the
screen. The item type (either a word or image) varies depending on the test stimulus type chosen.
Participants identify the item using the arrow keys. Once the participant has completed the pre-test
they will begin the memory recall test. The picture memory tests [pictures, faces, designs, and kanji]
flash images onto the screen, while the word memory test flashes written words. In the auditory test,
the subject wears headphones and listens to lists of words with no visual cues. Each version of the
MIT consists of four memory tests and a reaction time test: Tests 1, 2,
and 3
are identical. Each
presents a target list of twenty items and then a recognition list of fifty items. The recognition list
consists of the twenty target items randomly interspersed among thirty additional items (referred to as
distractors). The subject identifies which items they recognize from the previously presented target
list. Test 4
presents an additional recognition list of sixty items, consisting of ten items from each of
the target lists of Tests 1, 2, and 3, together with thirty distractors. The subject is asked to identify
which of the items in the recognition list appeared in the three previously presented target lists. Test
5
is a test of reaction times only, independent of any memory effects. It presents a group of fifty
items, consisting of twenty squares and thirty circles. The subject is required to identify which items
are squares and which are circles, and the computer records his or her reaction time on each
identification. Regardless of which type of test is taken the subject is exposed to the same three lists
of items in the same order. In addition to recording right and wrong answers, the program measures
reaction time. The computerized test takes approximately fifteen minutes to complete, and an
additional five minutes to fill out the demographic survey.
Please keep in mind that the MIT is not a measure of intelligence or education. It simply tests the
subject's memory at a particular point in time. Results can vary widely, depending on many factors
including sleep, stress, time of day, etc. This variability is one of the most interesting aspects of the
test, and it is what allows students to formulate and test research hypotheses.
Once you have had the option of taking the MIT, you can begin to think about a factor you would like
to test. For example, do people recall pictures and words differently? Take a look at the many
independent variables available in the database to get an idea of what you might like to test. Think
about a factor in which you are genuinely curious - you will be expected to write an individual lab
report on this topic, and it is much easier to write about something that interests you.
MIT Manual
This manual will guide you through the web interface for comparing two groups using the aggregated
database. To begin, we'll walk you through the process of defining the data, specifically focusing on
the dependent variables
that can be extracted from the aggregated database
. You will get a
chance to practice this process during lab in week 1.
Your preview ends here
Eager to read complete document? Join bartleby learn and gain access to the full version
- Access to all documents
- Unlimited textbook solutions
- 24/7 expert homework help
When it comes to evaluating test performance, there are two distinct dependent variables
available
for comparing the two groups:
1. Number of correct responses:
the accuracy with which a subject recalls the items.
2. Average response time:
the speed at which a subject, on average, responds to the correct
items.
Please note that these are two separate measures, and you will need to choose which one you want
to use for your experiment - you will not be writing about both of them. Whichever variable you pick,
the website will provide the relevant statistics and calculate the associated probability value (p-value)
through resampling simulations.
The Web Interface (
http://ls23l.lscore.ucla.edu/MIT
(http://ls23l.lscore.ucla.edu/MIT) )
Within the web interface, you'll find a set of interactive elements, including a prominent top-bar for
selecting the "
Test Stimulus Type
" (the example below shows ‘Pictures’ highlighted in red).
Additionally, the blue section labeled "
Questionnaire Lists
" allows you to choose from eight distinct
questionnaire lists (see Figure A.4
).
Each of these eight options presents a selection of independent variables derived from the initial MIT
test questionnaire. These independent variables serve as filters, enabling you to narrow down and
select specific groups from the entire pool of test participants. A list of these questions can be found
in Table 1 at the end of this section.
Figure A.4.
If you want to compare between two different test formats (such as pictures and words) you can
select the "
Compare
" button located on the top-left of the interface. Alternatively, you have the option
to look at a single test format and filter participants based on their demographic responses. We’ll
show you an example of each of these types of comparisons below, starting with comparing two
different test types.
How to Compare Different Test Stimulus Types
You may want to explore whether reaction times differ between different test stimulus types; for
example, do subjects remember pictures differently than words? How does auditory memory
compare to reading memory in terms of speed? In this scenario, you would formulate a hypothesis,
such as "Subjects have a different recall accuracy for pictures than words," or "Subjects identify
pictures at a different speed than they do words." Correspondingly, you would establish a null
hypothesis, like "Subjects remember pictures and words equally well," or "Subjects identify pictures
and words at the same rate."
First select the 'Compare' button (
Figure A.4
), positioned in the top bar. To compare all data from the
picture versus the all the word memory tests in the database, you would then select these two test
stimulus types from the top bar. "Picture" is the default setting, so you would only need to choose
"Words" for the second set (
Figure A.5a
). Now you will be able to compare the results from all
subjects who took the picture test with all subjects who took the word test.
