10.2, 10.3_
pdf
keyboard_arrow_up
School
University of Illinois, Chicago *
*We aren’t endorsed by this school
Course
361
Subject
Statistics
Date
Feb 20, 2024
Type
Pages
21
Uploaded by PresidentTurtlePerson1585
Stat 361: Section 10.2; Section 10.3
Page 1
Section 10.2- Testing a Statistical Hypothesis
Section 10.3 - The Use of
p
-value for Decision Making in Testing Hypotheses
INTRODUCTION
Q 1.
What will we be doing in these sections?
Goals:
•
Part of Step 3 of Hypothesis Testing.
•
Learn how to make decisions and draw conclusions as a result of the hypot-
hesis test.
•
Investigate potential problems with performing a hypothesis test.
Definition 1
(Test Statistic)
.
blank
In Step 2 of Hypothesis Testing, we calculate something called a
test statistic
. A test statistic
is a value calculated from data. We can either work with a test statistic directly from the
data, or centered and re-scaled to enable comparisons.
These test statistics enable us to
determine whether to Reject
H
0
or not.
Q 2.
How can we make decisions?
CRITICAL REGION APPROACH
Definition 2
(Critical Region (CR))
.
blank
A region where we would reject the null hypothesis. We have either 1 region or 2 regions
(depending on our alternative hypothesis), where we would Reject
H
0
. If we see a test sta-
tistic value within this “rejection” region, we would make the decision to Reject
H
0
.
Indicated by: “Reject
H
0
if
”.
Definition 3
(Critical Value)
.
blank
The value(s) where the “rejection” region(s) starts.
Stat 361: Section 10.2; Section 10.3
Page 2
Example
1
.
A cold vaccine is known to be only 25% e
↵
ective after 2 years. To determine if
a new vaccine is superior in providing protection against the same virus for a longer period
of time, suppose 20 people are chosen at random and inoculated.
Doctors believe that if more than 8 of those with the new vaccine surpass the 2-year period
without contracting the virus, the new vaccine will be considered superior.
(a) What are the hypotheses for this scenario?
(b) Where does the 8 come from in the statement “Doctors believe...”?
Solution:
•
It is somewhat arbitrary.
•
It appears reasonable as a modest gain in the number of people who have not
gotten sick.
•
How many people would we expect to be protected?
•
6 people and 7 people are still “reasonably” close to 5, so we choose a number of
people slightly larger than this.
•
If you still felt that 8 were too close to 5, you could choose 9 instead.
(c) When would we make the decision to Reject
H
0
or Do Not Reject
H
0
?
Stat 361: Section 10.2; Section 10.3
Page 3
(d) How can we represent our critical region as a picture? How can we formally write down
the critical region?
Remark
1
.
blank
1) For a 1-sided test, the sign on the critical region is the
same
as the sign on the
alternative hypothesis.
2) For a 2-sided test, there are two critical regions.
Your preview ends here
Eager to read complete document? Join bartleby learn and gain access to the full version
- Access to all documents
- Unlimited textbook solutions
- 24/7 expert homework help
Stat 361: Section 10.2; Section 10.3
Page 4
Example
2
.
We are interested in the average weight of male college students at a certain
college. We believe that they weight 68 kg (
⇡
150 pounds). We want to see if the average
weight is di
↵
erent from this.
(a) What are the hypotheses for this scenario?
(b) What are some potential critical values?
•
If we do not want to Reject
H
0
, then the sample mean should be “close” to our
hypothesized value of 68.
•
If we Reject
H
0
, then the sample mean should be “far” from our hypothesized
value of 68.
•
Our critical values could potentially be 67 and 69.
(c) How can we represent the critical region with a picture? When would we make the
decision to Reject
H
0
or Do Not Reject
H
0
?
Stat 361: Section 10.2; Section 10.3
Page 5
HOW TO FIND CRITICAL VALUES
Q 3.
How can we find critical values?
•
We have seen that critical values can be somewhat arbitrary.
•
Are there any guidelines as to how to determine which ones we should use?
Q 4.
What are the critical values and critical region for a 2-sided (tailed) test?
