9-2 Final Project Submission
docx
keyboard_arrow_up
School
Southern New Hampshire University *
*We aren’t endorsed by this school
Course
520
Subject
Statistics
Date
Feb 20, 2024
Type
docx
Pages
15
Uploaded by DeanRiver5511
Final Projection Submission
1
DAT 520 DECISION METHODS & MODELING
February 2. 2024
9-2 Final Project Submission
Final Projection Submission
2
I.
Introduction
The research question I chose for this project is “What in-game activity will most likely lead to winning in the National Football League?” I chose this topic because it was more relatable to my interests; I love sports and especially love football. There’s a saying that goes “Offense scores points, but defense wins championships,”; I feel this quote is relevant to the research question because it will allow me to analyze data and different in game techniques to see if there is any truth to this age old saying. “I feel the above question is very much an appropriate analysis technique-oriented question since the answer can only be aptly answered with the deep insight knowledge on understanding past data and the results will be easily determined at the end of analysis,” (SNHU, 2020). II.
Data Appraisal
The data set and information I will be using for research come from https://www.kaggle.com/datasets/kendallgillies/nflstatistics
, https://www.kaggle.com/code/blueblowfish/nfl-data-analysis
, and other articles from the Kaggle website. I will be using provided data in the PowerBI desktop also (see the
screenshot below).
Final Projection Submission
3
The “NFL Statistics” data set was originally intended to provide basic football stats and career stats for NFL players. I plan to analyze this data to find what in game activities lead to winning. The only limitations with this data set are that it also provides a lot of unnecessary data like player number, birthday, birthplace, etc. Once we eliminate what is irrelevant, the given data sets will provide a deep look at the important statistics like yards, passes, catches, sacks, wins, and more. “The identification of the column names and what it notifies and so on are really helpful for our analysis as we shouldn’t end up using the wrong columns for our research. Our analysis will fulfill the research question only when how effective we use the data and the important factors needed to be considered to complete this. A sample of the datasets is as shown, (SNHU, 2020).
Your preview ends here
Eager to read complete document? Join bartleby learn and gain access to the full version
- Access to all documents
- Unlimited textbook solutions
- 24/7 expert homework help
Final Projection Submission
4
Data set utilizes reorganize, change, or compare data in the data set and are mostly used in batch jobs. The utilities allow us to manipulate data sets to gain desired outcomes (IBM, n.d.).
III.
Techniques
The best steps to take when preparing data sets for analysis include Gather data, Discover & assess data, Cleanse & Validate data, Transform & Enrich data, and finally complete research (Talend, n.d.)
. In this situation, data has already been gathered so we come in at step 2 and begin to access data. This step is about getting to
know the data and understanding what has to be done before the data becomes useful in a particular context. The most important step in my opinion is the cleansing and validation of data. As mentioned earlier, the provided data sets do include a lot of unneeded data, in this step we will take the opportunity to get rid of the stats we do not plan to analyze.
Final Projection Submission
5
IV.
Defend & Evaluate
The reasons behind the choice of these data sets and the research question are only after the thorough validation of the data sets available. As mentioned earlier, since I’m a sports enthusiast I was very much sided towards this topic. The research question as described is asking
a query of how the games are won and what in game activities contribute to winning NFL games.
When it comes to professional study and statistics, we need data models and techniques to make people understand the hidden outliers which will take the game to a win or loss status. The National Football League (NFL) is a highly competitive and complex sporting league, where teams strive to achieve the ultimate goal of winning. Understanding the factors that contribute to winning in the NFL is of utmost importance for coaches, players, and fans alike. In recent years, researchers have begun to explore the relationship between in-game activities and their impact on the outcome of games. This project aims to investigate the question: "What in-game activity will most likely lead to winning in the NFL?" We will review top-down and bottom-up decision modeling approaches and determine which model is better suited to reach the ultimate goal of determining which activities directly correlate to winning in the NFL. These approaches provide distinct perspectives and methodologies for analyzing game activities and their relationship to winning. There are plenty of factors that can play a part in a team’s winning percentage such as weather conditions, home vs away, coaching changes and more; however, since this question specifically requests “in-game
activities”, the factors we will include in the data set are fumbles, passing yards & completions, rushing yards and attempts, receiving yards, defensive stats, and other roleplaying variables.
Final Projection Submission
6
Top-down decision modeling involves starting with a general hypothesis theory and then testing that hypothesis using specific data. In the context of the research question "What in-game
activity will most likely lead to winning in the NFL?", top-down decision modeling may not be as effective because it relies on preconceived ideas or theories about what factors are important for winning. The problem with this approach is that it may overlook or underestimate the importance of certain factors that were not initially considered in the hypothesis. It may also fail to capture the complexity and interplay of different variables in determining the outcome of a game. By starting with a preconceived theory, researchers may miss out on valuable insights and patterns that can only be derived by analyzing specific data from individual game activities.