Figure A.5a. Control Window
The results of the comparison between all data from the picture test (PMIT) and all data from the
word test (WMIT) are depicted in Figure A.5b
(please note that due to ongoing data collection, the
numbers may vary).
Figure A.5b. Result Panel
The web interface provides two panels, each corresponding to a dependent variable. As you recall,
we have two separate variables to choose from - accuracy and speed. On the left (in the purple
panel) are the statistics for the "
# of Correct Responses
," while on the right (in the blue panel) you'll
find the statistics for the "
Average Response Time
." In the lower section of each panel, there is a
graph displaying an overlay of two histograms, representing the data from each group (Stimulus
Types: Picture and Word). The bars in red illustrate the data for independent variable group A of each
experiment (Stimulus Type: Pictures), while green bars represent independent variable group B of
each experiment (Stimulus Type: Word). The respective effect sizes
(the absolute difference
between the medians of the two compared groups) are displayed in each panel's top-right corner. To
calculate the p-value, you'll need to run a simulation using the resampling method. To run the
simulation, simply click on the gray button labeled in red as '
Calculate p-value
' (
Figure A.5b
).
Figure A.5c.
Once the button is pushed the simulation will start (
Figure A.5c
) and might take a few seconds to
finish, depending on the sample size.
Figure A.5d.
In Figure A.5d
, both experiments have been assessed. The two new graphs at the center in the
lower panel illustrate crucial metrics: A histogram of the simulated effect sizes
, the observed effect
size
and the p-value
. Considering these results, would you deem them statistically significant?
Would you choose to either reject or fail to reject your null hypothesis?
Your preview ends here
Eager to read complete document? Join bartleby learn and gain access to the full version
- Access to all documents
- Unlimited textbook solutions
- 24/7 expert homework help
The Simulated Effect Size Histogram
Every p-value calculation utilizing the resampling method yields a graph similar to the one depicted in
Figure A.5e
. In this specific instance, the p-value is calculated to be 0.7004 indicating that 70,04% of
the 10,000 randomly selected group pairs exhibited absolute effect sizes equal to or greater than the
observed effect size of 2.
Figure A.5e.
Histogram of results of 10,000 simulations using the resampling method
Values equal or greater than 2 are represented by the red bars in the histogram. This indicates that
results as extreme as 2 happen under the Null Hypothesis at 70.04% of the time. Note that we use
the absolute effect size so the red bars are seen both above and below the center of the histogram
and a difference in either direction is included in the calculation of the p-value. When performing this
type of analysis you cannot specify better
or worse
in your hypothesis, just different
.
Important note for your writing assignment
: Once you have actually performed your experiment and
can see the outcome, it is possible to say which direction you saw a difference, if there was a
difference. However, you should refrain from mentioning the directionality in your experimental
hypotheses (e.g., there is a difference, not that one group is higher or lower than the other).
Figure A.5f.
Detailed Data Histogram
The web-interface will present the independent variable data in a histogram for each group depicted
in different colors (red for group A and green for group B). Their respective medians are indicated as
a red and green line respectively. Each bar in the histogram represents the number of students (as
indicated by the y-axis) which had an average reaction time indicated by its position on the x-axis.
Retrieving Filtered Data Based on Questionnaire's Independent Variables
If you prefer, instead of comparing all data between two test types, you can instead focus on one test
type and filter by demographic variables.
To access specific data filtered by independent variables derived from the questionnaire, follow these
steps:
1. Selecting the Questionnaire List:
There are eight distinct questionnaire lists, numbered 0-7, each with its unique set of options.
The blue window labeled "Questionnaire Lists" (depicted in Figure A.6a
) governs your choices.
Figure A.6a.
Upon selecting one of the eight options, several sub-windows will appear below the current window
(see Figure A.6b
).
Figure A.6b.
2. Choosing Independent Variables:
Read through the header questions in each panel to pinpoint your independent variable of
interest (e.g. “What time of day the MIT was completed [start]”).
Selecting Two Groups:
Proceed to select your two groups for comparison (eg. ‘morning’ & ‘afternoon’).
You are only able to compare groups listed under each independent variable.
The order in which you choose "Group A" and "Group B" has no bearing on your results. (as
illustrated in Figure A.6c
).
Figure A.6c.
Variable Selection Criteria:
Note that due to the ongoing nature of this project, some independent variables may not
display all possible options.
Your preview ends here
Eager to read complete document? Join bartleby learn and gain access to the full version
- Access to all documents
- Unlimited textbook solutions
- 24/7 expert homework help
For privacy reasons independent variables are shown only if there are at least 50 subjects in
the group.
