Condo Sales Case Study
docx
keyboard_arrow_up
School
University Of Charleston *
*We aren’t endorsed by this school
Course
LAW2
Subject
Statistics
Date
Feb 20, 2024
Type
docx
Pages
10
Uploaded by dharloff11
1
Condo Sales Case
Dylan Harloff
“The Condo Sales Case”
STAT 4300 – Data Driven Decision Making
April 30
th
, 2023
Professor Benestad
2
Condo Sales Case
Introduction In this case study, we delve into an examination of key variables that influence the selling
price of oceanfront condominium units. The focus is on a contemporary condominium development comprising two interconnected eight-story buildings. Numerous factors contribute to the pricing of each individual unit, encompassing aspects such as the actual sale price, floor elevation, proximity to the elevator, ocean view, whether it is an end unit or not, the inclusion of furniture, and whether the unit was sold through auction. The primary aim of this case study revolves around formulating an optimal regression model that accurately forecasts the sale price of condominium units sold at auction.
Variables
In our multiple regression model, we incorporate various factors to analyze their impact on the sale price of each condominium unit. These factors encompass: Price, which represents the actual sale price of each unit, measured in hundreds of dollars; Floor, denoting the floor height or specific location of each unit on a scale ranging from 1 to 8; Distance, indicating the proximity of each unit to the nearest elevator; View, a binary variable where 1 signifies a unit with an ocean view and 0 denotes units without an ocean view; End Unit, a binary variable where 1 designates units located at the ends, specifically those ending in 11, and 0 represents units that are not end units; Furnish, a binary variable where 1 indicates units that are furnished and 0 signifies unfurnished units; Auction, a binary variable where 1 denotes units sold at auction and 0 represents units sold through other means. These variables collectively contribute to our comprehensive regression model for predicting condominium unit sale prices.
3
Condo Sales Case
Relationship Between Dependent and Independent Variable
In preparation for running a multiple regression analysis, there are a few crucial steps that
must be taken beforehand. As previously mentioned, the first steps involve identifying potential dependent and independent variables and collecting relevant data for each. The subsequent step is to examine the relationship between each dependent variable and the independent variables using scatterplots and correlation analysis. If an independent variable is not correlated with a dependent variable, then it should not be included in the multiple regression model. To be
Your preview ends here
Eager to read complete document? Join bartleby learn and gain access to the full version
- Access to all documents
- Unlimited textbook solutions
- 24/7 expert homework help
4
Condo Sales Case
included in the regression model, the dependent and independent variables must display a strong linear relationship and should be correlated. In this case study, the scatterplot analysis and correlation calculations demonstrate that the sale price of each condo does not exhibit a particularly strong linear relationship with each independent variable. However, the distance and view variables show a small linear relationship with the sale price of each condo. The floor variable displays a negative correlation, which is generally not ideal for inclusion in the regression, but further analysis may be necessary due to its categorical nature. The three dummy variables of end, furnish, and auction are challenging to test for multicollinearity with the dependent variable of sale price. Dummy variables often result in multicollinearity with the dependent variable, making it difficult to determine whether certain dummy variables should be included in the multiple regression. As of now, all the independent variables will be included in the regression, with further analysis required to determine their impact on the sale price of each condo.
Relationship between Independent Variables
Next, it is important to examine the relationships among the independent variables by employing scatterplots and correlation analysis. To avoid an excessive number of scatterplots, the correlations between each independent variable are presented in the provided table. The objective is to identify whether the scatterplots display a robust linear relationship or a high correlation close to 1, as this indicates potential multicollinearity. It is crucial to avoid including
5
Condo Sales Case
variables that exhibit strong correlations, as they can introduce problems into the regression model. Upon inspecting the Excel image, it becomes evident that there are no variables demonstrating multicollinearity concerns with correlations near 1. The floor and auction variables display a relatively moderate correlation of 0.4608, which stands among the highest correlations observed. Nevertheless, this correlation value is not notably high and falls far from 1, suggesting that the floor and auction variables are not considered multicollinear. Consequently, none of the independent variables exhibit multicollinearity issues, thereby allowing for the inclusion of all independent variables in the multiple regression model based on this analysis.