Bottom-up decision modeling involves collecting and analyzing data from individual components or factors and then integrating them to form a comprehensive understanding. In the context of this research question, bottom-up decision modeling would involve gathering data on various game activities (such as passing yards, rushing yards, turnovers, etc.) and then analyzing each activity's impact on winning.
Your preview ends here
Eager to read complete document? Join bartleby learn and gain access to the full version
- Access to all documents
- Unlimited textbook solutions
- 24/7 expert homework help
Final Projection Submission
7
By analyzing historical data from previous NFL seasons, we can determine which activities are most strongly correlated with winning. This would involve analyzing how teams perform in games where they have high passing yards, low turnovers, or a significant time of possession advantage. By examining specific game statistics, we can identify patterns and factors that contribute to winning in the NFL. Using bottom-up decision modeling allows us to derive insights and draw conclusions based on the data you have collected, providing a more evidence-based approach to answering your research question.
Final Projection Submission
8
I would choose bottom-up decision modeling for this research question because it allows us to derive insights and draw conclusions based on the collected data. Few things to consider with researching NFL in-game statistics in regard to ethical and legal concerns. Injuries are an in-game activity that can affect how teams perform and win. Injuries to key players can lead to quick downfalls for a team, however we must remember to follow HIPPA guidelines when releasing health related player information. With sports betting becoming more and more popular, the legality of studying these stats and activities can be compromised if not following the proper course of action. Studying these topics for monetary gain would defy legal and ethical standards. Bottom-up models are characterized by and adaptability in responding to changing circumstances. They are built on the idea of empowering individual workers to make decisions and act based on their unique perspectives and expertise. This approach allows for quicker
Final Projection Submission
9
responses to emerging issues and greater innovation in problem-solving. Overall, the agility of a bottom-up model lies in its ability to harness the collective intelligence and creativity of individuals to drive innovation and improve decision-making processes. By incorporating this approach into future models, organizations can better adapt to complex and dynamic environments while promoting ethical considerations and bias mitigation strategies.
One example of a bottom-up model in action is the use of crowdsourcing in disaster response. In this approach, individuals on the ground can report information about the situation in real-time, allowing for a more accurate and timely response from aid organizations. This agile and decentralized approach has proven to be effective in improving the efficiency and effectiveness of disaster relief efforts. In terms of future applications, researchers can further explore the potential of bottom-up models in areas such as healthcare, education, and social services. By empowering frontline workers and individuals to contribute their insights and ideas, organizations can benefit from a more diverse range of perspectives and solutions.
The implementation process of the bottom-up model results involves several key steps:
1. Analysis of results: Once the collective intelligence and creativity of individuals have been harnessed to generate insights and recommendations, these results need to be analyzed to identify
key trends, patterns, and implications.
2. Decision-making: Based on the analysis of the results, organizational leaders need to make strategic decisions on how to implement the recommendations and drive change within the organization.
Your preview ends here
Eager to read complete document? Join bartleby learn and gain access to the full version
- Access to all documents
- Unlimited textbook solutions
- 24/7 expert homework help
Final Projection Submission
10
3. Action planning: Once decisions have been made, an action plan needs to be developed outlining specific steps, timelines, responsibilities, and resources required to implement the recommendations effectively.
4. Communication and alignment: It is essential to communicate the plan and rationale behind it to all stakeholders within the organization to ensure alignment and buy-in. This may involve engaging employees, customers, and other external partners in the process.
5. Implementation and monitoring: The action plan is then put into action, with progress monitored regularly to ensure that objectives are being met and any necessary adjustments are made as needed.
6. Evaluation and feedback: Finally, the results of the implementation process need to be evaluated to gauge the effectiveness of the changes made and gather feedback for continuous improvement. By following these steps, organizations can effectively translate the insights generated by the bottom-up model into concrete actions that drive innovation and improve decision-making processes. The steps taken to build the decision tree model include: 1.
Data collection and preprocessing: Gather relevant for the decision tree model, it is cleaned and properly.
2.
Feature selection: the most important features to include in the decision tree model based on their relevance to the problem at hand.
3.
Model training: Train decision tree model using the selected features and algorithms to predict outcomes accurately.
Final Projection Submission
11
4.
Model evaluation: Evaluate the performance of the decision tree model using metrics such as accuracy, precision, recall, and F1 score.
5.
Addressing potential complications: Identify any issues or challenges that may arise during the implementation of the decision tree model, such as overfitting, underfitting, or im, and take steps to address them accordingly.
6.
Interpretation and validation: Interpret the results of the decision tree model to understand
how it makes decisions and validate its effectiveness through testing and validation processes.
