Assignment 2 STAT1070
pdf
keyboard_arrow_up
School
The University of Newcastle *
*We aren’t endorsed by this school
Course
1070
Subject
Statistics
Date
May 22, 2024
Type
Pages
9
Uploaded by ChancellorPolarBearMaster1054
STAT1070 – Assignment 2 C3380626 – Jordan Proctor Jones QUESTION 1 Using appropriate graphs and statistics, describe the relationship between distance lived from campus and the type of enrolment. From the histogram and boxplot in Figure 1, the distribution of satisfaction appears left skewed in both enrolments. The Centre descriptives shows a mean for Online of a mean of 17.1 and median 13.1 and face-to-face a mean of 10.0 and median of 6.20, further supporting the shape to be left-
skewed. The Spread for online descriptive showed a standard deviation of 16.4 and IQR of 18.3, for face-to-face a standard deviation of 12.4 and IQR of 8.03. Shown in both diagram there appears to be multiple outliers in the upper end of the boxplot both for online and face-to-face. Figure 1: Side-by-side boxplot/histogram and descriptive statistics for Question 1a Are the online and face-to-face samples paired or independent? Write a sentence justifying your choice. Both online and face-to-face variable are not associated with one another as there sample of 50 students are 2 different samples, resulting in being an independent sample. Is there evidence that the average distance lived from campus is different for students enrolled in online classes and students enrolled in face-to-face classes? Conduct the appropriate test in Jamovi and include relevant output. Be sure to define any parameters you use, state the null and alternative hypotheses, observed test statistic, null distribution, p-value, decision and provide an appropriate conclusion in plain language. Let µ
d
be the true average difference in the distance people lived from campus who are enrolled in online and face-to-face classes.
The hypotheses are: 𝐻𝐻
0
∶
𝜇𝜇
𝑑𝑑
= 0
𝐻𝐻
𝐴𝐴
∶
𝜇𝜇
𝑑𝑑
≠
0
The test statistic is t = 2.44 (see statistic column in Figure 2) Null distribution: If H
0 is true t ~ t
98
, where the degrees of freedom is determined as n – 1 = 98 or observing the df
column of Figure 2. The p-value given by is: 2
𝑃𝑃
(
𝑡𝑡
98
≥
2.44)
0.017
Conclusion: Due the p-value being small, we reject the H
0
and conclude that there is strong evidence to suggest there is a difference in the average of those who study online and face-to-face and the distance they live from campus. Independent Samples T-Test
95% Confidence Interval Statistic df p Mean difference SE difference Lower Upper Distance
Student's t 2.44 ᵃ
98.0 0.017 7.08 2.90 1.32
12.8
Figure 2: Jamovi output for independent t-test for different distances of students enrolled in face-to-face and online. Report the 95% confidence interval using Jamovi for the difference in average distance lived from campus. Write a sentence interpreting this interval in plain language. From Figure 2, a 95% confidence interval for µ
d
is (1.32, 12.8). This means that from the data we can be 95% confident that the average percentage of student who enrolled in face-to-face and online is between 1.32% and 12.8%
Does this confidence interval from (d) support the decision made in part (c)? The confidence interval does not support the decision made in part c, as it does not contain 0, which was the claimed value of µ
d
under the null hypothesis and therefore the decision to reject the null hypothesis was aligned. What are the assumptions of your analyses in parts (c) and (d)? Are these assumptions met? Justify why or why not for each assumption, with appropriate references to Jamovi output where needed. For both the independent t-test and the confident interval for µ
d
we assume that: -
The 2 samples are independent. -
The sample differences are from a normal population or the sample size in each sample is large enough to rely on the Central Limit Theorem.
-
We are told that the samples were sampled from distances the student lives from campus, indicating the samples are independent. -
The normal quantile plot in Figure 3 shows that the points do not fall well along the expected line, therefore the assumption is not normally distributed. Figure 3: Normal quantile plot of the sample of difference. Defining the variable distance – enrolment. Question 2 income level and school life expectancy using descriptive statistics. Is there evidence of a difference in average school life expectancy values among the four income levels? state the null and alternative hypotheses, observed test statistic, null distribution, p-value, decision and provide an appropriate conclusion in plain language. Let µ
L
,
µ
LM,
µ
UM, and µ
H be the population mean of school life expectancy in the 4 levels of income, low income, low-middle income, upper-middle income, and high income, respectively. To proceed with the analysis of variance, ANOVA output is produced by Jamovi Figure 4. ANOVA - School life expectancy
Sum of Squares df Mean Square F p Income Level
376
3 125.25
38.0 < .001
Residuals 185
56 3.30
Figure 4: AVOVA output for the School life expectancy among income level.
