chapter 5 - planning - 8th edition
docx
keyboard_arrow_up
School
Durham College *
*We aren’t endorsed by this school
Course
1200
Subject
Economics
Date
Feb 20, 2024
Type
docx
Pages
74
Uploaded by sprakhar693
Chapter 5: Determining HR Demand
Figure 5.1
Forecasting the demand for human capital requires an understanding of the firm's
strategic requirements. [1]
Learning Outcomes
L.O. 5.1
Understand what HR forecasting is, and its strategic importance to the firm.
L.O. 5.2
Understand when to apply quantitative methods such as trend/ratio analysis, time series models, correlation, regression, structural equation modelling, or a categorization-based or prediction-based machine learning model.
L.O. 5.3
Describe the various qualitative forecasting methods including scenario planning, Delphi technique, nominal group technique, and HR budgets and staffing tables, and the situations in which they are most effective.
L.O. 5.4
Understand how quantitative and qualitative models can be combined in tandem or using simulations to produce more informative forecasts.
Introduction
The Great Resignation
Over the long term, the demand for labour in Canada is expected to increase. However, by 2036 the aging Canadian population is expected to lead to a decrease in the labour force participation rate. In the 1980s, there were six working Canadians for every non-
working Canadian over the age of 65. By 2017, this ratio declined to four people in the labour force for every person not in the labour force above the age of 65, and by 2036 the ratio is expected to further fall to less than three workers for every non-worker over the age of 65. This decline may be offset to some extent by an increase in the number of
workers in the labour force born outside of Canada.
The COVID-19 pandemic introduced significant changes to the global labour landscape. Many of the changes that resulted from the pandemic have yet to be fully understood. For example, the labour force in the United States has seen a large increase in resignations from full-time workers since 2020, but the same phenomenon has not been observed in Canada. The majority of the resignations occurring in the U.S. labour force are in the hospitality and retail sectors, where the effects of the pandemic have been very strong. These industries have not yet returned to pre-pandemic levels, and industries such as technology are growing rapidly. While it is too soon to tell, it is possible that American workers are leaving jobs in hospitality and retail to retrain for industries with a more promising future.
In Canada, the employment gains in the labour force have been driven by increases in full-time work, and a decrease in part-time work. The downward pressure in labour force participation in Canada that is coming from the aging population will be offset to some extent by increased participation from visible minorities and immigrants to Canada. However, current projections suggest that by 2050 less than 60 percent of Canadians
will be of working age (between the ages of 15 and 64) (Heaven 2022), and the immigration rate may not be sufficient to fill the projected employment gap. Employers that are better able to attract and retain visible minorities, Indigenous peoples, neurodiverse workers, and older workers will be more successful in fulfilling their firms' HR planning requirements. Refer to Video 5.1 to learn more about the labour supply issues.
Video 5.1
The Great Resignation [2]
Sources: Martel (2019); U.S. Bureau of Labor Statistics (2022); Statistics Canada (2022);
Lundy (2021)
5.1 Forecasting Demand
HR demand refers to the firm’s future need for human capital, and to the types of jobs and number of positions that must be filled for the firm to implement its strategy. The demand for human capital is determined by the strategic and operational requirements of
the firm or business unit. This means that understanding the demand for talent begins with the firm’s strategy, and flows from the value-generating activities of the firm. A systems perspective (read Chapter 9
,
Change Management, for more information about a systems view) suggests that in order to determine the demand for any job, it is first necessary to understand how that job fits into the needs of the organization, and how those job requirements interact with other jobs within the firm. Therefore, the demand
Your preview ends here
Eager to read complete document? Join bartleby learn and gain access to the full version
- Access to all documents
- Unlimited textbook solutions
- 24/7 expert homework help
question ultimately begins with a clear understanding of how the firm brings value to the customer, and an estimate of the extent to which the market will want to consume the firm’s products or services.
For example, as auto companies like Ford, GM, BMW, and Mercedes bring electric vehicles to the market, they might ask themselves what proportion of their total auto sales will be expected to come from electric vehicles over the next 10 years. The answer
to such a question will certainly impact the technologies in which these companies will be
investing, the kinds of materials they will be using, the methods of manufacturing they will employ, and the employees required to design and manufacture these vehicles. Given the high degree of uncertainty around the future availability of charging stations or other infrastructure necessary to make electric vehicles more convenient to use, and of the kinds of product attributes that will make consumers want to buy electric vehicles (Figure 5.2), the answer to this demand question is potentially complicated and error prone.
Figure 5.2
Uncertainty around the technology and a new market segment can make it
challenging for companies like Hyundai to forecast the demand for human capital to produce
new electric vehicles. [3]
Consider, on the other hand, a company in Canada’s garment industry, such as Canada Goose. As manufacturer of the well-known Canada Goose line of winter coats and parkas, the demand for labour at Canada Goose is probably tied fairly closely to sales of its products. While some styles will always be more fashionable than others, forecasting the demand for labour at Canada Goose over the next 10 years is probably more straightforward than doing so at an electric car manufacturing facility. Because of the variety in the complexity and levels of uncertainty in forecasting the demand for labour, multiple forecasting methods exist. These methods can be divided into two main categories: quantitative methods and qualitative methods. The two main factors that determine whether a quantitative model or qualitative model is a better choice are the degree of uncertainty involved in the demand forecast, and the volume and complexity of
the data that are available to assist in creating the demand forecast.
5.1.1 The Role of Job Analysis in Forecasting the Demand for Labour
There are several forces in the workplace today that are changing the way work is organized. The pace of change in the workplace coupled with the organizational need for
agility, and the increased specialization of certain types of work are all putting pressure on the concept of the job. The job is being broken apart into more loosely organized groups of tasks, and many tasks are being taken out of the job altogether and performed by specialized workers or outsourced to the gig economy. This is referred to by some scholars as "fluid work," and it is evidenced in the workplace by the increased use of gig workers, contractors and other forms of outsourcing of tasks, consultants for specialized tasks, part-time workers, and job-sharing practices (Boudreau 2020). However, even though the job may be dissolving in some respects, the practice of job analysis still allows the organization to codify the knowledge, skills, abilities, and other attributes (KSAOs) necessary for the organization to deliver its value proposition. A job analysis provides the organization with a prioritized list of duties, tasks, and the KSAOs required to perform those tasks. This is the first step in assessing the demand for labour, as it gives the organization a valid and reliable understanding of what work needs to be done.
From a planning perspective, the next critical question to answer is how many people will
be required to perform the necessary tasks.
5.1.2 Certainty in Forecasting
Some quantitative models are based on what is known about existing relationships between a level of consumer demand or production, where a forecast already exists, and
human capital demand. For example, a large furniture manufacturing company that produces a particular type of reclining chair probably knows its market quite well. It understands who its competitors are, how large a share of the market for recliners it
Your preview ends here
Eager to read complete document? Join bartleby learn and gain access to the full version
- Access to all documents
- Unlimited textbook solutions
- 24/7 expert homework help
holds, and consequently how many recliners it expects to sell over the next six months. The company would easily be able to measure the number of employees and hours required to build each chair, and so calculating the demand for employees over the next six-month period is simply based on a known forecast of customer demand. In this case, the demand for recliner chairs is fairly stable, because the market for recliner chairs is fairly stable. There exists a comparatively high degree of certainty in the market, and so a simple model based on an existing forecast provides an effective model for assessing the demand for labour.
5.1.3 Volume and Complexity of Available Data
While no forecast is ever perfectly accurate, it may be possible to improve the accuracy of forecasting by including more factors that contribute to changes in demand. For example, customer demand for some products may be highly seasonal, and so production and consequently the demand for labour will change seasonally. Rather than basing demand forecasts on total sales per year, labour demand forecasts can be produced that follow product sales more closely when the seasonality of sales is included. In this case, the demand for labour is based on product demand, but also includes data that relate to when that demand ebbs and peaks.
Organizations interact with their environments. Because the environment (customers, competitors, the economy, etc.) is always changing, the complete set of factors that predict the demand for a particular job, set of jobs, or set of tasks may never be known. However, by observing how the demand for labour changes, theorizing what might influence changes in demand, and collecting data based on theory and observations, it may be possible to increase the consistency of forecasts. However, this increase in consistency comes at the price of collecting data over longer periods of time and collecting data from a wider variety of sources.
In general, quantitative models are better when forecasting demand in stable markets when there is a high degree of certainty in the relationship between the demand for labour and the indicators of that demand. As the volume and complexity of data that are available increase, quantitative models can deal with higher levels of uncertainty, but when uncertainty becomes very high, as in the case for something like the market for electric vehicles over the next three years, qualitative models become preferable. HR Planning Notebook 5.4.1: Differences between Qualitative and Quantitative Forecasting Data, found toward the end of the chapter, summarizes the types of data used for quantitative and qualitative models, and the resulting outcomes from each model type.