3. Displaying the Data:
Once you have selected the two groups you want to compare, proceed by clicking the labeled
variable button (labeled as '
start
' in Figure A.6c
).
This action will prompt the display of the filtered data, as depicted in Figure A.6d
.
Figure A.6d.
How to Retrieve the p-value
In this example, we're comparing the performance of subjects who took the MIT picture test in the
morning (n = 3556) versus those who took it in the afternoon (n = 2770). Below the results, you'll
notice the "start" parameter window highlighted in dark yellow, indicating our current selection. Here
"start" signifies the time of day the test was administered.
For the morning test subjects, the median number of correct responses is 139, compared to 138 for
the afternoon test subjects. Additionally, the average response time for the morning test subjects is
817 milliseconds [msec], whereas it is 804 msec for the afternoon test subjects (see Figure A.6c
).
This suggests a slightly lower accuracy (one less correct) and a faster speed (13 milliseconds) in the
afternoon. (Remember that for your paper you will focus on EITHER accuracy or speed, but not
both.)
However, just observing the differences is not enough. For a scientific study it’s important to generate
a p-value to better assess the significance of the difference. Again, to calculate the p-value, we need
to resample the data through a simulation, as explained previously (refer to Figure A.3
). To initiate
the simulation, click on the prominent gray button labeled in red as 'Calculate p-value' (depicted in
Figure A.7a
).
Figure A.7a.
Following the simulation, we obtain a p-value of 0.4672 for Experiment 1 and a p-value of 0.0001 for
Experiment 2.
Figure A.7b.
Additional Information on effect size and p-value
Effect size measures the extent of difference between sample groups. For researchers evaluating
treatment options for patients, effect size holds great practical significance, not just statistical
significance. But, even an effect size and a p-value alone does not give you any sense of the
magnitude of the estimated effect size. In our example (Experiment 2) there is a difference of about
2% (13/803) . We need to know how precise that 2% estimate was. Could it have been 5%? We
need what is called a confidence interval
for that estimate of 2%, to quantify that uncertainty. We
are not going into details about that in LS23L. LS40 will discuss this in detail, if you are interested.
In Figure A.7b
, the effect size for the '
# Correct Responses
' between these two groups is small,
reflecting a difference of 1 correct response. Conversely, the effect size for '
Average Response
Time
' between these two groups is around 2% at a 13-millisecond difference.
As demonstrated in the effect size histogram graph
for Experiment 1, approximately 50% of the
10,000 simulated calculations, highlighted in red, exhibited a larger effect size than the two groups
being compared, whereas in Experiment 2 not one out of 10,000 simulations showed larger or equal
effect size (>=13ms), which is p <0.01%. This indicates that results as extreme as 13ms happen
under the Null Hypothesis less than 0.01% of the time.
The Aggregated Database
In Table 1
, you'll find a list with the questions for various sub-windows as they appear on the web
interface. It's important to note that if the sample size (n) falls below 50, the data may not be
accessible for testing. It's worth keeping in mind that this database is dynamic, and the available data
will undergo changes throughout the quarter as data gets added.
Table 1: Questions of Demographic Sub-Windows 0-7
Category
0
1
2
3
4
5
Theme
Situational Demographic Language Background History
Substanc
Use
Questions
Day of Week
the MIT was
completed.
Age
Fluent in
how many
languages
Country of
Birth
Have you ever
received
special
education
services?
Coffee
Frequency/
How long a
How fast the
MIT was
completed.
Gender
Primary
Language
Use
(Spoken)
City of Birth
Ever had loss
of
consciousness?
Alcohol
Frequency/
How long a
What time of
day the MIT
was started.
Orientation
Primary
Language
Use
(Reading)
If you live in
the US which
part
if yes duration
(indicate worst)
Tea
Frequency/
How long a
Is this your
first time
performing
the URI-
UCLA
Memory
Interference
Test?
Race/Ethnicity
Primary
Language
Use
(Writing)
How many
years have you
lived here
Loss of
consciousness
incident
Caffeinated
Frequency/
How long a
Your preview ends here
Eager to read complete document? Join bartleby learn and gain access to the full version
- Access to all documents
- Unlimited textbook solutions
- 24/7 expert homework help
Last time
performed
this task:
Education
COMPLETED
Primary
Language
Spoken by
Father
What best
describes your
area
Do you have
family history of
left
handedness?
Tobacco
Frequency/
How long a
Please
indicate what
trial this is:
Ethnic Group
Primary
Language
Spoken by
Mother
Handedness
Fluent in
how many
languages?
