STA4164 Assignment 1 S24 (1)
docx
keyboard_arrow_up
School
University of West Florida *
*We aren’t endorsed by this school
Course
4164
Subject
Statistics
Date
Feb 20, 2024
Type
docx
Pages
15
Uploaded by SargentResolve4477
STA4164 – Assignment 1 (100 pts)
(Due Feb 12 for section 0001 and Feb 13 for section 0002)
[10 pts] Question 1 (Chapter 5):
In an analysis of daily soil evaporation (EVAP), Freund (1979) identified the following 10 predictor variables to predict daily soil evaporation:
MAXAT: Maximum daily air temperature
MINAT: Minimum daily air temperature
AVAT: Integrated area under the daily air temperature curve (i.e., a measure of average air temperature)
MAXST: Maximum daily soil temperature
MINST: Minimum daily soil temperature
AVST: Integrated area under the daily soil temperature curve
MAXH: Maximum daily relative humidity
MINH: Minimum daily relative humidity
AVH: Integrated area under the daily relative humidity curve
WIND: Total wind, measured in miles per day
In this analysis, maximum daily air temperature was used as the sole predictor of daily soil evaporation. Measurements were recorded across 46 days in June and July. Use the R output below to answer the following questions.
Scatterplot:
Time vs. Residual Plot:
Residual Plot:
Normal Probability Plot:
Call:
lm(formula = EVAP ~ MAXAT, data = evap_data)
Residuals:
Min 1Q Median 3Q Max -32.130 -2.369 1.781 6.195 16.692 Coefficients:
Estimate Std. Error t value Pr(>|t|) (Intercept) -154.9245 27.3427 -5.666 1.04e-06 ***
MAXAT 2.0895 0.3009 6.945 1.38e-08 ***
---
Signif. codes: 0 ‘***’ 0.001 ‘**’ 0.01 ‘*’ 0.05 ‘.’ 0.1 ‘ ’ 1
Residual standard error: 10.22 on 44 degrees of freedom
Multiple R-squared: 0.5229,
Adjusted R-squared: 0.5121 F-statistic: 48.23 on 1 and 44 DF, p-value: 1.378e-08
(a)
Write the least squares estimates of the slope and y-intercept for the regression of daily soil evaporation (Y) on maximum daily air temperature (X). Interpret the slope and y-
intercept, if appropriate. Note that daily soil evaporation values range from 1 to 54, and maximum daily air temperatures range from 77 to 97.
(b) Conduct a hypothesis test to determine if maximum air temperature is a significant predictor of daily soil evaporation.
(c)
State the 5 assumptions we are making in this analysis. From the output provided, are any
assumptions clearly violated? State why or why not for each assumption.
(d) If any assumptions are clearly violated, state a possible fix for the assumption violation.
Your preview ends here
Eager to read complete document? Join bartleby learn and gain access to the full version
- Access to all documents
- Unlimited textbook solutions
- 24/7 expert homework help
[10 pts] Question 2 (5.7): Several research workers associated with the Office of Highway Safety were evaluating the relationship between driving speed (MPH) and the distance a vehicle travels once brakes are applied (DIST). The results of 19 experimental tests are displayed in the following table.
(a) Determine the least-squares estimates of the slope and intercept for each of the following straight-line regressions: Y
1
(DIST) on X and Y
2
(
√
DIST
)
on X. (b) Which of the two variable pairs mentioned in part (a) seems to be better suited for straight-line regression? (c) For the variable pair Y
2
and X, test the hypothesis that the true slope is equal to 0 (use α = .01). Be sure to interpret your result. (d) Construct a 99% confidence interval for the true slope in part (c). Interpret your result (you will need to look up a critical t-value for this problem).
(e)
Estimate the mean value of Y
2
when X = 45. Find a 95% confidence interval for the mean
value of Y
2
when X = 45. Interpret your result (Note that observation 4 has X = 45).
[10 pts] Question 3 (6.11): Use the data from question 2. The results of 19 experimental tests are displayed in the following table. For the variable pair Y
2
and X:
(a)
Determine r and r
2
and interpret the results.
(b) Test H
0
: ρ = 0 vs. H
a
: ρ ≠ 0. Interpret your findings.
(c)
Find a 95% confidence interval for ρ. Interpret your results regarding the test in part (b).
[5 pts] Question 4 (6.2): Examine the five pairs of data points given in the following table.
i
1
2
3
4
5
X
i
-2
-1
0
1
2
Y
i
4
1
0
1
4
(a)
What is the mathematical relationship between X and Y?