Hypothesis Test Setup:
H
0
:
p
=
p
0
versus
H
1
:
p
6
=
p
0
Preset
↵
:
Suppose
↵
= 0
.
10.
Test Statistic Values (establish a critical region based on
↵
):
Stat 361: Section 10.2; Section 10.3
Page 6
Q 5.
What are some other potential critical values for a 2-sided test involving
Z
as a test
statistic?
↵
↵
/
2
Critical Values
0.09
0.045
±
z
0
.
045
=
±
invnorm
(1
-
0
.
045) =
±
1
.
70
0.08
0.04
±
z
0
.
040
=
±
invnorm
(1
-
0
.
040) =
±
1
.
75
0.07
0.035
±
z
0
.
035
=
±
invnorm
(1
-
0
.
035) =
±
1
.
81
0.06
0.03
±
z
0
.
030
=
±
invnorm
(1
-
0
.
030) =
±
1
.
88
0.05
0.025
±
z
0
.
025
=
±
invnorm
(1
-
0
.
025) =
±
1
.
96
Example
3
.
Suppose we are testing
H
0
:
p
= 0
.
4
versus H
1
:
p
6
= 0
.
4
.
The success / failure
condition is met. If
↵
= 0
.
07, then the critical values are
±
1
.
81. The critical region is
Reject
H
0
if
z <
-
1
.
81 OR if
z >
1
.
81
.
(a) If we find, from our data, that
z
= 1
.
85, what decision should we make?
(b) If we find, from our data, that
z
=
-
1
.
75, what decision should we make?
Your preview ends here
Eager to read complete document? Join bartleby learn and gain access to the full version
- Access to all documents
- Unlimited textbook solutions
- 24/7 expert homework help
Stat 361: Section 10.2; Section 10.3
Page 7
Q 6.
What are the critical values and critical region for a 1-sided, lower tailed test?
Hypothesis Test Setup:
H
0
:
p
=
p
0
versus
H
1
:
p < p
0
Preset
↵
:
Suppose
↵
= 0
.
05.
Test Statistic Value (establish a critical region based on
↵
):
Q 7.
What are some other potential critical values for a 1-sided, lower tailed test involving
Z
as a test statistic?
↵
Critical Value
0.10
-
z
0
.
10
=
-
1
.
28
0.09
-
z
0
.
09
=
-
1
.
34
0.08
-
z
0
.
08
=
-
1
.
41
↵
Critical Value
0.07
-
z
0
.
07
=
-
1
.
48
0.06
-
z
0
.
06
=
-
1
.
55
0.05
-
z
0
.
05
=
-
1
.
645
Stat 361: Section 10.2; Section 10.3
Page 8
Example
4
.
Suppose we are testing
H
0
:
p
= 0
.
4
versus H
1
:
p <
0
.
4. The success / failure
condition is met. If
↵
= 0
.
07, then the critical value is
-
1
.
48. The critical region is
Reject
H
0
if
z <
-
1
.
48
.
(a) If we find, from our data, that
z
= 1
.
2, what decision should we make?
(b) If we find, from our data, that
z
=
-
1
.
5, what decision should we make?
Q 8.
What are the critical values and critical region for a 1-sided, upper tailed test?
Hypothesis Test Setup:
H
0
:
p
=
p
0
versus
H
1
:
p > p
0
Preset
↵
: Suppose
↵
= 0
.
10.
Test Statistic Value (establish a critical region based on
↵
):
Stat 361: Section 10.2; Section 10.3
Page 9
Q 9.
What are some other potential critical values for a 1-sided, upper tailed test involving
Z
as a test statistic?
↵
Critical Value
0.10
z
0
.
10
= 1
.
28
0.09
z
0
.
09
= 1
.
34
0.08
z
0
.
08
= 1
.
41
↵
Critical Value
0.07
z
0
.
07
= 1
.
48
0.06
z
0
.
06
= 1
.
55
0.05
z
0
.
05
= 1
.
645
Example
5
.
Suppose we are testing
H
0
:
p
= 0
.