Multiple Regression Models
The final step of the multiple regression process involves selecting the most suitable model using independent variables that are not redundant. Based on the previous stages, there are
no apparent variables that should be disregarded when utilizing the multiple regression model. The Excel image above illustrates the summary results of the multiple regression. In the "Regression Statistics" section, an R-Square value of 59.76% is observed, with an adjusted R-
Square of 58.56%. This signifies that the independent variables account for 58.56% of the variation in the dependent variable, namely the sale price. The relatively high R-Square score
6
Condo Sales Case
suggests that this model performs reasonably well in predicting the sale price of each condominium unit. While the standard error of the mean is relatively high at 21.8163, it is not a major cause for concern. The F-test result indicates the model's significance, with a significantly low p-value of 2.26E-37. However, the high F-test value raises the possibility of potential combined significance issues among the independent variables.
To further analyze the independent variables, we assess their individual impacts on the model. The four highlighted independent variables—distance, view, end, furnish, and auction—
are all statistically significant, with p-values below the alpha level of 0.05. The only independent variable in this model that lacks statistical significance is the floor variable, with a p-value of 0.1303, exceeding the alpha value. Considering the inclusion of factors in the regression model, the floor variable does not appear to exhibit a significant relationship with the dependent variable, i.e., the sales price.
After observing the absence of correlation between the floor variable and the dependent variable in the previous step, it was decided to exclude the floor variable from the multiple regression model. This decision was further reinforced by its lack of statistical significance in the
model that included all the independent variables. In the provided multiple regression summary
Your preview ends here
Eager to read complete document? Join bartleby learn and gain access to the full version
- Access to all documents
- Unlimited textbook solutions
- 24/7 expert homework help
7
Condo Sales Case
output, all the independent variables are now statistically significant, adhering to an alpha value of 0.05. However, both the R-Square and adjusted R-Square values are marginally lower than those obtained in the previous multiple regression model, which encompassed all the independent variables. The adjusted R-Square for the second regression stands at 58.29%, slightly higher than the adjusted R-Square result of 58.56% achieved in the regression that considered all the independent variables. Consequently, it appears that the first regression, which
incorporated all the factors, slightly outperforms in its ability to forecast the sale price of each condominium unit.
The multiple regression performed above excludes the floor and auction independent variables. The floor variable did not demonstrate statistical significance in the initial regression model that incorporated all the independent variables, thereby leading to its exclusion. Additionally, the auction variable, which exhibited the most substantial negative correlation with
the dependent variable of selling price and a strong association with the floor variable, was omitted to mitigate potential multicollinearity concerns.
In the Excel figure presented, the adjusted R-Square for the regression stands at 41.86%, which is lower than the adjusted R-Square of 58.56% obtained from the multiple regression
8
Condo Sales Case
involving all the independent variables. However, even after excluding the floor and auction independent variables, there is no noticeable improvement in model performance compared to the other two models. The modified R-Square values continue to decline when additional independent variables are removed, as evident in the Excel spreadsheet. Thus, the multiple regression model encompassing all independent variables remains the most accurate in forecasting the sale price of a condominium unit sold at auction.
Interpretation
The most suitable model for predicting the sale price of a condo unit is the multiple regression model. The coefficients in the model hold significant meanings. If all the independent variables are set to 0, the sale price of a condo unit would be $186.99 (in hundreds of dollars), as indicated by the intercept coefficient. This $186.99 can be considered the base price for a condo unit without any specified components from the regression.