(b) Show by computation (formula) that, for the straight-line regression of Y on X, ^
β
1
=
0.
(c)
Show by computation (formula) that r = 0. (d) Why is there apparently no relationship between X and Y, as indicated by the estimates of β
1
and ρ?
Your preview ends here
Eager to read complete document? Join bartleby learn and gain access to the full version
- Access to all documents
- Unlimited textbook solutions
- 24/7 expert homework help
[5 pts] Question 5 (7.8): (a)
Fill in the blank values in the ANOVA table below. In this analysis, there were 19 observations and only 1 predictor (this is the ANOVA table from questions 2 and 3).
Source
df
Sum of Squares
Mean Square
F
P-Value
Regression
399.351
< .0001
Residual
11.180
---------
Total
----------------
---------
(b) Using the table in part (a), perform an F-test for the significance of a straight-line regression.
[20 pts] Question 6 (R Coding Question – Simple Linear Regression):
This question involves a dataset of 32 white males over 40 years old from the town of Angina. The information provided for each person includes systolic blood pressure (SBP), body size (QUET), age (AGE), and smoking history (SMK, 0 = nonsmoker, 1 = current or former smoker). This problem will step you through how to conduct a simple linear regression analysis using R, but feel free to use another language if desired (SAS, Python, etc.).
Please note that all variable names are pretty general in this example. In practice, you should use descriptive names for your variables.
(a)
Download the .csv file “HW1Q6.csv” or “HW1Q6.xlsx” from webcourses. Make sure you place the downloaded dataset in whichever file you plan on setting your working directory to in RStudio.
Method 1: Open RStudio. Once in RStudio, go to Session -> Set Working Directory -> Choose Directory
Choose the folder which has your downloaded data. Use the function to load in the data set into a data frame: dataframe < - read.csv(file = “file_name.csv”)
Method 2: In the top right, click on “Import Dataset,” then “From Excel”.
In the top right, click on “Browse” and select the .xlsx file. A preview of the file should appear once done correctly. Click import.
To create a dataframe, run the following command:
dataframe <- data.frame(Data_Name)
For this problem, you can run the following line of code:
dataframe <- data.frame(HW1Q6)
Your preview ends here
Eager to read complete document? Join bartleby learn and gain access to the full version
- Access to all documents
- Unlimited textbook solutions
- 24/7 expert homework help
Once you do either method 1 or method 2, use the “attach” function to attach the data frame a specify to R what data frame we are using. Simply show the code you used for this part.
attach(dataframe)
(b) Create a basic scatterplot for age (AGE) vs. blood pressure (SBP) where age is on the x-
axis and blood pressure in on the y-axis. Also create a scatterplot of the ln(AGE) vs SBP.
The line below will plot a basic scatterplot between var_Y vs var_X.
plot(var_X, var_Y)
To create a scatterplot between a transformation of X vs. Y, you can apply a function to the variable X within the plot function.
This code would plot ln(X) vs. Y:
plot(log(var_X), var_Y))
This code would plot X-Squared vs. Y:
plot((var_X)^2, var_Y)
Comparing your two plots, does it appear that AGE or ln(AGE) has a better linear relationship with SBP?
(c)
Using your suggested transformation in part (b), estimate the parameters for a simple linear regression model. The code below will create a linear model between the dependent variable “var_Y” and independent variable “var_X”.
model <- lm(var_Y ~ var_X, data=dataset)
If you decide to transform a variable, you must use the I() function and then the function to transform X. For example, to transform X to ln(X):
model <- lm(var_Y ~ I(log(var_X)), data=dataset)
To see the estimates of the parameters:
summary(model)
Write the least-squares estimates of the y-intercept and slope.
(d) To see various diagnostic plots, you can run the following command:
plot(model)
The first 2 plots created are the most useful. The first plot is a residual plot, and the second plot is a normal probability plot. Does it appear all assumptions are valid?
(e)
Create an ANOVA table for your model. What is the F-statistic for the F-test for the significance of a straight-line regression?
anova(model)
(f)
Construct a 99% confidence interval for the true slope β
1
. Interpret this interval in context
of the problem. Does it appear that age is useful for predicting blood pressure? Explain.
confint(model, level=0.99)
(g) Predict the blood pressure of a 55-year-old. Construct a 95% confidence interval for the mean blood pressure of a 55-year-old and a 95% prediction interval for a 55-year-old. (Note that if you used ln(AGE), you must do ln(55) in your model rather than 55).
predicted_value <- data.frame(AGE=55) #Use if using X
predicted_value <- data.frame(SBP=AGE(55)) #Use if using ln(X)
predict(model, newdata=predicted_value, interval = 'confidence', level = 0.95)
predict(model, newdata=predicted_value, interval = 'prediction', level = 0.95)
(h) Between AGE (untransformed) and SBP, find the correlation coefficient, coefficient of determination, and a 95% confidence interval for the correlation. The following code may
be useful:
cor(var_X, var_Y)
cor.test(var_X, var_Y)
[10 pts] Question 7 (8.11):
Data on sales revenues Y, television advertising expenditures X
1
, and print media advertising expenditures X
2
for a large retailer for the period 1988–1993 are given in the following table:
Part (a): State the model for the regression of sales revenue on television advertising expenditures and print advertising expenditures.