Steeping some tea...
Steeping some tea...
5.2 Quantitative Methods
Table 5.1 summarizes the quantitative forecasting methods to be discussed.
Table 5.1 Quantitative Forecasting Methods
QUANTITATIVE
METHODS
DATA
Trend/Ratio Analysis
This uses historical changes to predict future human capital needs.
Time Series Models
These models use past data to predict future demand.
Time series models are especially useful for capturing seasonality in data (such as a December rush on sales and a summer slump).
Correlation
This method describes the strength of a relationship between two variables (for
example, job satisfaction and job commitment, or sales and number of employees).
Regression Analysis
This shows a linear relationship or trend between one or more predictor variables and an outcome variable.
A regression analysis allows the user to predict the outcome based on known values of the predictor variables.
Structural Equation Modelling (SEM)
This modelling shows the relationships between multiple predictor and outcome variables.
SEM is useful for examining more complex models with more variables than regression
models.
Machine Learning and Artificial Intelligence (AI)
Categorization-
Based
Prediction-Based
Neural Networks
Random Forests
This finds patterns in large amounts of data
(for example, Amazon customer product reviews).
Machine learning can make use of many more variables than regression or SEM, and can be used to predict a particular outcome, or identify categories among large amounts of data.
5.2.1 Trend/Ratio Analysis
Trend analysis
is a general term for any type of quantitative approach that attempts to forecast future human capital needs by extrapolating from historical changes in one or more organizational indices. A basic form of trend analysis could be plotting previous levels of employment to determine future needs. This method uses prior employment numbers for a job as the organizational index, and assumes that the future will be quite similar to the past because the inferences that are being made about future employee
Your preview ends here
Eager to read complete document? Join bartleby learn and gain access to the full version
- Access to all documents
- Unlimited textbook solutions
- 24/7 expert homework help
requirements are based on observations of actual past needs. Trend analysis can be used to spot how changes in other attributes such as sales, inventory levels, revenues, etc., might correspond with changes in employee requirements. Figure 5.3 demonstrates
how prior knowledge of employee numbers can be used in a trend analysis to predict future requirements.
Figure 5.3
Trend analysis is useful to forecast labour requirements.
Ratio analysis
involves examining the relationship between an operational index and the demand for labour (as reflected by the number of employees in the workforce) and is a relatively straightforward quantitative demand forecasting technique commonly used by many organizations (Elbo 2000, 1–3; Cascio 1991; Ward 1996, 54–55). For example, an organization may choose to look at the ratio of sales to employees. Although sales level is probably the most common index used by organizations, other operational indices include (1) the number of units produced, (2) the number of clients serviced, and (3) the production (i.e., direct labour) hours. Similarly, although the relationship between the operational index and workforce size (number of employees) can be calculated for the entire organization, as well as for the department or operational subunit, some organizations use ratio analysis to ascertain demand requirements for (1) direct labour and (2) indirect labour (e.g., HR staff). Table 5.2 in HR Planning Notebook 5.2.1 shows an example of ratio analysis. There are five steps to conducting an effective ratio analysis.
1.
Step 1. Select the Appropriate Business/Operational Index.
The HR
forecaster must select a readily available operational index, such as sales level, that is (1) known to have a direct influence on the organizational demand for labour and (2) subjected to future forecasting
as a result of the normal business planning process.
2.
Step 2. Track the Operational Index Over Time.
Once the index has been selected, it is necessary to go back in time for at least the four or five most recent years, but preferably for a decade or more, to record the quantitative or numerical levels of the index over time.
3.
Step 3. Track the Workforce Size Over Time.
Record the historical figures of the total number of employees, or, alternatively, the amount of
direct and indirect labour for exactly the same period used for the operational index in Step 2.
4.
Step 4. Calculate the Average Ratio of the Operational Index to the Workforce Size.
Obtain the employee requirement ratio by dividing the level of sales for each year of historical data by the number of employees required to produce that year’s level of sales. This ratio is
calculated for each year over the period of analysis so that an average ratio describing the relationship between the two variables over time can be determined.
5.
Step 5. Calculate the Forecasted Demand for Labour.
Divide the annual forecast for the operational index by the average employee requirement ratio for each future year to arrive at forecasted annual demand for labour. For example, obtain future sales forecast figures for the next five years. For each of the years, divide the level of sales by the average employee requirement ratio to obtain the forecasted numerical demand for labour for each future year (Figure 5.4).
Figure 5.4
By estimating output,
managers can predict labour inputs that are needed. [4]
HR Planning Notebook 5.2.1: Ratio Analysis: Puslinch Pottery
Table 5.2 Puslinch Pottery Ratio Analysis
Year
Operational Index
Sales
($ Thousands)
Number of Employees
Employee Requirement Ratio
(Sales per Employee)
201
7
2,800
155
18.06
201
8
3,050
171
17.83
201
3,195
166
19.25
9
202
0
3,300
1
177
18.64
202
1
3,500
188
2
18.64
3
202
2
3,600
193
4
18.64
202
3
3,850
207
5
18.64
1
The initial forecast is developed based on 2020 actual data. We are forecasting labour demand for 2021, 2022, and 2023, and therefore sales figures for those years are future estimates.
2
Employee numbers are historical, except for the figures for 2021, 2022, and 2023, which are our future HR demand forecasts. Now that the employee requirement ratio has
been established from 2020, we can use it and the expected sales from 2021 to establish the future required number of employees. In 2021, the required number of employees will be 3,500/18.64 = 188 employees (rounded to the nearest whole number).
3
The index used to calculate future demand (number of employees) can be the most recent figure, or an average of the up-to-date period (e.g., the past four years, for which the average is 18.44). In this trend analysis, the most recent ratio (18.64) for the year 2020 was used for forecasting.
4
3,600/18.64 = 193 (rounded to the nearest whole number)
5
3,850/18.64 = 207 (rounded to the nearest whole number)
Steeping some tea...
Your preview ends here
Eager to read complete document? Join bartleby learn and gain access to the full version
- Access to all documents
- Unlimited textbook solutions
- 24/7 expert homework help
Steeping some tea...
Steeping some tea...
Steeping some tea...
5.2.2 Time Series Models
Times series models use past data to predict future demand. They can range from very simple to highly complex. For example, a simple time series model might use the value from the last season as the estimate. If trying to forecast the number of employee hours required to work in a retail store during December, the HR planner might use the previous December’s total sales, or a ratio of sales to total hours worked as an estimate for this year. The ratio analysis in HR Planning Notebook 5.2.2 is a form of time series design that uses the data from the current year (period) to predict the next year’s demand. Other forms of time series designs use more information from previous years; for example, a simple moving average may use the average values for actual demand data over the past two or three periods as the estimate for future demand.
HR Planning Notebook 5.2.2 shows an adaptation of the trend analysis in Notebook 5.2.1 that uses a simple moving average. Another form of moving average is a weighted moving average, in which all periods of actual demand data are used to estimate future demand, but greater weight is given to more recent demand data. A weighted moving average places more importance on recent demand data. To capture seasonality or trends in demand, exponential smoothing is used. Exponential smoothing also uses all past demand data, but places much greater weight on the most recent demand data (even greater weighting than a weighted moving average).
HR Planning Notebook 5.2.2: Time Series Analysis: Puslinch Pottery
Table 5.3 Time Series Analysis
Year
Operational Index Sales
Actual Numbers of Employees Required
Actual Employee Requirement Ratio (Three-year Moving Average)
Forecast Number of Employees Required
201
7
$2, 880
151
18.06
201
8
$3,050
171
17.83
201
9
$3,195
166
19.25
202
0
$3,300
177
18.64
202
1
$3,500
185
18.82 (18.57)
6
188
202
2
$3,600
200
18.00 (18.90)
7
190
202
3
$3,850
210
18.30 (18.49)
8
208
6
three-year moving average = (17.83 + 19.25 + 18.64)/3
7
three-year moving average = (19.25 + 18.64 + 18.82)/3
8
three-year moving average = (18.64 + 18.82 + 18)/3
Although trend/ratio analysis is widespread due to its ease of use, remember that the analysis usually incorporates only the relationship between a single business variable
and demand for labour (workforce size). By design, any single-variable relationship provides a relatively simplistic forecast of demand. For more comprehensive analyses that reflect a variety of factors affecting business operations, such as customer preferences, interest rates, level of unemployment, consumer disposable income, and so
on, the quantitative techniques normally employed are multivariate regression or other similar modelling/programming models (Al-Subhi Al-Harbi 2000, 47–55; Meehan and Ahmed 1990, 297–307). These models typically depend on large amounts of reliable data.