9
Condo Sales Case
The floor variable coefficient of -1.16 suggests that the sale price of a condo unit would decrease by 1.16 units if there is an increase in floor level. This interpretation aligns with the notion that individuals may prefer lower floors for easier access to amenities such as the beach and pool. However, the floor variable itself has limited significance.
The distance variable implies that for every unit increase in the distance between a condo unit and the elevator, the sale price of the condo unit would rise by 0.92 units. This interpretation
may be debatable, as it contradicts the common belief that a condo unit's value increases if it is closer to the elevator. The assumption here is that increased distance provides more privacy and less foot traffic, thus boosting the value. The view variable, being a dummy variable, suggests that if a condo unit has an ocean view, the sale price would increase by 48.25 units. This interpretation is highly reasonable, as ocean views are generally desirable and can significantly enhance the value of the condo unit.
The end variable, another dummy variable, indicates that if a condo unit is an end unit ending at room number 11, the sale price would decrease by 23.63 units. This interpretation also aligns with the expectation that end units lacking ocean views and instead facing another building would have lower value compared to units with better views. The furnish variable, as a dummy variable, suggests that if a condo unit is furnished, the sale price would rise by 6.95 units. This interpretation is logical, as a fully furnished unit saves potential buyers the hassle of purchasing furnishings separately, thereby increasing the overall value of the property.
Lastly, the auction variable, being a dummy variable, indicates that if a condo unit was sold at auction, the sale price would decrease by 27.97 units. It is plausible that the condo unit was initially listed at a low starting bid to attract potential buyers, but this strategy may have resulted in a lower final sale price. Therefore, this explanation appears reasonable. Overall, these interpretations provide insights into how each independent variable influences the sale price of a condo unit within the multiple regression model.
Conclusion
The interpretations of the independent variables in the multiple regression model seem plausible and logical. Furthermore, when comparing the R-Square and adjusted R-Square values among the different regression models, it is evident that the model incorporating all the independent variables yields the highest values. This suggests that this multiple regression model
Your preview ends here
Eager to read complete document? Join bartleby learn and gain access to the full version
- Access to all documents
- Unlimited textbook solutions
- 24/7 expert homework help
10
Condo Sales Case
is the most effective in accurately predicting the sale price of condominium units. Each independent variable plays a significant role in the model and is essential for achieving accurate predictions of the sale price for these condo units.
References
Pearson: Chapter 12 – Multiple Regression and Model Building
Related Documents
Related Questions
Case study
The board of a large Australian investment bank of 7,200 employees (1,800 females and 5,400 males)
has received media criticism over its poor record on employee work-life balance. It is looking to implement
a range of workforce strategies and wants to survey its employee base to see how that might best improve.
The company is headquartered in Melbourne, with equally-sized offices in Sydney, Brisbane and Perth.
Some variables of interest in the proposed survey include:
Variable
Department ID
Age
Number of dependants
Desire to work-from-home
(WFH) preference
Description
Randomly generated 5-digit number for each of the company's 42
departments, which also identifies the department's location
In years
Scale from 'Highly undesirable' to 'Highly desirable'
a) For each of the variables mentioned in the table above, determine whether they are nominal,
ordinal or quantitative. Furthermore, for variables that are quantitative, also determine if the
variable is likely to be discrete…
arrow_forward
Chi-square tost of indopondance
Eapatel
Westminster Financial has released a summary of investment package information from the past fiscal year. During this time, Westminster provided a choice
among twelve pre-designed investment packages. Westminster classified the packages into three categories according to riskiness. We're interested in the
possible relationship between the age of an imvestor and the riskiness of the investment package she chose. So, there are two variables under consideration:
age of Westminster investor ("under 35", "35-49", or "50+") and riskiness of investment choice ("risky", "mnderate/mixed", or "conservative").