4
versus H
1
:
p >
0
.
4. The success / failure
condition is met. If
↵
= 0
.
07, then the critical value is 1
.
48. The critical region is
Reject
H
0
if
z >
1
.
48
.
(a) If we find, from our data, that
z
= 1
.
7, what decision should we make?
(b) If we find, from our data, that
z
= 0
.
1, what decision should we make?
Q 10.
What are the critical values for di
↵
erent scenarios?
Solution:
The distribution used to find critical values depends on the hypothesis test performed and
the information we know about the problem.
•
1 Proportion: Use
z
.
•
Di
↵
erence of Proportions: Use
z
.
•
1 Mean
–
If
σ
is known, use
z
.
–
If
σ
is unknown, use
t
.
•
Paired Data: Use
t
.
•
Di
↵
erence of Means:
–
If
σ
2
1
and
σ
2
2
are both known, use
z
.
–
If
σ
2
1
and
σ
2
2
are both unknown,
use
t
.
•
Variance: Use
χ
2
.
•
Ratio of Variances: Use
F
.
Your preview ends here
Eager to read complete document? Join bartleby learn and gain access to the full version
- Access to all documents
- Unlimited textbook solutions
- 24/7 expert homework help
Stat 361: Section 10.2; Section 10.3
Page 10
POTENTIAL ERRORS
Q 11.
What are some of the possible errors that could arise as a result of a hypothesis test?
1) Reject
H
0
, given
H
0
is true (Type I Error).
2) Do not reject
H
0
, given
H
0
is false (Type II Error).
Example
6
.
Suppose we have a trial. How can we relate this to the errors of a hypothesis
test?
Decision:
Jail
Set Free
Truth:
Innocent
blankblankblank
blankblankblank
Guilty
Decision:
Reject
H
0
Do Not Reject
H
0
Truth:
H
0
True
blankblankblank
blankblankblank
H
0
False
blankblank
Definition 4
(Probability of a Type I Error)
.
P
(Type I Error) =
P
(Reject
H
0
|
H
0
True)
=
↵
= Level of Significance
= Size of the test
.
•
This can be calculated directly from your null hypothesis and your critical region.
•
Usually we preset this value.
•
This
↵
, the
↵
-level for the critical value, and the
↵
for a confidence interval are all the
same.
Stat 361: Section 10.2; Section 10.3
Page 11
Definition 5
(Probability of a Type II Error)
.
P
(Type II Error) =
P
(Do Not Reject
H
0
|
H
0
False)
=
β
.
This can be calculated only if you have a specific alternative hypothesis.
Has an inverse
relationship with
↵
.
Definition 6
(Power of the Test)
.
blank
The probability that we correctly reject the null hypothesis when it is false.
power
= 1
-
β
= 1
-
P
(Do Not Reject
H
0
|
H
0
False)
=
P
(Reject
H
0
|
H
0
False)
Ideally, we want the power as high as possible, which means that
β
should be as low as
possible.
Example
7
.
We are interested in the average weight of male college students at a certain
college. We believe that they weigh 68 kg (
⇡
150 pounds). We want to see if the average
weight is di
↵
erent from this.
Hypotheses:
H
0
:
μ
= 68
versus
H
1
:
μ
6
= 68
Critical Region:
Reject
H
0
if ¯
x <
67 or if ¯
x >
69
Additional Assumptions:
•
Take a random sample of size 36.
•
Assume the population standard deviation is
σ
= 3
.
6.
Questions:
(a) Find the probability of a Type I Error. From the CLT, we remember that
E
(
¯
X
) =
μ
and
SD
(
¯
X
)
=
σ
/
p
n
= 3
.
6
/
p
36 = 0
.
6.
Stat 361: Section 10.2; Section 10.3
Page 12
(b) Find the probability of a Type II Error if we consider the specific alternative
hypothesis
H
1
:
μ
= 68
.
5.
β
=
P
(Do Not Reject
H
0
|
H
0
False)
=
P
(
67
¯
X
69
|
μ
= 68
.
5
)
=
P
(
-
2
.
5
< Z <
0
.