Steeping some tea...
Steeping some tea...
Question 5.9
Review
If a bike manufacturer is using last year’s sales value to calculate HR demand, what method is it using?
Select an answer and submit. For keyboard navigation, use the up/down arrow keys to select an answer.
a
a simple regression analysis model
b
Your preview ends here
Eager to read complete document? Join bartleby learn and gain access to the full version
- Access to all documents
- Unlimited textbook solutions
- 24/7 expert homework help
a simple annual budget model
c
a simple time series model
d
a simple Markov analysis model
Show Submitted Answer
You don't have a recorded answer from classroom or homework
Show Correct Answer
Check My Answer
Please enter an answer to submit.
5.2.3 Correlation
Correlation
is used to describe the relationship between two variables. Correlation can be
used to describe the strength of the relationship between SAT scores and undergraduate
GPA, or between conscientiousness and job performance, or between job satisfaction and turnover, or between the number of employees working on the sales floor of a department store and store sales. All these examples might range from a strong positive relationship, where changes in one variable such as SAT scores are associated with similar changes in the other variable, which in this case is undergraduate GPA, to a strong negative relationship, where an increase in one variable associates with a decrease in the other variable, such as how higher job satisfaction associates with lower turnover. If there is no relationship between two variables (for example, if changes in job satisfaction are not expected to have any association with changes in cognitive ability) then the correlation is zero. Thus, correlation describes the strength of the association between two variables, and it can range from 1, which represents a perfect positive relationship, to negative 1, which represents a perfect negative relationship.
Correlation can be viewed in data by looking at a scatterplot. A scatterplot shows one variable on the x-axis (the horizontal axis) and the other variable on the y-axis (the vertical axis). Imagine that you have completed a survey that measures your level of job satisfaction and your level of organizational commitment. You and every other participant
in the survey will have an observation for the measure of job satisfaction and an observation for the measure of commitment. Figure 5.5 offers a depiction of correlation.
Each dot in the scatterplot represents a pair of values for each subject. For example, if job satisfaction is placed on the x-axis and organizational commitment is on the y-axis, each participant would represent a dot where the position of the dot on the x-
axis represents that participant's score for job satisfaction, and the position of the dot along the y-axis represents that same individual's score for organizational commitment. Together, the dots demonstrate whether or not job satisfaction and commitment show a correlation in this sample of participants.
Figure 5.5
Scatterplots are useful for visually spotting trends in data, and can provide a good
sense of whether a model (such as a regression line) fits the data. (Magnusson 2022) [5]Long
Text Description
Shared Variance
Correlation provides information around the direction and strength of the association between two variables. The concept of
shared variance
allows us to understand how much
of one variable can be predicted by the other variable. Shared variance is simply the correlation coefficient squared; and since a correlation coefficient is a number between -
1 and 1, the shared variance, or R
2
, is a smaller number than the correlation coefficient (unless the correlation coefficient is exactly -1 or 1). The interpretation of the shared variance is the amount of variance in one variable that can be predicted by the other variable. If the correlation coefficient between job satisfaction and organizational commitment is 0.3 (that is, r = 0.30), then the shared variance is R
2
= (0.3)
2
= 0.09, and the interpretation is that 9 percent of the variability in organizational commitment can be predicted by job satisfaction. This also means that 90 percent of the variability in
organizational commitment may be predicted by other things and not by job satisfaction. The bubbles in Figure 5.5 above show the amount of shared variance (that is, R
2
) between two variables based on their correlation.
5.2.4 Regression Analysis
Regression analysis
extends the idea of correlation in several important ways. First, while correlation describes the strength of a relationship in values that range from -1 to 1, regression describes the strength of a relationship in the same values as the actual variables, which permits a more straightforward interpretation. Second, regression can integrate the combined effects of multiple variables on another variable. In other words, if
a practitioner was interested in predicting the number of employees required based on sales amounts and production amounts together, regression can provide the amount of variance in the required number of employees that is predicted by these two variables (sales and production) combined.
Regression analysis can be thought of loosely as an extension of trend analysis, except that rather than using a single operational index (which in regression would be called the independent or predictor variable), regression uses more data than the ratio of the operational index to employees, and regression can use multiple predictor variables to forecast the required number of employees. The purpose of regression is to estimate a trend or relationship between one or more predictor variables and an outcome variable (refer to HR Planning Today 5.2.1 for an example of regression analysis). If we are able to predict enough variability in the outcome (or criterion) variable with our trend line, then
the trend line may be used to extrapolate beyond the data at hand to create a forecast.
Remember that the predictor variable is the information that we are using to try to predict
or forecast the number of required employees; in this case, we are trying to predict the number of employees who will be required as sales revenues increase. Where trend analysis borrows information from a single or several data points on the scatterplot to derive an employee requirement ratio, regression borrows information from all the data points to predict the criterion. Regression essentially draws a line that best describes the trend or relationship between the predictor and the criterion as in Figure 5.6. It does this by drawing the line that reduces the sum of the distances between each point on the scatterplot and the line. Positive and negative distances from the line can cancel one another out. Regression therefore squares the distances from the line so that all distances are positive. The slope of that line represents the amount of change in the outcome variable resulting from a one-unit change in the predictor variable. By travelling up or down that trend line, we can predict the number of employees required for a given level of sales.
Your preview ends here
Eager to read complete document? Join bartleby learn and gain access to the full version
- Access to all documents
- Unlimited textbook solutions
- 24/7 expert homework help
Figure 5.6
Trend LineLong Text Description
HR Planning Today 5.2.1: Regression in Action
To get a sense of how regression may be used, we will take a very simple fictional case of a motorcycle manufacturer. Lacrosse Motorcycles represents the rejuvenation of an iconic brand of motorcycle from the 1960s. The motorcycles the company produces are large, rugged bikes that are technologically simple, and inexpensive to buy and maintain.
Lacrosse has been growing rapidly since first opening its doors almost five years ago, and now has sales and production data from approximately 20 quarterly periods. Using prior information about production, sales, and the numbers of full-time equivalent employees working during each of those quarters, we would like to forecast the company’s demand for employees as it grows (refer to Table 5.4).
Table 5.4
Regression Analysis Data
Sale
s
Productio
n
Employees
70
20
50
74
22
55
80
25
62
82
27
70
83
32
70
102
40
85
88
45
75
95
33
75
55
35
75
97.5
40
80
100
50
85
105
52
92
108
55
95
120
60
105
135
70
115
150
75
125
165
80
135
175
90
150
We can examine the extent to which production predicts the number of required employees by performing a regression analysis using production as the only predictor of employees. The resulting plot of our data including the regression trend line is shown in Figure 5.7.
Figure 5.7
A Regression Trend Line Using Production As The Only Predictor Of Employees
The equation for the trend line, which we get from our statistics package, looks like this:
Employees = 27.3 +
(1.3) ×
production
Our statistics package also reports the coefficient of determination as 0.97, which can be
interpreted as meaning that 97 percent of the variability in the number of employees can be explained by production. This is clearly very high – and is much higher than you might
see in most real-life situations. The equation can be interpreted as meaning that for every one-unit increase in production, we will need 1.3 more employees. The 27.3 number represents the intercept point on the graph where the predictor (in our case, production) is zero. While the number of workers required when production is zero is probably not relevant to the forecaster, this point shows where the trend line begins for these data. So, if we want to extrapolate how many employees we will need when production ramps up to 200 motorcycles, we simply insert it into our formula:
Employees = 27.3 +
(1.3) ×
200 motorcycles
This means that in order to produce 200 motorcycles, we will need 27.3 + 260 = 287 employees. The trend line shows production values and the required number of employees for values that are included in the data. However, we have used the regression equation to extrapolate beyond the data to forecast a future anticipated level of production. In this way, regression is useful for making predictions beyond the data at hand, provided that it is safe to assume that the relationship between the predictor and the outcome is more or less linear.
Not forgetting that we also have sales data, we can create another model that includes sales in our regression. The results of this statistical analysis are as follows:
Employees = 19.82 +
(0.97) ×
p
roduction + (.22) × sales
Our statistics package reports the coefficient of determination for this model is .975, which means that 97.5 percent of the variability in the number of employees required can
be accounted for by both production and sales. The model is interpreted as saying that when holding production constant, for every one-unit increase in sales (sales units are in thousands of dollars, so one unit of sales equals $1,000), we require 0.22 more employees. When holding sales constant, every one-unit increase in production requires 0.97 more employees. This suggests that our model is more sensitive to changes in production
than to changes in sales
. How does this compare to our earlier (and simpler) model? This model predicts .5 percent more variability in employees than our model that uses just production. Is that worth making the model more complicated? Probably not, as
we are just as well served by the model that uses only production as a predictor of employees. The best regression model is the simplest model that predicts the most variability in our criterion variable. Thus, the best model given these data is our first model, which uses production as a single predictor of employees.