The contingency table below gives a summary of the information released by Westminster regarding age and riskiness of investment package for a random
sample of 150 investors. Each cell of the table contains three numbers: the first number is the observed cell frequency fo): the second number is the expected
cell frequency e) under the assumption that…
arrow_forward
The business problem facing the director of broadcasting operations for a television station was the issue of standby hours (i.e. hours in which unionized graphic artists at the station are paid but are not actually involved in any activity) and what
factors were related to standby hours. A study of standby hours was conducted for 26 weeks. The variables in the study are described below and the data from the study are shown in the accompanying table. Complete parts a throughg below.
Standby hours (Y)-Total number of standby hours in a week
Total staff present (X,)-Weekly total of people-days
Remote hours (X,)-Number of hours worked by employees off-site
E Click the icon to view the data table.
Standby hours
a. State the multiple regression equation.
Standby
Hours (Y) Present (X,) Hours (X2)
247
Total Staff
Remote
Ý, =- 328.6 + (1.8) x, + (-
328.6)X (Round to one decimal place as needed.)
338
333
358
372
337
414
177
600
656
269
211
631
196
135
195
118
116
147
528
289
419
334
382
293
399…
arrow_forward
Help me please
arrow_forward
Hardev
arrow_forward
Classification of Data
Identify the individuals and give the variables under the following:
1.
You want to study about the people who climbed Mt. Everest.
2.
The Department of Agriculture wishes to conduct a study about the pineapples in Tagaytay.
arrow_forward
1
2
3
4
5
6
7
8
9
_0
1
2
3
_4
5
6
_7
8
9
20
21
22
23
24
25
26
27
28
29
30
31
52
53
4
35
36
37
38
39
10
11
12
13
14
15
16
47
18
19
50
51
52
53
54
A
Income
($1000s)
54
30
32
50
31
55
37
40
66
51
25
48
27
33
65
63
42
21
44
37
62
21
55
42
41
54
30
48
34
67
50
67
55
52
62
64
22
29
39
35
39
54
23
27
26
61
30
22
46
66
B
Household
Size
3
2
4
5
2
2
1
2
4
3
3
4
1
2
3
4
6
2
1
5
6
3
7
2
7
6
1
2
5
4
2
5
6
2
3
2
3
4
2
1
4
3
6
2
7
2
AUANN
2
4
5
4
с
Amount
Charged ($)
4,016
3,159
5,100
4,742
1,864
4,070
2,731
3,348
4,764
4,110
4,208
4,219
2,477
2,514
4,214
4,965
4,412
2,448
2,995
4,171
5,678
3,623
5,301
3,020
4,828
5,573
2,583
3,866
3,586
5,037
3,605
5,345
5,370
3,890
4,705
4,157
3,579
3,890
2,972
3,121
4,183
3,730
4,127
2,921
4,603
4,273
3,067
3,074
4,820
5,149
D
E
F
G
H
I
J
K
arrow_forward
Please fill in the blanks and select the correct answers over the image provided. Thank you
arrow_forward
Management of a major company has made the following assumptions regarding their consumer profile.
Over 60% of all shoppers are women
Less than 40% of our customer primarily make purchases through online resources
The average age of the purchasers of product B is below 45 years.
The average weekly purchase of the top selling product A exceeds $50.
The company hired market researchers to determine the validity of their consumers' demographic assumptions. The researchers conducted survey and focus groups to
evaluate the purchasing pattern of the company's current consumers. In a survey of 400 consumers, 252 respondents were women and 148yrespondents indicated they
purchase the company's products online. The average age of the respondents was 44 years with standard deviation of 10 years. The average weekly purchase of top
selling products A and B by the respondents were $52 with standard deviation of $10.5 and $60 with standard deviation of $22.0 respectively.
Determine which of the…
arrow_forward
MINDTAP
Connect - Class:.
H Mail - Sanchez, B.
nework 7 (Chapter 14) - Part A
Q Search this COL
The Wall Street Journal asked Concur Technologies, Inc., an expense managèment company, to examine data from 8.3 million expense reports to provide insights regarding business travel
expenses. Their analysis of the data showed that New York was the most expensive city. The following table shows the average daily hotel room rate (2) and the average amount spent on
entertainment (y) for a random sample of 9 of the 25 most-visited U.S. cities. These data lead to the estimated regression equation û = 17.49 + 1.0334x. For these data SSE = 1541.4.