By following these steps, you can build a robust decision tree model and effectively address potential complications to ensure accurate and reliable results for the research question. In conclusion, utilizing a bottom-up modeling approach to determine the in-game activities that lead to winning in the NFL provides valuable insights for coaching strategies, player development programs, and overall game understanding. By analyzing macro-level strategies and micro-level actions, patterns and correlations can be identified to inform evidence-based decision-making. This research has the potential to contribute significantly to the success of teams in the NFL and drive improvements in performance.
V.
Decision Tree Model
A. Summarize My research question “What in game activities most likely contribute to winning in the NFL?” has proven difficult for me to get a solid decision tree model formed. This question calls for a bottom-up modeling style, meaning I need to use a decision tree chart for my model. I have been continuously struggling to get a chart to even establish for me.
My target variable is set as ‘Outcome’ from the NFL game logs and this represents
Final Projection Submission
12
whether the selected team Won or Lost. Input variables have included: Touchdown (TD) passes, rushing TD, pass yards, rush yards, and receiving yards. I used several different combinations of these input variables to manipulate the system enough to create the decision chart, these attempts remained unsuccessful. I initially thought the data set I was using may be too large and that was the reasoning for my constant error with the decision tree, so I tried minimizing my data set and input variables. I was able to get a small chart created this way, so I made some progress, however I need to continue to adjust and add more variables to see if I am able to establish a larger and more accurate chart. The image shown below is the model I ended up with this week.
Your preview ends here
Eager to read complete document? Join bartleby learn and gain access to the full version
- Access to all documents
- Unlimited textbook solutions
- 24/7 expert homework help
Final Projection Submission
13
B. Evaluate
The current results clearly are not very reasonable with the given input variables. The chart shows that the team with the most passing completions wins the game 59% of the time. While the result seems accurate and I can agree that pass completions and touchdowns contribute to winning, I cannot get fully reasonable and accurate results without other input variables in the chart.
C. Leverage
Tools I plan to use to determine the accuracy of my decision model include PowerBI, NFL Stats, and the game logs provided for data sets. As stated above, the current model is
accurate yet does not give enough input variables to conclude feasible research for the question at hand.
Final Projection Submission
14
D. Identify As it stands, my model is currently missing several elements since I am still not able to get an accurate chart loaded. I am continuing to mess with the system and input variables. Changing the target variable would not be beneficial, since the main goal here is to determine what activities lead to winning, Win or Lose would be the variable. E. Outline
Common errors associated with creating a bottom-up decision tree model include: Insufficient data, Overfitting, Incorrect assumptions, Lack of validation, and disregarding
model interpretation. To avoid insufficient data, we need to ensure data sets are relevant & accurate. To avoid overfitting the data set needs to be simplified to keep the model from becoming overly complex. It is essential to split the data into training and test sets to evaluate the model’s performance and accuracy.
Ultimately, I never was able to generate a full decision tree model that would give me all the outcomes I seek for my research purposes. The in-game activity most likely leading to winning in the NFL is passing and rushing touchdowns, each of these items yielded the most common outcome of winning the game.
Final Projection Submission
15
REFERENCES
IBM documentation
. (n.d.). https://www.ibm.com/docs/en/zos-basic-skills?topic=programs-data-
set-utilities
NFL statistics
. (2017, June 9). Kaggle. https://www.kaggle.com/datasets/kendallgillies/nflstatistics/data
SNHU. (2020). DAT 520 Milestone 1
. CourseHero. https://www.coursehero.com/u/file/71426547/DAT-520-Milestone-Onedocx/?
userType=student
Talend. (n.d.). What is Data Preparation? Processes and Example
. Talend - a Leader in Data Integration & Data Integrity. https://www.talend.com/resources/what-is-data-preparation/
Your preview ends here
Eager to read complete document? Join bartleby learn and gain access to the full version
- Access to all documents
- Unlimited textbook solutions
- 24/7 expert homework help
Related Documents
Recommended textbooks for you
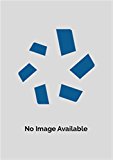
Big Ideas Math A Bridge To Success Algebra 1: Stu...
Algebra
ISBN:9781680331141
Author:HOUGHTON MIFFLIN HARCOURT
Publisher:Houghton Mifflin Harcourt
Recommended textbooks for you
- Big Ideas Math A Bridge To Success Algebra 1: Stu...AlgebraISBN:9781680331141Author:HOUGHTON MIFFLIN HARCOURTPublisher:Houghton Mifflin Harcourt
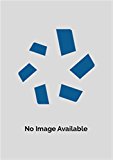
Big Ideas Math A Bridge To Success Algebra 1: Stu...
Algebra
ISBN:9781680331141
Author:HOUGHTON MIFFLIN HARCOURT
Publisher:Houghton Mifflin Harcourt