Now You Try It!
Let's replicate the regression analysis in HR Planning Today 5.2.1 on your computer. Download
Jamovi
. It's free and intuitive to use, and there is a cloud-based version if you are using a mobile device and cannot access a computer. Once installed and opened on
your computer, click on the data tab at the top. Copy and paste or manually enter the data provided in Table 5.4, Regression Analysis Data, into the data section. Where Jamovi lists A, B, and C as column names at the top of the data, click on these letters, and change the names (for A, enter "Sales"; for B, enter "Production"; and for C, enter "Employees").
Now that the data has been entered and named, let's create a scatterplot with a trend line. Click on the "Analyses" tab at the top of the page, and then the "Exploration" tab. Within the "Exploration" submenu, click "Scatterplot" and click "Production" over to the x-
axis and "Employees" to the y-axis. Where it says "Regression line," click "Linear" to get a straight trend line through the data. The resulting plot will show the linear trend between production and the level of employees. To quantify this relationship, click the "Regression" box at the top of the page and select "Linear Regression." Click
Your preview ends here
Eager to read complete document? Join bartleby learn and gain access to the full version
- Access to all documents
- Unlimited textbook solutions
- 24/7 expert homework help
"Employees" as the dependent variable, and "Production" as the covariate. Jamovi will provide the output for the regression, which should be very close to the results shown in HR Planning Today 5.2.1. For more on how to use Jamovi and perform and interpret statistical analyses, read
Learning Statics by Jamovi: A Tutorial for Psychology Students and Other Beginners
(Navarro and Foxcroft 2022).
Video 5.2 presents an online tutorial to take you further into learning how to use Jamovi.
Video 5.2
Jamovi Tutorial [6]
When a regression includes two independent variables (for example, sales as well as production volume), another axis is added as in Figure 5.8, and the regression line becomes a three-dimensional regression plane. A regression can include as many variables as make sense for the model in order to explain more of the variance in the outcome variable, although the model becomes more complex. The choice of predictor (also known as independent) variables is based on a logical or theoretical basis for assuming that a change in each predictor should for one reason or another lead to a change in the criterion (or outcome, which is the demand forecast). When trying to predict demand, it is useful to try to include enough independent variables to predict as much variability in the criterion as possible. Predictors that are highly correlated with each other should be avoided, and if the model contains more than six–eight predictor variables, it might be better to use a different kind of analysis (like structural equation modelling or a big data technique).
Your preview ends here
Eager to read complete document? Join bartleby learn and gain access to the full version
- Access to all documents
- Unlimited textbook solutions
- 24/7 expert homework help
Your preview ends here
Eager to read complete document? Join bartleby learn and gain access to the full version
- Access to all documents
- Unlimited textbook solutions
- 24/7 expert homework help
Figure 5.8
Three-dimensional Regression PlaneLong Text Description
When developing a model, Meehan and Ahmed (1990, 297–307) suggest grouping possible predictor variables in three categories:
1.
key variables, which are almost certain to play a role in predicting the employee requirement;
2.
promising variables, which appear important and you suspect will probably relate to predicting the employee requirement; and
3.
possible variables, which may not be necessary for the model.
Once the variables have been selected, past data for the variables can be entered into a regression model and run through a statistics package (such as SPSS, JAMOVI, R, or even Excel). The results of the statistics software will indicate how much variability in the criterion is predicted by the entire model; this is called the coefficient of determination. Again, the more variability in the criterion variable that is predicted by the model, the better. The model can be refined until the maximum amount of variability is predicted.
At this point, the HR planner can use the model to forecast future needs for service technicians based on known values of the predictor variables, and use the model to create “what if”’ scenarios. The relative strength of each of the predictor variables is assessed using regression, which provides an understanding of the sensitivity of the criterion variable to changes in each of the predictors. This flexibility and ease of use as a modelling tool once the model has been developed helps to make regression a powerful and highly useful forecasting tool. Given the explosion in the types and amounts of data gathered by organizations today, regression models can benefit from this proliferation of data and help to make sense of an otherwise confusing mass of information.
Limitations of Regression
While regression models can be highly useful forecasting tools, like any powerful tool, statistical regression has its limitations.
Unsuitable for nonlinear relationships.
A primary limitation of regression models is when relationships are nonlinear. An example of a
linear relationship between two variables might be the relationship between gas consumption and the number of kilometres driven per year. The more kilometres you drive, the more gas you will use. A nonlinear relationship might be the relationship between the sales at a grocery store and the number of required employees. While these
Your preview ends here
Eager to read complete document? Join bartleby learn and gain access to the full version
- Access to all documents
- Unlimited textbook solutions
- 24/7 expert homework help
variables should correlate, and while the relationship might be linear over a particular set of values, a grocery store requires a full complement of employees even when there are only a few shoppers, and as the number of shoppers and the size of their grocery carts increase, the numbers of additional employees required might be expected to increase at a decreasing rate. Thus, we might expect to see a relationship such as the one depicted in Figure 5.9, which is a fictional example showing that as sales increase, the number of required employees at first increases linearly, but that the rate of increase then changes as economies of scale require proportionately fewer employees. Linear regression produces a prediction (or trend) line that is a straight line. Thus, when the relationship between a predictor and the criterion is not linear, it is not a good choice as a predictor. Because a stable, linear relationship cannot be assumed in emerging markets or in environments characterized by dramatic innovations, regression may not be an ideal forecasting method for these highly unstable markets.
Large amounts of historical data are required.
Regression models require sufficient amounts of historical data on which to develop predictions. For newer or smaller firms, it may take some time to develop enough data to enable regression to be useful. A general rule of thumb to begin is that a minimum of 20 observations are required in order to detect a strong relationship; however, as the number of predictor variables increases in the model and as the expected effect size of the predictors on the criterion decreases, the number of required
observations becomes larger.
Your preview ends here
Eager to read complete document? Join bartleby learn and gain access to the full version
- Access to all documents
- Unlimited textbook solutions
- 24/7 expert homework help
Figure 5.9
When relationships are nonlinear, regression becomes an unreliable and potentially
misleading tool for prediction.Long Text Description
Regression models help HR planners to make use of large amounts of organizational data. These models can be easily adapted to reflect organizational changes or new assumptions, and are a good choice for short-term, medium-term, and long-term forecasts. However, quantitative models based on historical data may not be the best indicator of future needs if the business or environment is in a period of significant transition.
Steeping some tea...
Your preview ends here
Eager to read complete document? Join bartleby learn and gain access to the full version
- Access to all documents
- Unlimited textbook solutions
- 24/7 expert homework help
Steeping some tea...
Steeping some tea...
Steeping some tea...
Steeping some tea...
Your preview ends here
Eager to read complete document? Join bartleby learn and gain access to the full version
- Access to all documents
- Unlimited textbook solutions
- 24/7 expert homework help
Steeping some tea...
5.2.5 Structural Equation Modelling
Structural equation modelling (SEM)
can be thought of as a process similar to regression, except that where regression deals with a single outcome variable at a time, there can be many outcome variables in a single SEM model. This permits the use of more variables and the development of more complex models using SEM. The primary drawback to SEM versus regression is that SEM typically requires more data observations than regression.
Forecasters can develop a model of labour demand based on their own theory as to what factors may be driving demand. Provided that measures exist for each of the variables in their proposed theoretical model, and provided that enough data has been collected on each variable, forecasters can use SEM to statistically test the effect of their
proposed model on demand.
Figure 5.10 shows a fictitious example of a basic structural equation model of employee demand for the job of a manufacturing worker in a company
that produces recliners.
Your preview ends here
Eager to read complete document? Join bartleby learn and gain access to the full version
- Access to all documents
- Unlimited textbook solutions
- 24/7 expert homework help
Figure 5.10
Structural Equation ModelLong Text Description
Question 5.16
Review
Structural Equation Modelling (SEM) permits the use of many outcome variables.
Select an answer and submit. For keyboard navigation, use the up/down arrow keys to select an answer.
Your preview ends here
Eager to read complete document? Join bartleby learn and gain access to the full version
- Access to all documents
- Unlimited textbook solutions
- 24/7 expert homework help
a
True
b
False
Show Submitted Answer
You don't have a recorded answer from classroom or homework
Show Correct Answer
Check My Answer
Please enter an answer to submit.