Your preview ends here
Eager to read complete document? Join bartleby learn and gain access to the full version
- Access to all documents
- Unlimited textbook solutions
- 24/7 expert homework help
The hypothesis: 𝐻𝐻
0
: 𝜇𝜇
𝐿𝐿
= 𝜇𝜇
𝐿𝐿𝐿𝐿
= 𝜇𝜇
𝑈𝑈𝐿𝐿
= 𝜇𝜇
𝐻𝐻
𝐻𝐻
𝐴𝐴
∶ 𝑎𝑎𝑡𝑡
𝑙𝑙𝑙𝑙𝑎𝑎𝑙𝑙𝑡𝑡
2 𝜇𝜇
′
𝒾𝒾
𝑎𝑎𝑎𝑎𝑙𝑙
𝑑𝑑𝑑𝑑𝑑𝑑𝑑𝑑𝑙𝑙𝑎𝑎𝑙𝑙𝑑𝑑𝑡𝑡
𝑡𝑡𝑡𝑡
𝑙𝑙𝑎𝑎𝑒𝑒ℎ
𝑡𝑡𝑡𝑡ℎ𝑙𝑙𝑎𝑎
The test statistic is F = 38.0 shown in Figure 4 as shown in the F column for income level
. Null distribution: If H
0
is true F ~ F
3, 56
. The degrees of freedom are shown in the df
column of the Income Level
and residuals rows of Figure 4 The p-value is given by: The p-value = P(F
3, 56
> 38.0) <0.001 ( see p
column of income level
in Figure 4) Conclusion: Because the p-value is small, reject H
0
. There is strong evidence that at least two income levels have significantly different average in school life expectancy. If appropriate, perform post-hoc tests to determine which income levels have significantly different average school life expectancies. If post-hoc tests are not appropriate, explain the purpose of a post-
hoc test and why it’s not appropriate in this example. Due to rejecting H
0
, it is appropriate to consider the post-hoc tests, as there is evidence stating that there at least 2 means that are different as the p-value was low. However a post-hoc test is needed to determine which means are different. Post Hoc Comparisons - Income Level
Comparison Income Level Income Level Mean Difference SE df t p
tukey
Low income - Lower-middle income -2.06 0.712 56.0 -2.89 0.027 - Upper-middle income -4.38 0.654 56.0 -6.69 < .001
- High income -6.92 0.688 56.0 -
10.05 < .001
Lower-middle income - Upper-middle income -2.32 0.654 56.0 -3.55 0.004 - High income -4.86 0.688 56.0 -7.06 < .001
Upper-middle income - High income -2.54 0.627 56.0 -4.05 < .001
Figure 5: Post-hoc output for the average school life expectancy across 4 types of income levels. The post-hoc test suggests that: -
The mean of the average school life expectancy for lower income was
not significantly different from the mean of the average life expectancy for lower-middle income. Therefore
we have no reason the dispute the claim that µ
L = µ
LM. This evidence is due to the p-value being small (p = 0.027) -
The mean of the average school life expectancy for lower income was significantly different from the mean of the average school life expectancy for upper-middle income. There is evidence that µ
L
≠ µ
UM
. This evidence due the p-value being small (p < 0.001). -
The mean of the average school life expectancy for lower income was significantly different
from the mean of the average school life expectancy for high income. There is evidence that µ
L
≠ µ
H
. This evidence is due to the p-value being small (p < 0.001) -
The mean of the average school life expectancy for Lower-middle income not significantly different from the mean of the average school life expectancy for upper-middle income. There is evidence that µ
LM
= µ
UM
. This evidence is due to the p-value being small (p = 0.004) -
The mean of the average school life expectancy for Lower-middle income significantly different
from the mean of the average school life expectancy for high income. There is evidence that µ
LM
≠
µ
H
. This evidence us due to the p-value being small. (p = < 0.001) -
The mean of the average school life expectancy for Upper-middle income significantly different
from the mean of the average school life expectancy for High income. There is evidence that µ
UM
≠
µ
H
. This evidence is due the p-value being small (p = < 0.001) What are the assumptions of the analysis performed in part (a)? State whether each assumption is reasonable with reference to appropriate Jamovi output. The ANOVA assumes: -