Use the accompanying computer output to answer the following questions.
Part (b): State the estimate of the model in part (a).
Part (c): What is the estimated change in sales revenue for every $1,000 increase in television advertising expenditures (make sure to check the units of X
1
)?
Part (d): Find the R
2
-value for the regression of Y on X
1
and X
2
. Interpret your result. (Hint: R
2 = (SSY-SSE)/SSY)
Part (e): Predict the sales for a year in which $5 million is spent on TV advertising and $1 million is spent on print advertising.
[15 pts] Question 8: In 1990, Business Week magazine compiled financial data on the 1,000 companies that had the biggest impact on the U.S. economy. Data sampled from the top 500
Your preview ends here
Eager to read complete document? Join bartleby learn and gain access to the full version
- Access to all documents
- Unlimited textbook solutions
- 24/7 expert homework help
companies in Business Week’s report are presented in the following table. In addition to the company name, four variables are shown:
1990 Rank (RANK90)
o
Based on company’s market value (share price on March 16, 1990, multiplied by available common shares outstanding).
1989 Rank (RANK89)
o
Rank in 1989 compilation
P-E Ratio (PERATIO)
o
Price-to-earnings ratio, based on 1989 earnings and March 16, 1990, share price.
Yield (YIELD)
o
Annual dividend rate as a percentage of March 16, 1990, share price.
The dataset is shown here:
COMPANY RANK90 RANK89 PERATIO YIELD 1 AT&T 4 4 17 2.87 2 MERCK 7 7 19 2.54 3 BOEING 27 41 24 1.72 4 AMER. HOME PROD. 32 37 14 4.26 5 WALT DISNEY 33 42 23 0.41 6 PFIZER 46 46 14 4.10 7 MCI COMMUNICATIONS 52 72 19 0.00 8 DUNN & BRADSTREET 55 48 15 4.27 9 UNITED TELECOMM. 63 93 22 2.61 10 WARNER LAMBERT 77 91 17 2.94 11 ITT 81 57 8 2.98 12 HUMANA 162 209 15 2.62 13 SALOMON 236 172 7 2.91 14 WALGREEN 242 262 17 1.87 15 LINCOLN NATIONAL 273 274 9 4.73 16 CITIZENS UTILITIES 348 302 21 0.00 17 MNC FINANCIAL 345 398 6 5.46 18 BAUSCH & LOMB 354 391 15 1.99 19 MEDTRONIC 356 471 16 1.10 20 CIRCUIT CITY 497 514 14 0.33 Some R Output is shown below.
Let Y=Yield, X
1
= 1990 Rank, X
2
= 1989 Rank, X
3 = P-E Ratio, and X
4 = (P-E ratio)
2
.
We want to create the full model: Y
=
β
0
+
β
1
x
1
+
β
2
x
2
+
β
3
x
3
+
β
4
x
4
+
E
. Find the least-squares estimates of this model.
lm(formula = YIELD ~ RANK90 + RANK89 + PERATIO + I(PERATIO^2), data = df)
Residuals:
Min 1Q Median 3Q Max -2.2848 -0.7133 0.2326 0.6757 1.2072 Coefficients:
Estimate Std. Error t value Pr(>|t|) (Intercept) 7.840196 2.002818 3.915 0.00138 **
RANK90 -0.013758 0.008120 -1.694 0.11084 RANK89 0.008025 0.007370 1.089 0.29338 PERATIO -0.325918 0.269166 -1.211 0.24469 I(PERATIO^2) 0.002146 0.008830 0.243 0.81130 ---
Signif. codes: 0 ‘***’ 0.001 ‘**’ 0.01 ‘*’ 0.05 ‘.’ 0.1 ‘ ’ 1
Residual standard error: 1.096 on 15 degrees of freedom
Multiple R-squared: 0.625, Adjusted R-squared: 0.5249 F-statistic: 6.249 on 4 and 15 DF, p-value: 0.003635
> anova(full_model)
Analysis of Variance Table
Response: YIELD
Df Sum Sq Mean Sq F value Pr(>F) RANK90 1 1.8289 1.8289 1.5214 0.2363852 RANK89 1 0.0016 0.0016 0.0013 0.9714506 PERATIO 1 28.1467 28.1467 23.4139 0.0002168 ***
I(PERATIO^2) 1 0.0710 0.0710 0.0590 0.8112987 Residuals 15 18.0321 1.2021 ---
Signif. codes: 0 ‘***’ 0.001 ‘**’ 0.01 ‘*’ 0.05 ‘.’ 0.1 ‘ ’ 1
(a)
Conduct an overall F-test to determine if at least 1 of the variables significantly helps predict yield.