83)
=
normalcdf
(
-
2
.
5
,
0
.
83) = 0
.
7905
.
If
μ
is actually 68.5, then this test will not reject the null hypothesis 79.05%
of the time.
Note: We used the fact that
μ
= 68
.
5 in our calculation of the
z
-scores:
z
1
=
67
-
68
.
5
0
.
6
=
67
-
68
.
5
3
.
6
/
p
36
=
-
2
.
5
z
2
=
69
-
68
.
5
0
.
6
=
69
-
68
.
5
3
.
6
/
p
36
= 0
.
83
(c) Find the power of the test for the alternative described above.
Describe
what this means.
•
This means that if
μ
is truly 68.5, the test as described will properly
reject the null hypothesis only 20.95% of the time.
•
This is not a good test if it is important that the analyst has a reasonable
chance of being able to distinguish between a mean of 68 and 68.5.
Your preview ends here
Eager to read complete document? Join bartleby learn and gain access to the full version
- Access to all documents
- Unlimited textbook solutions
- 24/7 expert homework help
Stat 361: Section 10.2; Section 10.3
Page 13
Example
8
.
A cold vaccine is known to be only 25% e
↵
ective after 2 years. Suppose 20 people
are chosen at random and inoculated. Doctors believe that if more than 8 of those with the
new vaccine surpass the 2-year period without contracting the virus, the new vaccine will be
considered superior.
Hypotheses:
H
0
:
p
= 0
.
25
versus
H
1
:
p >
0
.
25
Critical Region:
Reject
H
0
if
X >
8
Questions:
(a) Find the probability of a Type I Error.
A Type I Error will occur with a 4.09% chance. 4.09% of all samples of size
20 will reject
p
= 0
.
25 when
p
= 0
.
25 is actually true.
(b) Find the probability of a Type II Error if we consider the specific alternative
hypothesis
H
1
:
p
= 0
.
5.
β
p
=0
.
5
=
P
(Do Not Reject
H
0
|
H
0
False)
=
P
(
X
8
|
p
= 0
.
5) =
8
X
x
=0
✓
20
x
◆
(0
.
5)
x
(0
.
5)
20
-
x
=
binomialcdf
(20
,
0
.
5
,
8)
= 0
.
2517
.
This value is a bit high. It means that it is likely that we will reject the
new vaccine when it is actually better than the current one (50% e
↵
ective
versus 25% e
↵
ective).
Stat 361: Section 10.2; Section 10.3
Page 14
(c) Find the probability of a Type II Error if we consider the specific alternative
hypothesis
H
1
:
p
= 0
.
7.
β
p
=0
.
7
=
P
(Do Not Reject
H
0
|
H
0
False)
=
P
(
X
8
|
p
= 0
.
7)
=
binomialcdf
(20
,
0
.
7
,
8)
= 0
.
0051
.
This value has dropped and is now very small. It is unlikely that the new
vaccine would be rejected when it was 70% e
↵
ective.
(d) Find the power of the test for each of the alternatives described above.
VISUALIZATION OF ERRORS VIA PROPORTIONS
Recall 1.
blank
Note: Sometimes we calculate
↵
and
β
for proportions using the Binomial Distribution, and
sometimes we calculate it using the Normal Approximation to the Binomial Distribution.
Recall how we can do this approximation:
•
We can do this when
n
is large and
p
is not close to 0 or 1. We require that
np
≥
5 and
n
(1
-
p
)
≥
5
.
•
For the Binomial Distribution,
E
(
X
) =
np
,
V
(
X
) =
np
(1
-
p
) and
SD
(
X
) =
p
np
(1
-
p
).
•
Suppose
X
⇠
Bin
(
n, p
). Then (ignoring any continuity corrections)
P
(
X <
5)
⇡
P
Z
5
-
np
p
np
(1
-
p
)
!
.
Stat 361: Section 10.2; Section 10.3
Page 15
Q 12.
How can we visualize our errors for our proportion example?
Q 13.
How can we summarize the relationships between
↵
,
β
, 1
-
β
in the graph above?