5.2.6 Machine Learning and Artificial Intelligence (AI)
One of the largest potential benefits of artificial intelligence and machine learning tools is the ability to find patterns in large amounts of data that would otherwise not be detectable. For example, machine learning tools can make use of large amounts of text, such as customer feedback comments on a website like Amazon.com to discover the product features that are most valued about a product, to diagnose product failures, or to
learn about features that customers would like to have in future iterations of the product. Machine learning can also be used to track inventory and delivery location data from GPS, customer transaction data, customer movement within retail stores, or employee digital footprint data. Organizations are now able to collect enormous amounts of information about customers, processes, and employees, and machine learning can help
to make connections within and between these areas. As shown in Figure 5.11, the two primary forms of machine learning tools are categorization methods and prediction methods. These tools essentially either categorize information based on patterns of similarity, or predict outcomes from within the data.
Your preview ends here
Eager to read complete document? Join bartleby learn and gain access to the full version
- Access to all documents
- Unlimited textbook solutions
- 24/7 expert homework help
Figure 5.11
Categorization methods and prediction methods are the two main forms of machine
learning tools.Long Text Description
Categorization-Based Machine Learning Methods
Your preview ends here
Eager to read complete document? Join bartleby learn and gain access to the full version
- Access to all documents
- Unlimited textbook solutions
- 24/7 expert homework help
Your preview ends here
Eager to read complete document? Join bartleby learn and gain access to the full version
- Access to all documents
- Unlimited textbook solutions
- 24/7 expert homework help
Figure 5.12
A machine learning analysis of the length and width of petals on a large number of
Iris flowers could lead to the identification of different categories of Iris species that are not
otherwise easily detected. [7]
These methods sort data into categories based on similarity. They often do not depend on a particular outcome that is to be predicted from the data. Rather, the analyses attempt to find patterns of similarity among the variables in the data, and are therefore called unsupervised methods. For instance, a botanist might record the lengths and widths of the petals for a large number of Iris flowers, depicted in Figure 5.12. The analysis will seek to find patterns of similarity based on petal length and width, and will identify whether there are multiple species of Iris flowers in the data.
Categorization-based machine learning methods may be useful to identify patterns among employee data as well. For example, a retail coffee shop could use data on its baristas' choices for what time of day they prefer to work shifts, how long a shift they like to work, how many years they have worked for the company, and the total number of hours worked per week to analyze whether or not there are different employee segments
within this particular job. Figure 5.13 presents an example of how length of shift and organizational tenure might be used to sort and identify different employee segments among baristas. Such information may be used to attract job seekers from each of these segments and plan work schedules using knowledge about the preferences from each of
these segments. Chapter 6, Ascertaining HR Supply, includes more information about how employee segmentation may be used to aid in the supply of human capital.
Figure 5.13
The length of a shift and organizational tenure can be used to segment casual, part-time, and full-time employees.
Prediction-Based Machine Learning Methods
The section on regression analysis has already introduced you to prediction-based methods, where a set of variables (which we can also call features) are chosen as predictors of the outcome variable. Many prediction models are called supervised models because the analysis is based on the prediction of a particular outcome. Prediction-based learning methods learn using existing data. If the outcome is known, the algorithm uses the existing data to predict the outcome. This is the learning portion of
the model, where the algorithm
learns
how to correctly predict the outcome. A portion of the data is withheld from this stage so that it can be used later to validate the model. At the validation stage, the model is used on these new data to predict the outcome, and the accuracy of the prediction model can be tested by examining the proportion of correct predictions made by the model.
Some of the features in a prediction model may be strong predictors of the outcome variable, and other features may not. However, where regression is limited to the use of relatively few features (that is, usually fewer than 10 variables) to predict the outcome, supervised machine learning methods can use many features. This is particularly useful with the variety and volume of data that organizations can now utilize, as supervised
Your preview ends here
Eager to read complete document? Join bartleby learn and gain access to the full version
- Access to all documents
- Unlimited textbook solutions
- 24/7 expert homework help
machine learning methods can make use of hundreds of features to predict the outcome variable. There are many different types of prediction models, some of which have interesting names.
Neural Networks
Neural networks
are named for their resemblance to the structure of the neural pathways in our brains. Neural networks are made up of many nodes, where a node is a point at which information arrives and is acted upon before being passed on as another piece of information for another node. For example, when prioritizing work tasks, information relevant to the decision such as the complexity of the tasks, the estimated amount of time a task might take, the extent of involvement from other people or resources, and other information might come from one or more nodes to a decision node around when to schedule one particular task over another. The blend and relative weight of variables to consider to make the best decision for how to use one's time comes with familiarity with making such a decision, and in time a person learns how to make this decision most
effectively. The algorithm for a neural network works in much the same way; it builds a neural network of nodes based on the information that it has been given in order to produce the desired outcome. The network is then tested on new data to discover how well the network predicts the outcome on the new data. As more data is received, the algorithm refines its network to improve prediction. Figure 5.14 depicts the similarities between the neural pathways in the human brain and a neural network. Neural networks can involve many variables and deal with multiple simultaneous pathways and outcomes. This may be quite useful for organizational planning, where workforce planning is embedded in an overall planning process that also incorporates production, marketing, and finance.
Your preview ends here
Eager to read complete document? Join bartleby learn and gain access to the full version
- Access to all documents
- Unlimited textbook solutions
- 24/7 expert homework help
Figure 5.14
Neural Pathways in the Human Brain and Neural Networks [8]
Random Forests
Like all forests, random forests begin with a single tree. A prediction tree begins with a single variable that is randomly selected from all possible predictor variables in the data; this is the root of the tree
.
In
Figure 5.15, the single variable (or root) is cognitive ability. This variable is then divided into two levels at the point that maximizes the difference in the outcome variable. (The numbers refer to the predicted values of the
Your preview ends here
Eager to read complete document? Join bartleby learn and gain access to the full version
- Access to all documents
- Unlimited textbook solutions
- 24/7 expert homework help
outcome variable [performance] based on the paths taken through the prediction tree.) For example, if the root variable is conscientiousness as a predictor of job performance, then the conscientiousness variable will be divided into two levels at the point that maximizes the difference in job performance between these two levels. The analysis continues by adding another level (or branch) to the tree, which is simply another predictor variable that has been randomly chosen from the data by the software. The analyst decides how many branches each tree should have, and the process continues until all the branches have been added to the tree. The completed tree shows how individuals are expected to score in terms of job performance based on their score on each of the predictors in the tree. However, this tree was built using only a few of the total number of variables in the data, and the variables were chosen at random. What if a
different set of predictor variables would make a better prediction? Rather than using a single tree, random forests build a model based on many trees, where each tree is based on a randomly selected group of predictors. In the end, the model is based on the average of the results of all the trees in the forest.
Your preview ends here
Eager to read complete document? Join bartleby learn and gain access to the full version
- Access to all documents
- Unlimited textbook solutions
- 24/7 expert homework help
Your preview ends here
Eager to read complete document? Join bartleby learn and gain access to the full version
- Access to all documents
- Unlimited textbook solutions
- 24/7 expert homework help
Figure 5.15
The tree from a random forest model begins with a single, randomly-selected
variable.Long Text Description
An advantage to random forest models is that they take advantage of the diversity of the variables in the data. A disadvantage to random forest models is that it is very difficult to know in the end which predictors are the most important or influential variables in predicting the outcome. The model would only show the analyst what the expected score
would be for each individual based on their responses to each of the predictor variables in the data.
There are many different types of supervised and unsupervised machine learning methods. The common aspects of machine learning models in general are that they learn by being trained on data where the predictors and the value of the outcome are already known, and then validated against a portion of the data that was withheld from the training stage. This ensures that the algorithm is predicting with a high degree of accuracy and that the algorithm has not been
over-trained
on the data. This is partly why the validation stage is so important when developing a machine learning model.
There are hundreds of machine learning methods, with more being developed all the time. Often, a data scientist will apply many different models on a set of data to find out what model does the best job of accurately predicting a desired outcome. We have taken
a brief look at machine learning models that categorize data to help uncover underlying structures within the data, and models that predict outcomes, to help identify trends and associations among data. As technology continues to drive down the cost of capturing data, the amount of data available has been increasing rapidly. Tools such as machine learning and artificial intelligence are becoming indispensable as aids to sifting and sorting through the growing mountains of data for knowledge. Learn more about the benefits of machine learning and artificial intelligence in HR Planning Today 5.2.2.