Observation are independently sampled from the target population -
Each populations are the same variance. -
Each population have the same distribution. Homogeneity of Variances Tests
Statistic df df2 p School life expectancy
Levene's 1.06 3 56 0.375
Bartlett's 1.32 3 0.725
Figure 6: Additional output (Levene’s Test) related to the ANOVA output. Assumption checks: -
We can assume that the observations are independent with each other due to the target population being the economic development level of the 4 categories of income in each country and how school life expectancy reflected off each other. -
The p -value in Figure 6 is larger (0.375) due to this there is no reason to reject the null hypotheses of equal variances. -
Shown in Figure 7, the normal quantile plot are along the expected line well and therefore the assumption of normality is reasonable, and the sample size is large enough to reply in the Central Limit Theorem.
Figure 7: Normal quantile plot of school life expectancy. Question 3 Generate an appropriate scatter plot with a fitted regression line. In Figure 8 shows a scatterplot of the Osmolality vs USG (urine specific gravity). The scatterplot indicted a linear increasing and moderate strong relationship between the variables. The strength can be measure by the correlation coefficient of 0.871, as shown in Figure 9 . Figure 8: Scatterplot of USG VS Osmolality
Your preview ends here
Eager to read complete document? Join bartleby learn and gain access to the full version
- Access to all documents
- Unlimited textbook solutions
- 24/7 expert homework help
Is there a statistically significant positive linear relationship between specific gravity and osmolality? Be sure to define any parameters you use, state the null and alternative hypotheses, observed test statistic, null distribution, p-value, decision and provide an appropriate conclusion in plain language. Let β
1
be the slope of the population regression line of USG on the osmolality. The hypothesis is: 𝐻𝐻
0
∶
𝛽𝛽
1
= 0
𝐻𝐻
𝐴𝐴
∶
𝛽𝛽
1
≠
0
The test statistic is 𝑡𝑡
=
𝑏𝑏
1
−𝛽𝛽
1
𝑆𝑆𝑆𝑆
(
𝑏𝑏
1
)
= 15.5
(shown in Osmolality
row of the t column of Figure 9) Null distribution: If H
0 is true, the observed test statistic t comes from the t-distribution with n – 2 degrees of freedom. As we have 78 urine specimens, the null distribution is t
76
. The p-value given by is: p- value = 2 𝑥𝑥
𝑃𝑃
(
𝑡𝑡
76
> 15.5
) = < 0.001 Conclusion: Due the p-value being small, we reject the null hypothesis and conclude that there is a significant linear relationship between the USG and osmolality. Model Fit Measures
Model R R² 1 0.871 0.759
Model Coefficients - USG
95% Confidence Interval Predictor Estimate SE Lower Upper t p Intercept 1.00 0.00113 0.999 1.00
883.7 < .001
Osmolality
2.66e-5 1.72e-6 2.32e-5 3.00e-5
15.5 < .001
Figure 9: Regress output from Jamovi for predicting the USG from the Osmolality. State the assumptions necessary for a regression analysis to be appropriate. State whether each of them is satisfied with a brief justification. The assumptions for line regression are: -
The data consists of independent observation from each of population X and Y – this would be reasonable as you the measurement are taken from 2 variable over 78 types of specimen. -
There is a linear relationship between the mean of X and Y. The plot of residuals vs fitted value in Figure 10 shows a trend where most points sit around the zero line. -
Residuals have a constant variance. In Figure 10 The residues tend to be centred about zero indicating no evidence of violation of the linearity assumption, however, there does appear to be a pattern in the variance where as we go left to right the amount of variance increases