•
If we shift
p
1
to the left, then
↵
stays the same,
β
increases, and power decreases.
•
If we decrease
↵
(moving
p
⇤
to the right), then
β
increases so power decreases.
•
If
p
1
shifts right, then
↵
stays the same,
β
decreases, and power increases.
•
The only way to decrease both
↵
and
β
at the same time is to increase sample size.
•
In practice, you usually choose
↵
first, and then choose the test that has the highest
power.
Your preview ends here
Eager to read complete document? Join bartleby learn and gain access to the full version
- Access to all documents
- Unlimited textbook solutions
- 24/7 expert homework help
Stat 361: Section 10.2; Section 10.3
Page 16
THE P-VALUE APPROACH
Definition 7
(P-Value)
.
blank
A
p
-value is the probability that you observe your test statistic value or something even more
extreme, given that the null hypothesis is true.
p
-value =
P
(observed value or more extreme
|
H
0
true)
Interpretation Example:
If
p
-value = 0
.
03, then there is a 3% chance of observing the test statistic value
from our data,
given that the null hypothesis is true
.
Definition 8
(Alternative Definitions of P-Values)
.
blank
•
The lowest level of significance at which the observed value of the test statistic is
significant.
•
A measure of the evidence in the data supporting
H
0
.
–
A “large”
p
-value indicates there is more evidence in favor of
H
0
.
–
A “small”
p
-value indicates there is more evidence in favor of
H
1
.
Q 14.
How can we calculate a
p
-value during a hypothesis test?
•
The calculation depends on the alternative chosen.
•
The calculation depends on the test statistic value.
Stat 361: Section 10.2; Section 10.3
Page 17
Examples:
Test
Null Hypothesis
Alternative Hypothesis
p
-value Calculation
1 Mean
μ
=
μ
0
μ < μ
0
P
(
Z < z
)
σ
known
μ > μ
0
P
(
Z > z
)
μ
6
=
μ
0
2
·
P
(
Z >
|
z
|
)
1 Mean
μ
=
μ
0
μ < μ
0
P
(
T < t
)
σ
unknown
μ > μ
0
P
(
T > t
)
μ
6
=
μ
0
2
·
P
(
T >
|
t
|
)
Paired
μ
=
μ
0
μ
D
< d
0
P
(
T < t
)
Observations
μ
D
> d
0
P
(
T > t
)
μ
D
6
=
d
0
2
·
P
(
T >
|
t
|
)
Di
↵
erence of Means
μ
1
-
μ
2
=
d
0
μ
1
-
μ
2
< d
0
P
(
Z < z
)
σ
2
1
,
σ
2
2
μ
1
-
μ
2
> d
0
P
(
Z > z
)
known
μ
1
-
μ
2
6
=
d
0
2
·
P
(
Z >
|
z
|
)
Di
↵
erence of Means
μ
1
-
μ
2
=
d
0
μ
1
-
μ
2
< d
0
P
(
T < t
)
σ
2
1
,
σ
2
2
unknown
μ
1
-
μ
2
> d
0
P
(
T > t
)
σ
2
1
=
σ
2
2
μ
1
-
μ
2
6
=
d
0
2
·
P
(
T >
|
t
|
)
Di
↵
erence of Means
μ
1
-
μ
2
=
d
0
μ
1
-
μ
2
< d
0
P
(
T < t
)
σ
2
1
,
σ
2
2
unknown
μ
1
-
μ
2
> d
0
P
(
T > t
)
σ
2
1
6
=
σ
2
2
μ
1
-
μ
2
6
=
d
0
2
·
P
(
T >
|
t
|
)
1 Proportion
p
=
p
0
p < p
0
P
(
X
x
|
p
=
p
0
)
Binomial RV
p > p
0
P
(
X
≥
x
|
p
=
p
0
)
p
6
=
p
0
2
·
P
(
X
x
|
p
=
p
0
) if
x < np
0
2
·
P
(
X
≥
x
|
p
=
p
0
) if
x > np
0
1 Proportion
p
=
p
0
p < p
0
P
(
Z < z
)
Normal Approximation
p > p
0
P
(
Z > z
)
p
6
=
p
0
2
·
P
(
Z >
|
z
|
)
Di
↵
erence of
p
1
=
p
2
p
1
< p
2
P
(
Z < z
)
Proportions
p
1
> p
2
P
(
Z > z
)
p
1
6
=
p
2
2
·
P
(
Z >
|
z
|
)
Variance
σ
2
=
σ
2
0
σ
2
<
σ
2
0
P
(
χ
2
<
test stat)
1 Population
σ
2
>
σ
2
0
P
(
χ
2
>
test stat)
σ
2
6
=
σ
2
0
Critical Region is Better
Ratio of Variances
σ
2
1
=
σ
2
2
σ
2
1
<
σ
2
2
P
(
F <
test stat)
2 Populations
σ
2
1
>
σ
2
2
P
(
F >
test stat)
σ
2
1
6
=
σ
2
2
2
·
P
(
F <
test stat) if
F <
1
2
·
P
(
F >
test stat) if
F >
1
Stat 361: Section 10.2; Section 10.3
Page 18
Q 15.