Click on the datafile logo to reference the data. Use Table 1 of Appendix B.
%3D
%3D
DATA file
Room Rate
Entertainment
City
($)
($)
Boston
148
161
Denver
96
105
Nashville
91
101
New Orleans
110
142
Phoenix
90
100
San Diego
102
120
San Francisco
136
167
90
140
San Jose
82
98
Tampa
a. Predict the amount spent on entertainment for a particular city that has…
arrow_forward
SEE MORE QUESTIONS
Recommended textbooks for you
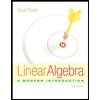
Linear Algebra: A Modern Introduction
Algebra
ISBN:9781285463247
Author:David Poole
Publisher:Cengage Learning
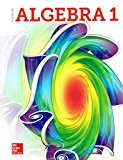
Glencoe Algebra 1, Student Edition, 9780079039897...
Algebra
ISBN:9780079039897
Author:Carter
Publisher:McGraw Hill
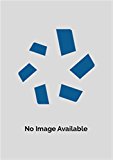
Big Ideas Math A Bridge To Success Algebra 1: Stu...
Algebra
ISBN:9781680331141
Author:HOUGHTON MIFFLIN HARCOURT
Publisher:Houghton Mifflin Harcourt
Related Questions
- Case study The board of a large Australian investment bank of 7,200 employees (1,800 females and 5,400 males) has received media criticism over its poor record on employee work-life balance. It is looking to implement a range of workforce strategies and wants to survey its employee base to see how that might best improve. The company is headquartered in Melbourne, with equally-sized offices in Sydney, Brisbane and Perth. Some variables of interest in the proposed survey include: Variable Department ID Age Number of dependants Desire to work-from-home (WFH) preference Description Randomly generated 5-digit number for each of the company's 42 departments, which also identifies the department's location In years Scale from 'Highly undesirable' to 'Highly desirable' a) For each of the variables mentioned in the table above, determine whether they are nominal, ordinal or quantitative. Furthermore, for variables that are quantitative, also determine if the variable is likely to be discrete…arrow_forwardChi-square tost of indopondance Eapatel Westminster Financial has released a summary of investment package information from the past fiscal year. During this time, Westminster provided a choice among twelve pre-designed investment packages. Westminster classified the packages into three categories according to riskiness. We're interested in the possible relationship between the age of an imvestor and the riskiness of the investment package she chose. So, there are two variables under consideration: age of Westminster investor ("under 35", "35-49", or "50+") and riskiness of investment choice ("risky", "mnderate/mixed", or "conservative"). The contingency table below gives a summary of the information released by Westminster regarding age and riskiness of investment package for a random sample of 150 investors. Each cell of the table contains three numbers: the first number is the observed cell frequency fo): the second number is the expected cell frequency e) under the assumption that…arrow_forwardThe business problem facing the director of broadcasting operations for a television station was the issue of standby hours (i.e. hours in which unionized graphic artists at the station are paid but are not actually involved in any activity) and what factors were related to standby hours. A study of standby hours was conducted for 26 weeks. The variables in the study are described below and the data from the study are shown in the accompanying table. Complete parts a throughg below. Standby hours (Y)-Total number of standby hours in a week Total staff present (X,)-Weekly total of people-days Remote hours (X,)-Number of hours worked by employees off-site E Click the icon to view the data table. Standby hours a. State the multiple regression equation. Standby Hours (Y) Present (X,) Hours (X2) 247 Total Staff Remote Ý, =- 328.6 + (1.8) x, + (- 328.6)X (Round to one decimal place as needed.) 338 333 358 372 337 414 177 600 656 269 211 631 196 135 195 118 116 147 528 289 419 334 382 293 399…arrow_forward