8. Appendix: An Example of Comparing Two Groups Using
Resampling
(Used in Scientific Methodology and the MIT)
Based on Section 6.3 of “Understanding Data: an Experimental Approach to Statistics” by Alan
Garfinkel and Yina Guo
In a hypothetical case study, we are evaluating a potential treatment for an immune disease by
testing its effect on the number of T-cells in culture (more T-cells means the treatment is better). This
test is unpaired (also called independent because the data come from two different groups of
subjects. We could have made this a paired test by applying both treatments to pairs of genetically
identical T-cells.
We have 49 measurements in the Control group and 45 measurements in the Treatment group,
depicted in bee swarm and box plots in Figure AA.1
below. Since the data is not “bell-shaped” with
unimodal, symmetric, and thin-tailed distribution, we should use the descriptive statistic of the
median. Our effect size is the difference in medians, which is 69.5 - 59.8 = 9.7 T-cells per unit area
(calculated as Treatment - Control since Treatment is larger).
Return to top
Your preview ends here
Eager to read complete document? Join bartleby learn and gain access to the full version
- Access to all documents
- Unlimited textbook solutions
- 24/7 expert homework help
Figure AA.1.
Experimental hypothesis:
Treatment plates have a different median number of T-cells per unit area
than Control plates.
Null hypothesis:
There is no difference in the number of T-cells between the Control and Treatment
plates (and any observed difference is due to random sampling).
When we combine the two groups of collected measurements (in a “Big Box”) and resample new
datasets 10,000 times, we found results equally or more extreme than our difference of medians
never occurred. Thus, the p-value is less than 1 in 10,000 (0.0001). Therefore, we can conclude that
the drug treatment produced an absolute increase in T-cell numbers that was statistically significant
and reject our null hypothesis.
Your preview ends here
Eager to read complete document? Join bartleby learn and gain access to the full version
- Access to all documents
- Unlimited textbook solutions
- 24/7 expert homework help
Figure AA.2.
To learn more about statistical analysis, we recommend taking LS 40: Statistics of Biological Systems
and reading its textbook from which this example was taken.
Your preview ends here
Eager to read complete document? Join bartleby learn and gain access to the full version
- Access to all documents
- Unlimited textbook solutions
- 24/7 expert homework help
Related Documents
Recommended textbooks for you
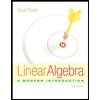
Linear Algebra: A Modern Introduction
Algebra
ISBN:9781285463247
Author:David Poole
Publisher:Cengage Learning
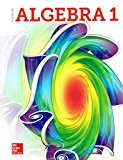
Glencoe Algebra 1, Student Edition, 9780079039897...
Algebra
ISBN:9780079039897
Author:Carter
Publisher:McGraw Hill
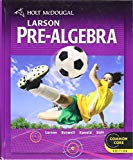
Holt Mcdougal Larson Pre-algebra: Student Edition...
Algebra
ISBN:9780547587776
Author:HOLT MCDOUGAL
Publisher:HOLT MCDOUGAL
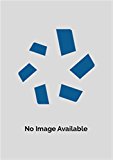
Big Ideas Math A Bridge To Success Algebra 1: Stu...
Algebra
ISBN:9781680331141
Author:HOUGHTON MIFFLIN HARCOURT
Publisher:Houghton Mifflin Harcourt
Recommended textbooks for you
- Linear Algebra: A Modern IntroductionAlgebraISBN:9781285463247Author:David PoolePublisher:Cengage LearningGlencoe Algebra 1, Student Edition, 9780079039897...AlgebraISBN:9780079039897Author:CarterPublisher:McGraw HillHolt Mcdougal Larson Pre-algebra: Student Edition...AlgebraISBN:9780547587776Author:HOLT MCDOUGALPublisher:HOLT MCDOUGAL
- Big Ideas Math A Bridge To Success Algebra 1: Stu...AlgebraISBN:9781680331141Author:HOUGHTON MIFFLIN HARCOURTPublisher:Houghton Mifflin Harcourt
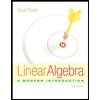
Linear Algebra: A Modern Introduction
Algebra
ISBN:9781285463247
Author:David Poole
Publisher:Cengage Learning
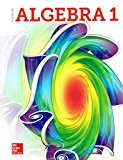
Glencoe Algebra 1, Student Edition, 9780079039897...
Algebra
ISBN:9780079039897
Author:Carter
Publisher:McGraw Hill
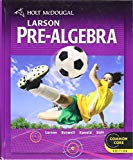
Holt Mcdougal Larson Pre-algebra: Student Edition...
Algebra
ISBN:9780547587776
Author:HOLT MCDOUGAL
Publisher:HOLT MCDOUGAL
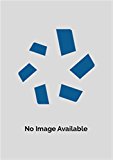
Big Ideas Math A Bridge To Success Algebra 1: Stu...
Algebra
ISBN:9781680331141
Author:HOUGHTON MIFFLIN HARCOURT
Publisher:Houghton Mifflin Harcourt