What happens when I obtain a small
p
-value?
Example
9
.
We want to know if motorcycle helmet use in Florida has declined among riders
under the age of 21 after helmet laws changed to be less strict? Before the law changed, we
know that only 60% of those under 21 wore helmets.
To answer this question, a random, representative sample was taken of 781 riders under the
age of 21. Of these, 396 were wearing helmets.
Hypotheses:
P-Value Calculation:
p
-value =
P
✓
ˆ
p <
396
781
p
= 0
.
6
◆
=
P
(ˆ
p <
0
.
507
|
p
= 0
.
6)
⇡
P
0
@
Z <
0
.
507
-
0
.
6
q
0
.
6(1
-
0
.
6)
781
1
A
⇡
P
(
Z <
-
5
.
31)
=
normalcdf
(
-
999
,
-
5
.
31)
= 5
.
49
⇥
10
-
8
⇡
0
The closer you are to the null hypothesis value, the larger the
p
-value will be.
The farther away you are from the null hypothesis value, the smaller the
p
-value
(provided the alternative hypothesis is the correct direction).
P-Value Meaning:
Our
p
-value means that if the true rate of helmet wearing
among riders less than 21 years old were still 60%, we would expect to see a rate
no higher than 50.7% in a sample of 781 riders less than 21 years old less than 1
chance in 1000.
Decision:
Conclusion:
•
There is strong evidence that there is a decline in helmet use among riders
less than 21 years old.
•
The closer the
p
-value is to 0, the stronger the evidence provided.
•
If you Reject
H
0
, then we do not know any information on the population
parameter. To see where it could be, create a confidence interval.
Your preview ends here
Eager to read complete document? Join bartleby learn and gain access to the full version
- Access to all documents
- Unlimited textbook solutions
- 24/7 expert homework help
Stat 361: Section 10.2; Section 10.3
Page 19
Q 16.
What happens when I obtain a large
p
-value?
Example
10
.
blank
•
Therapeutic Touch (TT) is a therapy method where the practitioner moves their hands
near a patient to manipulate a “human energy field”.
•
15 TT practitioners were randomly chosen.
•
An experiment was performed to determine if the practitioners could determine whet-
her an unseen hand was hovering over their left or right hand (determined by a coin
flip).
•
Each practitioner attempted 10 trials.
•
In total, there were 150 trials.
The TT practitioners were successful 70 times.
We
want to determine if TT can detect where the “human energy field” is.
Hypotheses:
P-Value Calculation:
p
-value =
P
✓
ˆ
p >
70
150
p
= 0
.
5
◆
=
P
(ˆ
p >
0
.
467
|
p
= 0
.
5)
⇡
P
0
@
Z >
0
.
467
-
0
.
5
q
0
.
5(1
-
0
.
5)
150
1
A
⇡
P
(
Z >
-
0
.
81)
= 0
.
7910
Decision:
Conclusion:
In order to Reject
H
0
, the practitioners should be doing better than
guessing, not worse.