(b) Conduct a partial F-test (or the equivalent t-test) to determine if RANK90 significantly contributes to the prediction of yield, assuming it was added last.
(c)
Conduct a multiple partial F-test to determine if at least one of P-E ratio and (P-E ratio)
2
contributes significantly to the prediction of yield, assuming the variables are added to a model with RANK90 and RANK89 in it.
(d) Conduct a partial F-test to determine if P-E Ratio significantly contributes to the prediction of yield, assuming that P-E Ratio is added to a model with RANK90 and RANK89 in it.
[15 pts] Question 9 (R Coding Question – Multiple Linear Regression)
: Using the same data from question 6, answer the following questions. For all hypothesis tests in this section, make sure to include the null and alternate hypothesis, the test statistic, p-value, and conclusion.
(a)
Consider a model using age (AGE), body size (QUET), and squared body size (QUET
2
) to predict blood pressure (SBP). What is the least-squares estimates of this model. Make sure you write out the model (not just show R output).
full_model <- lm(SBP ~ AGE + QUET + I(QUET^2), data=dataframe
summary(full_model)
(b) Suppose we want to determine if QUET is useful to predict blood pressure (either QUET or QUET
2
may be useful). To conduct the multiple partial F-test, we can create a reduced model without those terms.
reduced_model <- lm(SBP ~ AGE, data=dataframe)
We can then use the anova function to conduct the hypothesis test. anova(reduced_model,full_model)
Using the output generated above, conduct the hypothesis test to determine if QUET is useful for predicting blood pressure.
(c)
Using the same method in part (b) or using the output in part (a), conduct the following 2 hypothesis tests:
1.
The first for QUET, assuming it was added last to the model.
2.
The second for QUET
2
, assuming it was added last to the model.
For both tests, state the chosen test (partial F-Test or T-test), the null/alternate hypothesis,
the p-value, rejection decision, and conclusion.
Your preview ends here
Eager to read complete document? Join bartleby learn and gain access to the full version
- Access to all documents
- Unlimited textbook solutions
- 24/7 expert homework help
Related Documents
Related Questions
1
arrow_forward
The following scatterplot shows the mean annual carbon dioxide (CO,) in parts
(CO2)
per million (ppm) measured at the top of a mountain and the mean annual air
temperature over both land and sea across the globe, in degrees Celsius (C).
Complete parts a through h on the right.
f) View the accompanying scatterplot of the residuals vs. CO2. Does the
scatterplot of the residuals vs. CO, show evidence of the violation of any
assumptions behind the regression?
16.800
A. Yes, the outlier condition is violated.
16.725
O B. Yes, the linearity and equal variance assumptions are violated.
16.650
C. Yes, the equal variance assumption is violated.
16.575
O D. No, all assumptioris are okay.
16.500
O E. Yes, all the assumptions are violated.
325.0
337.5
350.0
362.5
CO2 (ppm)
OF
Yes, the linearity assumption is violated.
his vear, What mean temperature does
arrow_forward
The toco toucan, the largest member of the toucan family, possesses the largest beak relative to body size of all birds. This exaggerated feature has received various interpretations, such as being a refined adaptation for feeding. However, the large surface area may also be an important mechanism for radiating heat (and hence cooling the bird) as outdoor temperature increases. Here are data for beak heat loss, as a percent of total body heat loss from all sources, at various temperatures in degrees Celsius. [Note: The numerical values in this problem have been modified for testing purposes.]