HR Planning Today 5.2.2: Machine Learning in Action
One of the great benefits of machine learning is that it can integrate a great deal of data from across different areas of the organization, and arrive at a more comprehensive, holistic solution than if planning was done in different areas at different times (Figure 5.16). For example, it would be ideal to model the organization's need for employees in all jobs given an expected level of production, and to incorporate estimates of employee turnover in a given period, how many will be needed for recruitment, what levels of promotion are most appropriate, and how much training to provide in order to meet those
production needs. Researchers who attempt to model these types of problems have discovered that it is very difficult to do, since the inputs to these pieces of information can
Your preview ends here
Eager to read complete document? Join bartleby learn and gain access to the full version
- Access to all documents
- Unlimited textbook solutions
- 24/7 expert homework help
vary between companies for many reasons. After all, it is more difficult to predict the actions of people.
Your preview ends here
Eager to read complete document? Join bartleby learn and gain access to the full version
- Access to all documents
- Unlimited textbook solutions
- 24/7 expert homework help
Your preview ends here
Eager to read complete document? Join bartleby learn and gain access to the full version
- Access to all documents
- Unlimited textbook solutions
- 24/7 expert homework help
Figure 5.16
Artificial intelligence is transforming work processes throughout organizations. [9]
One example of how machine learning can be used to integrate processes across the organization is demonstrated in a simulation study performed in 2022. This study modelled the maintenance needs of a factory production system along with the technical skills required for that maintenance. The researchers were able to determine the optimum level of job training, hiring, and promotion rates necessary to keep the assets used for production in proper working condition while at the same time weighing the competing costs of maintaining the system, the workforce costs, and the costs of delayed production due to malfunction (Akl et al. 2022, 108175).
In another, more focused study, researchers were interested in identifying the attributes that associate with turnover intentions among U.S. federal employees. Using a survey with a large sample size of almost 6,000 workers, the analyses helped to identify a set of
attributes that associated with individuals who have lower turnover intentions, including job satisfaction, satisfaction with opportunities for promotion, loyalty, and job tenure (Kang, Croft, and Bichelmeyer 2020, 538–558).
IBM uses artificial intelligence and machine learning in a different manner from either of the studies listed above. IBM assesses employee skills based on the passive observation of employee communications and work. The machine learning then recommends development and training opportunities to employees to strengthen skills and fill in gaps (Moore and Bokelberg 2019, 52–55).
These examples demonstrate the varied ways in which machine learning and artificial intelligence can be used to facilitate the planning process in organizations. The key to deriving the greatest benefit from these analytical tools lies in understanding what are the most important questions to ask, and what variables or processes are of the greatest importance in answering these questions. In other words, the benefits of big data techniques will be realized by bridging the skills from multiple disciplines such as data scientists, market researchers, operations experts, and HR professionals (Oswald, Behrend, Putka, and Sinar 2020, 505–533).
5.3 Qualitative Forecasting Techniques
Quantitative models can be very useful, especially in mature industries or where environmental changes are predictable or stable. When there is a great degree of uncertainty, qualitative models may be preferable. Qualitative models may also be useful
when no formal planning exists, and no formal data collection occurs around a planning process. Qualitative forecasting in the form of direct managerial input is the most commonly used method for determining workforce requirements (Ward 1996, 54–55).
5.3.1 Management Survey
Your preview ends here
Eager to read complete document? Join bartleby learn and gain access to the full version
- Access to all documents
- Unlimited textbook solutions
- 24/7 expert homework help
A large portion of Canadian companies use basic forms of human capital planning, and some do not forecast their demand for human capital at all (Cooper and Jackson 2017). Those firms that do no planning essentially hire on an as-needed basis. As jobs become open through attrition or expansion, the line manager notifies the HR department of the need to hire, and the recruitment process is initiated. Basic informal planning occurs when managers anticipate their needs over the near term, and submit requests based on
their knowledge of and past experience with the business.
Using experts to arrive at a numerical estimate of future labour demand is considered to be a more formal qualitative process for determining future labour requirements, because it is a detailed process of stating assumptions, considering potential organizational and environmental changes, and deriving a rationale to support the numerical estimate.
One of the issues with qualitative decision methods, as pointed out by Philip Tetlock (2005) in his book
Expert Political Judgment: How Good Is It? How Can We Know?
, is that experts are very often wrong. However, James Surowiecki (2005) finds that groups of people are very often correct. Qualitative forecasting methods such as the Delphi technique and nominal group technique (NGT) make use of groups of experts in an effort
to increase the validity and reliability of the forecast and of the information used to formulate the forecast. When a group of experts is involved in a forecasting exercise, each expert benefits from the knowledge and assumptions of all other experts involved. This way, the limitations of any single mental model used by any single expert are reduced by exposure to others. Furthermore, qualitative methods of forecasting such as these increase the reliability of the information by working through an iterative process where each expert has the opportunity to clearly explain his or her assumptions and decisions. This means that all experts share the same understanding of one another’s assumptions before arriving at a final forecast decision. In HR Notebook 5.3.1, learn about how organizations can broaden their applicant pools and develop a competitive advantage through their HR practices.
HR Planning Notebook 5.3.1: Diversity and Demand
The rate of growth in participation in the Canadian labour force for women and visible minorities is expected to outpace the rest of the labour market until the early 2030s. Because a majority of the new entrants into the labour force will be women and visible minorities, it is important for firms to develop diversity plans. Furthermore, Canada’s Employment Equity Program requires that all federally regulated firms (which include banks, telecommunications firms, air and rail transportation companies, and the federal government) produce annual reports of the representation of members of the four designated groups in their organizations, and plans to achieve full representation. Therefore, in addition to forecasting the demand for particular jobs, organizations should incorporate demand estimates for increasing participation among Canada’s designated
Your preview ends here
Eager to read complete document? Join bartleby learn and gain access to the full version
- Access to all documents
- Unlimited textbook solutions
- 24/7 expert homework help
groups (Figure 5.17). Diversity has the potential to increase organization-wide performance when diversity goals go beyond participation rates for protected groups, and incorporate the mental models used by members of protected groups when setting strategy and determining the core activities of the firm.
Your preview ends here
Eager to read complete document? Join bartleby learn and gain access to the full version
- Access to all documents
- Unlimited textbook solutions
- 24/7 expert homework help
Figure 5.17
The workplace should reflect the community in which the firm is embedded. [10]
Your preview ends here
Eager to read complete document? Join bartleby learn and gain access to the full version
- Access to all documents
- Unlimited textbook solutions
- 24/7 expert homework help
One way to understand diversity requirements is by comparing the diversity of the applicant pool and of the firm’s employees to that of the general population. If the diversity of the applicant pool or of employees is substantially different from the Canadian population, strategies can be developed to increase the recruitment and selection of members of designated groups.
Figure 5.18 shows that the labour market availability of women, Indigenous peoples, members of visible minorities, and persons with disabilities has risen in Canada over the past two decades. Organizations that can appeal to these groups are in a position to broaden their applicant pools and further develop a competitive advantage through their HR practices.
Figure 5.18
Labour Market Availability of Designated Groups, 1996 and 2016 (Employment and
Social Development Canada. 2020)
Source: Thomas and Ely (1996, 79–90)
Steeping some tea...
A wide variety of individuals may be considered experts for their knowledge of organizational operations, competitive HR practices, international trends in the labour markets, and so on (Anonymous 2005, 10–11; Gatewood and Gatewood 1983, 83–94). First and foremost, the organization’s own line managers, who each have detailed knowledge of workload, responsibilities, and overall task responsibilities for a department, possess important insights into how future demand for labour should or might change in the manager’s own areas of responsibility. Second, the organization’s HR and line managers certainly have critical information to provide guidance in forecasting future levels of labour demand. For example, the planning staff may use econometric and strategic models to predict the future level of sales of, or demand for, the organization’s goods and services, as well as provide important insights into future economic indicators affecting labour demand such as interest rates, change in gross national product, level of consumer disposable income and savings, and so on. The HR staff, whether they are HR generalists or a team of HR planning specialists, are able to draw up a detailed set of assumptions with respect to industry, local, and international
Your preview ends here
Eager to read complete document? Join bartleby learn and gain access to the full version
- Access to all documents
- Unlimited textbook solutions
- 24/7 expert homework help
labour market trends that affect how the organization organizes and employs its own workforce. Third, business consultants, financial analysts, university researchers, union staff members, industry spokespersons, and others possess detailed knowledge of specific industries or types of organizational activity and are able to give rich, detailed, and largely impartial judgments on future labour demand because of their external perspective relative to the organization. Finally, but not exclusively, federal, provincial, and local governmental staff and officials are important individuals to consult, because they possess knowledge of future environmental changes in labour and business legislation that can dramatically change labour demand not only for a specific organization but also for the industry in general. For example, pending legislation to ban the use of certain materials in product manufacturing might cause a substantial drop in demand for these products and hence an associated reduction in demand for employees
who are involved in their manufacture.