Stat 361: Section 10.2; Section 10.3
Page 20
Example
11
.
Which of the following statements are true?
(a) A very high
p
-value is strong evidence that the null hypothesis is false.
(b) A very low
p
-value proves that the null hypothesis is false.
(c) If the null hypothesis is true, you can’t get a
p
-value below 0
.
01.
HYPOTHESIS TESTING USING CRITICAL REGIONS: SUMMARY
Report the following information:
•
Null and Alternative Hypotheses using symbols
•
Test Statistic Value
•
↵
value
•
Critical Region:
Reject
H
0
if [insert criteria to be met for rejection]
•
Decision: Reject
H
0
or Do Not Reject
H
0
•
Conclusion: Explain, in words, what your decision means in the context of the problem.
Stat 361: Section 10.2; Section 10.3
Page 21
HYPOTHESIS TESTING USING P-VALUES: SUMMARY
Report the following information:
•
Null and Alternative Hypotheses using symbols
•
Test Statistic Value
•
P
-Value Calculation
•
Decision: Reject
H
0
or Do Not Reject
H
0
•
Conclusion: Explain, in words, what your decision means in the context of the problem.
General Guideline for Decision:
•
If
p
-value
<
↵
, Reject
H
0
.
•
If
p
-value
6
<
↵
, Do Not Reject
H
0
.
•
↵
depends on how much Type I Error you are willing to have.
•
↵
does
NOT
always equal 0
.
05.
ALPHA DOES NOT ALWAYS EQUAL 0.05
What we SHOULD NOT do regarding
p
-value’s (taken directly from below reference):
•
Don’t base your conclusions solely on whether an association or e
↵
ect was found to
be “statistically significant” (i.e. the
p
-value passed some arbitrary threshold such as
p <
0
.
05).
•
Don’t believe that an association or e
↵
ect exists just because it was statistically signi-
ficant.
•
Don’t believe that an association or e
↵
ect is absent just because it was not statistically
significant.
•
Don’t believe that your
p
-value gives the probability that chance alone produced the
observed association or e
↵
ect or the probability that your test hypothesis is true.
•
Don’t conclude anything about scientific or practical importance based on statistical
significance (or lack thereof).
Reference: Ronald L. Wasserstein, Allen L. Schirm & Nicole A. Lazar (2019) Moving to a
World Beyond “
p <
0
.
05”, The American Statistician, 73:S1, 1-19,
DOI:10.1080/00031305.2019.1583913
Your preview ends here
Eager to read complete document? Join bartleby learn and gain access to the full version
- Access to all documents
- Unlimited textbook solutions
- 24/7 expert homework help
Related Documents
Recommended textbooks for you
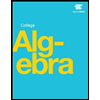
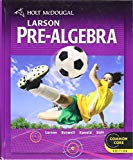
Holt Mcdougal Larson Pre-algebra: Student Edition...
Algebra
ISBN:9780547587776
Author:HOLT MCDOUGAL
Publisher:HOLT MCDOUGAL
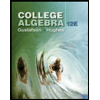
College Algebra (MindTap Course List)
Algebra
ISBN:9781305652231
Author:R. David Gustafson, Jeff Hughes
Publisher:Cengage Learning
Recommended textbooks for you
- Holt Mcdougal Larson Pre-algebra: Student Edition...AlgebraISBN:9780547587776Author:HOLT MCDOUGALPublisher:HOLT MCDOUGALCollege Algebra (MindTap Course List)AlgebraISBN:9781305652231Author:R. David Gustafson, Jeff HughesPublisher:Cengage Learning
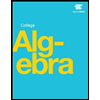
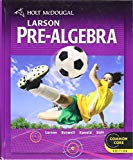
Holt Mcdougal Larson Pre-algebra: Student Edition...
Algebra
ISBN:9780547587776
Author:HOLT MCDOUGAL
Publisher:HOLT MCDOUGAL
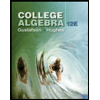
College Algebra (MindTap Course List)
Algebra
ISBN:9781305652231
Author:R. David Gustafson, Jeff Hughes
Publisher:Cengage Learning