Temperature (oC)(oC)
15
16
17
18
19
20
21
22
23
24
25
26
27
28
29
30
Percent heat loss from beak
33
33
34
31
37
46
54
49
41
50
44
56
55
61
64
59
The equation of the least-squares regression line for predicting beak heat loss, as a percent of total body heat loss from all sources, from temperature is: (Use decimal notation. Enter the values of the intercept and slope rounded to two decimal…
arrow_forward
The toco toucan, the largest member of the toucan family, possesses the largest beak relative to body size of all birds. This exaggerated feature has received various interpretations, such as being a refined adaptation for feeding. However, the large surface area may also be an important mechanism for radiating heat (and hence cooling the bird) as outdoor temperature increases. Here are data for beak heat loss, as a percent of total body heat loss from all sources, at various temperatures in degrees Celsius. [Note: The numerical values in this problem have been modified for testing purposes.]
Temperature (oC)(oC)
15
16
17
18
19
20
21
22
23
24
25
26
27
28
29
30
Percent heat loss from beak
33
33
33
31
37
44
56
52
45
54
46
55
59
59
61
63
The equation of the least-squares regression line for predicting beak heat loss, as a percent of total body heat loss from all sources, from temperature is: (Use decimal notation. Enter the values of the intercept and slope rounded to two…
arrow_forward
The toco toucan, the largest member of the toucan family, possesses the largest beak relative to body size of all birds. This exaggerated feature has received various interpretations, such as being a refined adaptation for feeding. However, the large surface area may also be an important mechanism for radiating heat (and hence cooling the bird) as outdoor temperature increases. Here are data for beak heat loss, as a percent of total body heat loss from all sources, at various temperatures in degrees Celsius. [Note: The numerical values in this problem have been modified for testing purposes.]
Temperature (oC)(oC)
15
16
17
18
19
20
21
22
23
24
25
26
27
28
29
30
Percent heat loss from beak
34
32
33
29
38
46
58
50
46
53
44
51
57
58
59
58
The equation of the least-squares regression line for predicting beak heat loss, as a percent of total body heat loss from all sources, from temperature is: (Use decimal notation. Enter the values of the intercept and slope rounded to two…
arrow_forward
5. Oxygen demand is a term blologists use to describe the axygen needed by fish and
other aquatic organisms for survval. The Environmental Protection Agency conducted a
study of a wetland area in Marin County, Californla. In this wetland environment, the mean
oxygen demand was u=9.9 mg/L, and a= 1.7 mg/L. (Reference: EPA Report 832-R- 93-
005). Let x be a random varlable that represents oxygen demand in this wetland
environment. Assume x has a probability distribution that is approximately normal.
a. An oxygen demand below 8 mg/L Indicates that some organisms In the wetland
environment may be dying. What is the probability that the oxygen demand will fall
below 8 mg/L?
b. A high oxygen demand can also indicate trouble. An oxygen demand above 12 mg/L
may indicate an overabundance of organisms that endanger some types of plant life.
What is the probability that the oxygen demand will exceed 12 mg/L?
arrow_forward
Please help me with this problem and i needed only part d and e only please.... Very urgent
arrow_forward
An article in the journal Air and Waste (Update on Ozone Trends in California's South Coast Air
Basin, Vol. 43, 1993) investigated the ozone levels in the South Coast Air Basin of California for
the years 1976-1991. The author believes that the number of days the ozone levels exceeded 0.20
ppm (the response) depends on the seasonal meteorological index, which is the seasonal average
850-millibar Temperature (the predictor). The following table gives the data.
Year
Index
1976
1977
1978
1979
1980
1981
1982
1983
1984
1985
1986
1987
1988
1989
1990
1991
Days
91
105
106
108
88
91
58
82
81
65
61
48
61
43
33
36
16.7
17.1
18.2
18.1
17.2
18.2
16.0
17.2
18.0
17.2
16.9
17.1
18.2
17.3
17.5
16.6
(a) Construct a scatter diagram of the data.
(b) Estimate the prediction equation.
(c) Test for significance of regression.
(d) Calculate the 95% CI and PI on for a seasonal meteorological index value of 17.
Interpret these quantities.
arrow_forward
5.2
arrow_forward
Aa
Febru
The body mass index (BMI) of a person is defined to be the person's body mass
divided by the square of the person's height. The article "Influences of Parameter
Uncertainties within the ICRP 66 Respiratory Tract Model: Particle Deposition" (W.
Bolch, E. Farfan, et al., Health Physics, 2001:378-394) states that body mass index (in
kg/m2) in men aged 25-34 is lognormally distributed with parameters u = 3.215 and o = 0.157.
a.Find the mean and standard deviation BMI for men aged 25-34.
b.Find the standard deviation of BMI for men aged 25-34.
c.Find the median BMI for men aged 25-34.
d.What proportion of men aged 25-34 have a BMI less than 20?
e.Find the 80th percentile of BMI for men agėd 25
-34.
04...