then decreases and increases again resulting in non-constant variance and therefore representing a violation of assumption. -
Residuals are normal distributed, or the sample size is large enough to rely on the Central Limit Theorem. The point do not fall along the expected line well in the normal quantile plot in Figure 10, suggesting that the assumption is not reasonable. In any case, the sample size would be large enough to rely on the Central Limit Theorem, n = 78. Figure 10: Residual plot and a normal quantile plot of residuals for the regression of USG per Osmolality. Write down the equation for the estimated regression line and provide an interpretation of the slope coefficient. The equation for the estimate regression line is ŷ
= 2.66e-5 + 1.00x where ŷ
is the predicted USG and x is the osmolality. The intercept of b
0
= 2.66e-5 (2.66 x 10
-5
) indicates that when the value of Y when X = 0 The slope b
1
= 1.00 indicates that on average every increase for each unit increase in X Predict the osmolality for a USG value of 1.025. Ŷ
= (2.66 × 10
−5
) + 1.00 × 1.025
= (2.66 × 10
−5
) + 1.025
= 1.025
Write down the R2 value for this regression and give an interpretation. From Figure 9, R
2
= 0.759. we can interpret this as 75.9% of the variability of the USG can be explained by the linear relationship with the osmolality. Based on the R2 value do you think that using USG to predict osmolality is a sensible thing to do? Why or why not. the coefficient of determination measure the strength on how much of the variation in Y is explained by the regression. As stated in above question and in Figure 9, there is a 75.9% of variation in
osmolality. You could say that using USG to predict osmolality is a sensible thing as USG is a measure of the weight of solids in water and osmolality is a measure of the concentration of the urine, due being both highly positively correlated, however with larger and heavy molecules being present in the urine numbers can diverge. Due to this Osmolality to predict USG could be more sensible as it would be more of an accurate measurement.
Your preview ends here
Eager to read complete document? Join bartleby learn and gain access to the full version
- Access to all documents
- Unlimited textbook solutions
- 24/7 expert homework help
Related Documents
Recommended textbooks for you
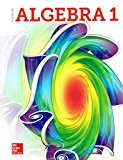
Glencoe Algebra 1, Student Edition, 9780079039897...
Algebra
ISBN:9780079039897
Author:Carter
Publisher:McGraw Hill
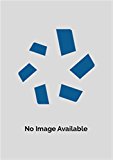
Big Ideas Math A Bridge To Success Algebra 1: Stu...
Algebra
ISBN:9781680331141
Author:HOUGHTON MIFFLIN HARCOURT
Publisher:Houghton Mifflin Harcourt
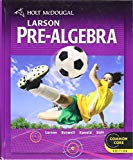
Holt Mcdougal Larson Pre-algebra: Student Edition...
Algebra
ISBN:9780547587776
Author:HOLT MCDOUGAL
Publisher:HOLT MCDOUGAL
Recommended textbooks for you
- Glencoe Algebra 1, Student Edition, 9780079039897...AlgebraISBN:9780079039897Author:CarterPublisher:McGraw HillBig Ideas Math A Bridge To Success Algebra 1: Stu...AlgebraISBN:9781680331141Author:HOUGHTON MIFFLIN HARCOURTPublisher:Houghton Mifflin HarcourtHolt Mcdougal Larson Pre-algebra: Student Edition...AlgebraISBN:9780547587776Author:HOLT MCDOUGALPublisher:HOLT MCDOUGAL
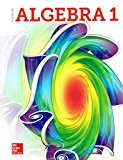
Glencoe Algebra 1, Student Edition, 9780079039897...
Algebra
ISBN:9780079039897
Author:Carter
Publisher:McGraw Hill
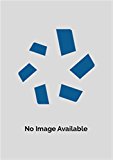
Big Ideas Math A Bridge To Success Algebra 1: Stu...
Algebra
ISBN:9781680331141
Author:HOUGHTON MIFFLIN HARCOURT
Publisher:Houghton Mifflin Harcourt
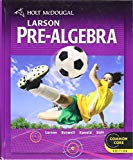
Holt Mcdougal Larson Pre-algebra: Student Edition...
Algebra
ISBN:9780547587776
Author:HOLT MCDOUGAL
Publisher:HOLT MCDOUGAL