Government ministries and departments, most specifically those devoted to labour, HR, and economic development, as well as Statistics Canada, can provide expert information
for the labour demand forecasting process. Interviews, questionnaires (conducted in person or by mail or email), and conference calls are some of the options available for gathering information from experts, but other techniques can be employed to maximize the benefit of each expert’s contributions in specific circumstances. We now turn our attention to some of these methods of facilitating high-quality labour demand forecasts.
5.3.2 Scenario Planning
Scenario planning
is a method often used to develop organizational strategy. A primary strength of scenario planning as a strategy-setting tool is that it encourages participants to develop strongly shared mental models of future organizational states. Scenario planning is a method for imagining future possible conditions in which the organization might operate. As a technique, it requires participants to challenge existing assumptions and to generate vivid pictures of possible future states. The general process of scenario planning is as follows:
1.
Propose the forecasting question about the future state of the firm or -
environment. For example, “How many households will own an electric car in 10 years?”
2.
Generate a list of factors that are likely to influence the outcome in question. It is often useful to perform a SWOT analysis that takes into account factors such as the economy, the political landscape, society, and the impact of technology.
3.
Sort the factors into naturally occurring groups and rank the groups according to their importance to the main question and the ability of the
Your preview ends here
Eager to read complete document? Join bartleby learn and gain access to the full version
- Access to all documents
- Unlimited textbook solutions
- 24/7 expert homework help
firm to control the factor. Factors that the firm has less ability to control should receive higher rankings.
4.
Select the two groups of factors that are likely to have the strongest and
most unpredictable impact on the question. Create four quadrants (refer
to Figure 5.19) by stretching one group along a continuum from its extreme negative condition to its extreme positive condition on the x-
axis, and stretching the other group along a continuum from its extreme low to high conditions on the y-axis.
5.
Name and describe in story form each of the four worlds in the four resulting quadrants.
6.
Suggest the skills, competencies, and other organizational requirements that would be necessary for the firm to be able to operate in each of these four worlds.
7.
Generate a demand forecast necessary to fulfill the firm’s requirements in each of the four worlds.
Your preview ends here
Eager to read complete document? Join bartleby learn and gain access to the full version
- Access to all documents
- Unlimited textbook solutions
- 24/7 expert homework help
Figure 5.19
Scenario planning is often used to develop organizational strategy.Long Text
Description
The process of describing each of the proposed worlds helps participants to understand ways in which current mental models are influencing thinking and action, and to develop new shared mental models. The benefit that scenario planning brings to demand forecasting is that while there is really no limit to the number of possible scenarios that the future could hold, this method allows planners to understand the most important assumptions that go into each demand forecast. Understanding what assumptions are the most influential for a demand forecast helps the planner to know how sensitive the forecast is to each assumption. For example, if the scenario planning exercise suggests that the driving range and passenger capacity of electric cars are the factors that will most influence the future demand for electric cars, then demand estimates for the future number of employees required to produce electric cars will be most sensitive to changes in these assumptions. In other words, potentially small changes to these assumptions
Your preview ends here
Eager to read complete document? Join bartleby learn and gain access to the full version
- Access to all documents
- Unlimited textbook solutions
- 24/7 expert homework help
could bear large differences in the demand estimates for employees. Scenario planning therefore assists planners in developing a variety of future demand scenarios, and helps planners to understand how much leeway exists in any one demand forecast before that forecast is no longer viable (Korte and Chermack 2006, 645–656).
This flexible demand forecasting process is very useful for incorporating the effects of uncertainty and change into the strategic HR planning process. Each of the scenarios or predicted future states contains its own set of assumptions, resulting in an entirely different estimate presented in a single staffing table for each specific course of action. In
this way, an organization’s HR staff are able to develop – with the associated staffing tables – future scenarios that are optimistic (e.g., sales levels will increase by 10 to 15 percent), realistic or most likely (e.g., sales levels will increase by 5 to 10 percent), or pessimistic (e.g., sales levels will remain constant or increase by less than 5 percent). By
means of this comprehensive, explicit set of staffing tables, which reflects a wide variety of future organizational circumstances, the scenario planning technique allows ready access to flexible, pre-planned demand estimates when circumstances rapidly change.
Steeping some tea...
Steeping some tea...
5.3.3 Delphi Technique
The
Delphi technique
, named after the Greek oracle at Delphi and developed by N. C. Dalkey and associates at the Rand Corporation in 1950, is another useful qualitative method for deriving detailed assumptions of long-run HR demand (Passmore, Cebeci, and Baker 2005, 33–49; Luthans 1992). This forecasting technique is “a carefully designed program of sequential, individual interrogations (usually conducted through
Your preview ends here
Eager to read complete document? Join bartleby learn and gain access to the full version
- Access to all documents
- Unlimited textbook solutions
- 24/7 expert homework help
questionnaires) interspersed with feedback on the opinions expressed by the other participants in previous rounds” (Helmer 1999, 47–58). Its key feature is that once a group of experts is selected, the experts do not meet face to face (Passmore,
Cebeci, and Baker 2005, 33–49
;
Fusfeld and Foster 1971, 63–74
)
. Instead, a project coordinator canvasses them individually for their input and forecasts by means of a progressively more focused series of questionnaires.
Advantages.
The advantage of the Delphi technique is that it avoids many of the problems associated with face-to-face groups, namely reluctance on the part of individual experts to participate due to (1) shyness, (2) perceived lower status or authority, (3) perceived communication deficiencies, (4) issues of individual dominance and groupthink (i.e., group conformity pressures), and so on (Loo and Thorpe 2003, 824–825; Hampton, Summer, and Webber 1987; Milkovich, Annoni, and Mahoney 1972, 381–388; Milkovich and Mahoney 1978, 19–30). Because the Delphi technique does not employ
face-to-face meetings, it can serve as a great equalizer and can elicit valid feedback from all expert members. It is also advantageous that the
Delphi technique can effectively use experts who are drawn from widely
dispersed geographical areas (Bramwell and Hykawy 1999, 47–58).
Disadvantages.
There are disadvantages associated with the Delphi technique, as there are with all forecasting techniques. In particular, because of the series of questionnaires administered to derive a forecast, the time and costs incurred when using the Delphi technique can be higher than those incurred when using alternative forecasting methods. Another deficiency is that since the results cannot be validated statistically, the process is greatly dependent on the individual
knowledge and commitment of each of the contributing experts (Loo and Thorpe 2004, 88–97; Chan et al. 2004, 31–56; Meehan and Ahmed
1990, 297–307). Furthermore, if the experts are drawn from one specific field, their common professional training might guide them along a single line of inquiry (i.e., follow a single mental model) rather than pursuing more innovative and creative courses of action. Finally, if insufficient attention has been paid to developing criteria for the identification and selection of experts, the people selected to derive the
Your preview ends here
Eager to read complete document? Join bartleby learn and gain access to the full version
- Access to all documents
- Unlimited textbook solutions
- 24/7 expert homework help
demand forecasts may lack sufficient expertise or information to contribute meaningfully to the process (Bramwell and Hykawy 1999, 47–58).
Figure 5.20 highlights the six steps associated with using the Delphi technique for HR demand forecasting.
Your preview ends here
Eager to read complete document? Join bartleby learn and gain access to the full version
- Access to all documents
- Unlimited textbook solutions
- 24/7 expert homework help
Your preview ends here
Eager to read complete document? Join bartleby learn and gain access to the full version
- Access to all documents
- Unlimited textbook solutions
- 24/7 expert homework help
Figure 5.20
Delphi Technique for HR Demand ForecastingLong Text Description
1.
Define and Refine the Issue or Question.
During this stage, a project coordinator is assigned, and they work with the HR staff to determine the specific job or skills category or activity that will be the focus of the Delphi technique. It is essential that the group targeted for HR forecasting be well defined so that relevant, focused, and detailed feedback based on a minimum of assumptions (redundant assumptions
are associated with loss of the experts’ time) can be derived.
2.
Identify the Experts, Terms, and Time Horizon.
The project coordinator, normally in conjunction with the HR staff, identifies and selects a team of individuals deemed to be experts with respect to the specific human capital grouping that requires a forecast. The number of experts to include in an expert panel typically range between seven and
15 (Sobaih, Ritchie, and Jones 2012, 886–906). Next, given that in many cases the group of experts will include individuals who are not members of the organization, it is important for both parties to reach agreement on the terms and conditions for participation in the forecasting process, as well as setting the context and explicitly defining
the nature of the work. For example, the team of experts must be absolutely clear on which jobs constitute “production workers” if those experts are being asked to derive a demand forecast for this category. Similarly, the exact time horizon(s) must be specified for the human capital category being analyzed.