Rext
田
arrow_forward
1) What is the line of best fit for this data?
a. y =-1.11x+ 11.83; r=-0.9760964904
Average Speed
(mi/h)
Time (hours)
b. y 11.83x- 1. 11; r=0.9527643586
8.5
2.5
c. y= 11.83x –- 1. 11; r=-0.9760964904
7.5
3.75
d. y =-1.11x+ 11.83; r= 0.9527643586.
6.5
4.5
6.0
5.0
5.5
5.5
5.0
6.25
4.0
6.75
3.5
8.75
r notes
MacBook Pro
*
2$
&
4
5
6
7
8
9.
%3D
deletc
{
[
Y
]
G H
J
K
L
>
?
C
V
N
M
+ ||
.. ..
B
arrow_forward
An experiment was conducted to investigate leaking current in a SOS MOSFETS device. The purpose of the experiment was to investigate how leakage current varies as the channel length changes. Four channel lengths were selected. For each channel length, five different widths were also used, and width is to be considered a nuisance factor. The data are as follows: (a) Test the hypothesis that mean leakage voltage does not depend on the channel length using a= 0.05. (b) Analyze the residuals from this experiment. Comment on the residual plots. (c) The observed leakage voltage for channel length 4 and width 5 was erroneously recorded. The correct observation is 4.0. Analyze the corrected data from this experiment. Is there evidence to conclude that mean leakage voltage increases with channel length?
arrow_forward
Please do 3c and 3d with handwritten working out
arrow_forward
NW 6.12 CORPORATE SUSTAINABILITY OF CPA FIRMS. Corporate sustainability refers to business practices designed
CORSUS
around social and environmental considerations. Refer to the Business and Society (March 2011) study on the sustainability behaviors of
CPA corporations, Exercise 2.23 (p. 59). Recall that the level of support for corporate sustainability (measured on a quantitative scale
ranging from 0 to 160 points) was obtained for each in a sample of 992 senior managers at CPA firms. Higher point values indicate a
higher level of support for sustainability. The accompanying StatCrunch printout gives a 99% confidence interval for the mean level of
support for all senior managers at CPA firms.
One sample T confidence interval:
μ: Mean of variable
99% confidence interval results:
Variable Sample Mean Std. Err. DF L. Limit U. Limit
Support 67.75504 0.85314633 991 65.553241 69.95684
a. Locate the 99% confidence interval on the printout.
b. Use the sample mean and standard deviation on the…
arrow_forward
SEE MORE QUESTIONS
Recommended textbooks for you
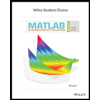
MATLAB: An Introduction with Applications
Statistics
ISBN:9781119256830
Author:Amos Gilat
Publisher:John Wiley & Sons Inc
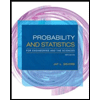
Probability and Statistics for Engineering and th...
Statistics
ISBN:9781305251809
Author:Jay L. Devore
Publisher:Cengage Learning
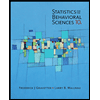
Statistics for The Behavioral Sciences (MindTap C...
Statistics
ISBN:9781305504912
Author:Frederick J Gravetter, Larry B. Wallnau
Publisher:Cengage Learning
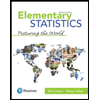
Elementary Statistics: Picturing the World (7th E...