- Help me pleasearrow_forwardHardevarrow_forwardClassification of Data Identify the individuals and give the variables under the following: 1. You want to study about the people who climbed Mt. Everest. 2. The Department of Agriculture wishes to conduct a study about the pineapples in Tagaytay.arrow_forward
- 1 2 3 4 5 6 7 8 9 _0 1 2 3 _4 5 6 _7 8 9 20 21 22 23 24 25 26 27 28 29 30 31 52 53 4 35 36 37 38 39 10 11 12 13 14 15 16 47 18 19 50 51 52 53 54 A Income ($1000s) 54 30 32 50 31 55 37 40 66 51 25 48 27 33 65 63 42 21 44 37 62 21 55 42 41 54 30 48 34 67 50 67 55 52 62 64 22 29 39 35 39 54 23 27 26 61 30 22 46 66 B Household Size 3 2 4 5 2 2 1 2 4 3 3 4 1 2 3 4 6 2 1 5 6 3 7 2 7 6 1 2 5 4 2 5 6 2 3 2 3 4 2 1 4 3 6 2 7 2 AUANN 2 4 5 4 с Amount Charged ($) 4,016 3,159 5,100 4,742 1,864 4,070 2,731 3,348 4,764 4,110 4,208 4,219 2,477 2,514 4,214 4,965 4,412 2,448 2,995 4,171 5,678 3,623 5,301 3,020 4,828 5,573 2,583 3,866 3,586 5,037 3,605 5,345 5,370 3,890 4,705 4,157 3,579 3,890 2,972 3,121 4,183 3,730 4,127 2,921 4,603 4,273 3,067 3,074 4,820 5,149 D E F G H I J Karrow_forwardPlease fill in the blanks and select the correct answers over the image provided. Thank youarrow_forwardManagement of a major company has made the following assumptions regarding their consumer profile. Over 60% of all shoppers are women Less than 40% of our customer primarily make purchases through online resources The average age of the purchasers of product B is below 45 years. The average weekly purchase of the top selling product A exceeds $50. The company hired market researchers to determine the validity of their consumers' demographic assumptions. The researchers conducted survey and focus groups to evaluate the purchasing pattern of the company's current consumers. In a survey of 400 consumers, 252 respondents were women and 148yrespondents indicated they purchase the company's products online. The average age of the respondents was 44 years with standard deviation of 10 years. The average weekly purchase of top selling products A and B by the respondents were $52 with standard deviation of $10.5 and $60 with standard deviation of $22.0 respectively. Determine which of the…arrow_forward
arrow_back_ios
arrow_forward_ios
Recommended textbooks for you
- Linear Algebra: A Modern IntroductionAlgebraISBN:9781285463247Author:David PoolePublisher:Cengage LearningGlencoe Algebra 1, Student Edition, 9780079039897...AlgebraISBN:9780079039897Author:CarterPublisher:McGraw HillBig Ideas Math A Bridge To Success Algebra 1: Stu...AlgebraISBN:9781680331141Author:HOUGHTON MIFFLIN HARCOURTPublisher:Houghton Mifflin Harcourt
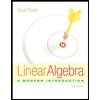
Linear Algebra: A Modern Introduction
Algebra
ISBN:9781285463247
Author:David Poole
Publisher:Cengage Learning
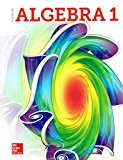
Glencoe Algebra 1, Student Edition, 9780079039897...
Algebra
ISBN:9780079039897
Author:Carter
Publisher:McGraw Hill
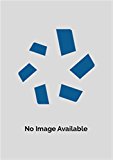
Big Ideas Math A Bridge To Success Algebra 1: Stu...
Algebra
ISBN:9781680331141
Author:HOUGHTON MIFFLIN HARCOURT
Publisher:Houghton Mifflin Harcourt