Statistics
ISBN:9780134683416
Author:Ron Larson, Betsy Farber
Publisher:PEARSON
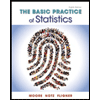
The Basic Practice of Statistics
Statistics
ISBN:9781319042578
Author:David S. Moore, William I. Notz, Michael A. Fligner
Publisher:W. H. Freeman
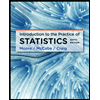
Introduction to the Practice of Statistics
Statistics
ISBN:9781319013387
Author:David S. Moore, George P. McCabe, Bruce A. Craig
Publisher:W. H. Freeman
Related Questions
- 1arrow_forwardThe following scatterplot shows the mean annual carbon dioxide (CO,) in parts (CO2) per million (ppm) measured at the top of a mountain and the mean annual air temperature over both land and sea across the globe, in degrees Celsius (C). Complete parts a through h on the right. f) View the accompanying scatterplot of the residuals vs. CO2. Does the scatterplot of the residuals vs. CO, show evidence of the violation of any assumptions behind the regression? 16.800 A. Yes, the outlier condition is violated. 16.725 O B. Yes, the linearity and equal variance assumptions are violated. 16.650 C. Yes, the equal variance assumption is violated. 16.575 O D. No, all assumptioris are okay. 16.500 O E. Yes, all the assumptions are violated. 325.0 337.5 350.0 362.5 CO2 (ppm) OF Yes, the linearity assumption is violated. his vear, What mean temperature doesarrow_forwardThe toco toucan, the largest member of the toucan family, possesses the largest beak relative to body size of all birds. This exaggerated feature has received various interpretations, such as being a refined adaptation for feeding. However, the large surface area may also be an important mechanism for radiating heat (and hence cooling the bird) as outdoor temperature increases. Here are data for beak heat loss, as a percent of total body heat loss from all sources, at various temperatures in degrees Celsius. [Note: The numerical values in this problem have been modified for testing purposes.] Temperature (oC)(oC) 15 16 17 18 19 20 21 22 23 24 25 26 27 28 29 30 Percent heat loss from beak 33 33 34 31 37 46 54 49 41 50 44 56 55 61 64 59 The equation of the least-squares regression line for predicting beak heat loss, as a percent of total body heat loss from all sources, from temperature is: (Use decimal notation. Enter the values of the intercept and slope rounded to two decimal…arrow_forward
- The toco toucan, the largest member of the toucan family, possesses the largest beak relative to body size of all birds. This exaggerated feature has received various interpretations, such as being a refined adaptation for feeding. However, the large surface area may also be an important mechanism for radiating heat (and hence cooling the bird) as outdoor temperature increases. Here are data for beak heat loss, as a percent of total body heat loss from all sources, at various temperatures in degrees Celsius. [Note: The numerical values in this problem have been modified for testing purposes.] Temperature (oC)(oC) 15 16 17 18 19 20 21 22 23 24 25 26 27 28 29 30 Percent heat loss from beak 33 33 33 31 37 44 56 52 45 54 46 55 59 59 61 63 The equation of the least-squares regression line for predicting beak heat loss, as a percent of total body heat loss from all sources, from temperature is: (Use decimal notation. Enter the values of the intercept and slope rounded to two…arrow_forwardThe toco toucan, the largest member of the toucan family, possesses the largest beak relative to body size of all birds. This exaggerated feature has received various interpretations, such as being a refined adaptation for feeding. However, the large surface area may also be an important mechanism for radiating heat (and hence cooling the bird) as outdoor temperature increases. Here are data for beak heat loss, as a percent of total body heat loss from all sources, at various temperatures in degrees Celsius. [Note: The numerical values in this problem have been modified for testing purposes.] Temperature (oC)(oC) 15 16 17 18 19 20 21 22 23 24 25 26 27 28 29 30 Percent heat loss from beak 34 32 33 29 38 46 58 50 46 53 44 51 57 58 59 58 The equation of the least-squares regression line for predicting beak heat loss, as a percent of total body heat loss from all sources, from temperature is: (Use decimal notation. Enter the values of the intercept and slope rounded to two…arrow_forward5. Oxygen demand is a term blologists use to describe the axygen needed by fish and other aquatic organisms for survval. The Environmental Protection Agency conducted a study of a wetland area in Marin County, Californla. In this wetland environment, the mean oxygen demand was u=9.9 mg/L, and a= 1.7 mg/L. (Reference: EPA Report 832-R- 93- 005). Let x be a random varlable that represents oxygen demand in this wetland environment. Assume x has a probability distribution that is approximately normal. a. An oxygen demand below 8 mg/L Indicates that some organisms In the wetland environment may be dying. What is the probability that the oxygen demand will fall below 8 mg/L? b. A high oxygen demand can also indicate trouble. An oxygen demand above 12 mg/L may indicate an overabundance of organisms that endanger some types of plant life. What is the probability that the oxygen demand will exceed 12 mg/L?arrow_forward
- Please help me with this problem and i needed only part d and e only please.... Very urgentarrow_forwardAn article in the journal Air and Waste (Update on Ozone Trends in California's South Coast Air Basin, Vol. 43, 1993) investigated the ozone levels in the South Coast Air Basin of California for the years 1976-1991. The author believes that the number of days the ozone levels exceeded 0.20 ppm (the response) depends on the seasonal meteorological index, which is the seasonal average 850-millibar Temperature (the predictor). The following table gives the data. Year Index 1976 1977 1978 1979 1980 1981 1982 1983 1984 1985 1986 1987 1988 1989 1990 1991 Days 91 105 106 108 88 91 58 82 81 65 61 48 61 43 33 36 16.7 17.1 18.2 18.1 17.2 18.2 16.0 17.2 18.0 17.2 16.9 17.1 18.2 17.3 17.5 16.6 (a) Construct a scatter diagram of the data. (b) Estimate the prediction equation. (c) Test for significance of regression. (d) Calculate the 95% CI and PI on for a seasonal meteorological index value of 17. Interpret these quantities.arrow_forward5.2arrow_forward
- Aa Febru The body mass index (BMI) of a person is defined to be the person's body mass divided by the square of the person's height. The article "Influences of Parameter Uncertainties within the ICRP 66 Respiratory Tract Model: Particle Deposition" (W. Bolch, E. Farfan, et al., Health Physics, 2001:378-394) states that body mass index (in kg/m2) in men aged 25-34 is lognormally distributed with parameters u = 3.215 and o = 0.157. a.Find the mean and standard deviation BMI for men aged 25-34. b.Find the standard deviation of BMI for men aged 25-34. c.Find the median BMI for men aged 25-34. d.What proportion of men aged 25-34 have a BMI less than 20? e.Find the 80th percentile of BMI for men agėd 25 -34. 04... Rext 田arrow_forward1) What is the line of best fit for this data? a. y =-1.11x+ 11.83; r=-0.9760964904 Average Speed (mi/h) Time (hours) b. y 11.83x- 1. 11; r=0.9527643586 8.5 2.5 c. y= 11.83x –- 1. 11; r=-0.9760964904 7.5 3.75 d. y =-1.11x+ 11.83; r= 0.9527643586. 6.5 4.5 6.0 5.0 5.5 5.5 5.0 6.25 4.0 6.75 3.5 8.75 r notes MacBook Pro * 2$ & 4 5 6 7 8 9. %3D deletc { [ Y ] G H J K L > ? C V N M + || .. .. Barrow_forwardAn experiment was conducted to investigate leaking current in a SOS MOSFETS device. The purpose of the experiment was to investigate how leakage current varies as the channel length changes. Four channel lengths were selected. For each channel length, five different widths were also used, and width is to be considered a nuisance factor. The data are as follows: (a) Test the hypothesis that mean leakage voltage does not depend on the channel length using a= 0.05. (b) Analyze the residuals from this experiment. Comment on the residual plots. (c) The observed leakage voltage for channel length 4 and width 5 was erroneously recorded. The correct observation is 4.0. Analyze the corrected data from this experiment. Is there evidence to conclude that mean leakage voltage increases with channel length?arrow_forward
arrow_back_ios
SEE MORE QUESTIONS
arrow_forward_ios
Recommended textbooks for you
- MATLAB: An Introduction with ApplicationsStatisticsISBN:9781119256830Author:Amos GilatPublisher:John Wiley & Sons IncProbability and Statistics for Engineering and th...StatisticsISBN:9781305251809Author:Jay L. DevorePublisher:Cengage LearningStatistics for The Behavioral Sciences (MindTap C...StatisticsISBN:9781305504912Author:Frederick J Gravetter, Larry B. WallnauPublisher:Cengage Learning
- Elementary Statistics: Picturing the World (7th E...StatisticsISBN:9780134683416Author:Ron Larson, Betsy FarberPublisher:PEARSONThe Basic Practice of StatisticsStatisticsISBN:9781319042578Author:David S. Moore, William I. Notz, Michael A. FlignerPublisher:W. H. FreemanIntroduction to the Practice of StatisticsStatisticsISBN:9781319013387Author:David S. Moore, George P. McCabe, Bruce A. CraigPublisher:W. H. Freeman
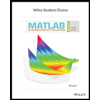
MATLAB: An Introduction with Applications
Statistics
ISBN:9781119256830
Author:Amos Gilat
Publisher:John Wiley & Sons Inc
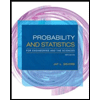
Probability and Statistics for Engineering and th...
Statistics
ISBN:9781305251809
Author:Jay L. Devore
Publisher:Cengage Learning
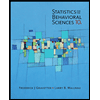
Statistics for The Behavioral Sciences (MindTap C...
Statistics
ISBN:9781305504912
Author:Frederick J Gravetter, Larry B. Wallnau
Publisher:Cengage Learning
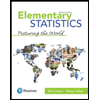
Elementary Statistics: Picturing the World (7th E...
Statistics
ISBN:9780134683416
Author:Ron Larson, Betsy Farber
Publisher:PEARSON
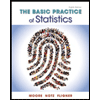
The Basic Practice of Statistics
Statistics
ISBN:9781319042578
Author:David S. Moore, William I. Notz, Michael A. Fligner
Publisher:W. H. Freeman
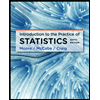
Introduction to the Practice of Statistics
Statistics
ISBN:9781319013387
Author:David S. Moore, George P. McCabe, Bruce A. Craig
Publisher:W. H. Freeman