3.
Orient the Experts.
In addition to identifying the relevant time horizon(s) and clarifying which employee groups are of interest, the orientation process for experts includes an overview of the demand forecasting decision process (which is very similar to the structural framework in which you are now engaged!). The experts are told either that there will be a predetermined number of questionnaire iterations or that the sequence will continue until a majority opinion exists among the
experts.
4.
Issue the First-Round Questionnaire.
The project coordinator sends each expert the questionnaire by courier, fax, mail, or email and
Your preview ends here
Eager to read complete document? Join bartleby learn and gain access to the full version
- Access to all documents
- Unlimited textbook solutions
- 24/7 expert homework help
includes a time frame for completing and returning it. Typically, this first questionnaire focuses on defining both the explicit assumptions made by each of the experts and the background rationale supporting his or her particular demand estimate.
5.
Issue the First-Round Questionnaire Summary and the Second Round of Questionnaires.
Following the completion of the first questionnaire, the project coordinator sends the second and subsequent rounds of questionnaires to the experts with a written summary of the findings from the previous round. The aim of the subsequent questionnaires is to focus the experts’ initial assumptions and estimates by providing summarized feedback from all members of the group. Points of commonality and conflict are identified in the summary, as is the need to clarify specific assumptions identified by the
responses to the previous round.
6.
Continue Issuing Questionnaires.
The project coordinator continues to issue questionnaires until either all the predetermined questionnaire stages have been completed and summarized or the group reaches a clear majority decision. In either case, the majority or
n
th-round summary summarizes the experts' future demand estimate for the HR category under analysis.
HR Planning Today 5.3.1 provides more details on the Delphi technique.
HR Planning Today 5.3.1: The Delphi Technique in Action
The Delphi technique is used in a wide variety of applications for HR forecasting. For example, an agricultural research organization started by deriving nine organizational core competencies from interviews and internal organizational documentation. The experts were identified by the organization, which asked them to respond to a set of questionnaires based on the Delphi technique. The questionnaires investigated
1.
the importance of human competencies in the future,
2.
the capacity of the organization’s current human resources, and
Your preview ends here
Eager to read complete document? Join bartleby learn and gain access to the full version
- Access to all documents
- Unlimited textbook solutions
- 24/7 expert homework help
3.
ranking the nine organizational core competencies in order of priority.
HR policy interventions were developed from the resulting information.
A three-round Delphi procedure was used to identify the basic competencies of research
chefs, who develop new products, create new recipes, and conduct food testing. Thirty-
three expert chefs were involved in the Delphi undertaking, and they were asked questions concerning
1.
factors differentiating successful from less successful research chefs,
2.
knowledge and skills required by successful research chefs, and
3.
how tasks for a research chef differ from those of an ordinary chef.
The experts identified 19 basic competencies that a successful research chef should possess.
Finally, subsequent to the September 11, 2001, terrorist attacks on the United States, the Delphi technique was used by the government and insurance and risk managers to estimate the possibility of future losses due to terrorism, and the likely types of terrorist acts various organizations might experience. Experts used the Delphi technique to analyze databases on landmarks, tourist attractions, “vital points,” and property assessment data to come up with their forecasts.
Sources: Guimaraes et al. (2001, 249–255); Birdir and Pearson (2000, 205–209); Coffin (2000, 8–9)
Steeping some tea...
Your preview ends here
Eager to read complete document? Join bartleby learn and gain access to the full version
- Access to all documents
- Unlimited textbook solutions
- 24/7 expert homework help
Steeping some tea...
Steeping some tea...
5.3.4 Nominal Group Technique
Your preview ends here
Eager to read complete document? Join bartleby learn and gain access to the full version
- Access to all documents
- Unlimited textbook solutions
- 24/7 expert homework help
Figure 5.21
Expert opinion can be used to
prepare estimates. [11]
Although the
nominal group technique (NGT)
is also a long-run qualitative demand forecasting method, it differs from the Delphi technique in several important respects. First, unlike in the Delphi technique, the group does, in fact, meet face to face and interact, but only after individual, written preparatory work has been done and all the demand estimates (idea generation) have been publicly tabled, or written on a flip chart, without discussion (Figure 5.21) (Fraser and Fraser 2000, 228–243; Van de Ven 1974). Second, each demand estimate is considered to be the property of the entire group and to be impersonal in nature, which minimizes the potential for dominance, personal attacks, and defensive behaviour in support of estimates presented in the group forum (Rohrbaugh 1981, 272–288). Finally, the expert forecast is determined by a secret vote of all group members on their choice of the tabled demand forecasts. The estimate receiving the highest ranking or rating during the voting process is deemed to be the group’s forecast (Fraser 2000, 29–36; Delbecq, Van de Ven, and Gustafson 1975; Green 1975, 63–73). A primary advantage to NGT is that it allows all participants to contribute to the process at an equal level by reducing the tendency for more extroverted
individuals to dominate the process (Roeden, Maaskant, and Curfs 2012, 588–593).
Your preview ends here
Eager to read complete document? Join bartleby learn and gain access to the full version
- Access to all documents
- Unlimited textbook solutions
- 24/7 expert homework help
Refer to HR Planning Today 5.3.2 for more information on the NGT. Readers are invited to experience a nominal group technique as part of a group exercise at the end of this chapter.
HR Planning Today 5.3.2: Assessing the Utility of the
Nominal Group Technique
Studies have shown that nominal group technique (NGT) is especially effective for brainstorming sessions to ensure all participants have an equal voice in the sessions, and when a problem or issue stems from several widely diverse causes. Furthermore, studies have shown that NGT provides highly reliable and valid qualitative data that is ranked by importance and is superior to that derived from focus group sessions. Nominal
group sessions investigating teaching performance competencies on 13 dimensions (technical knowledge, planning and organizing, managing interaction, commitment to teaching objectives, proactive orientation, student development orientation, class presentation ability, impact on the class, adaptability and flexibility, personal motivation, listening skills, oral communication skills, and presentation skills) were dramatically superior to information obtained from focus groups. The study predicted that NGT will replace focus groups as the qualitative research method of choice and will reduce the need for the administration of surveys.
Sources: Andersen and Fagerhaug (2000, 144–145); Langford, Schoenfeld, and Izzo (2002, 58–70)
Steeping some tea...
Your preview ends here
Eager to read complete document? Join bartleby learn and gain access to the full version
- Access to all documents
- Unlimited textbook solutions
- 24/7 expert homework help
Steeping some tea...
Steeping some tea...
Steeping some tea...
5.3.5 HR Budgets/Staffing Tables
HR budgets
are quantitative, operational, or short-run demand estimates that contain the number and types of jobs or positions required by the organization as a whole and for each subunit, division, or department. Table 5.5 provides a hypothetical example for the firm “Ennotville Eateries” (McBeath 1992).
These HR budgets, prepared by the HR staff in conjunction with line managers, take into consideration information from historical company staffing trends, competitor staffing practices, industry and professional associations, and Statistics Canada.
Table 5.5 Staffing Table and HR Budget, Ennotville Eateries
Staff Demand Sales ($1–
Sales ($10–
Sales (>$25–
Sales (>$50–
Your preview ends here
Eager to read complete document? Join bartleby learn and gain access to the full version
- Access to all documents
- Unlimited textbook solutions
- 24/7 expert homework help
Requirements
10 million)
25 million)
50 million)
75 million)
Administrative Positions
President
1
1
1
1
Vice-presidents
1
1
2
3
Marketing managers
1
1
2
2
Sales staff
4
7
10
18
HR staff
2
4
5
7
Treasurer
1
1
1
2
Financial staff
3
5
7
9
Clerical and general staff
5
8
12
14
Executive chef
1
1
1
1
Production Positions
Chef
2
4
5
6
Cook
8
15
25
35
Haggis helper
10
20
30
40
Saucier
1
3
5
6
The HR budget process produces a
staffing table
, which contains information related to a specific set of operational assumptions or levels of activity (e.g., maintain the current organization structure, increase the sales level by 5 percent over last year’s level). The staffing table presents the total HR demand requirement, laid out in terms of the number of people required by level (e.g., vice-presidents) and function (e.g., marketing).
In this way, HR planners can determine short-run future demand requirements for subunits and the organization as a whole (Figure 5.22). This enables budgeting processes to incorporate changes in compensation costs linked to the level of future human capital demand.
Your preview ends here
Eager to read complete document? Join bartleby learn and gain access to the full version
- Access to all documents
- Unlimited textbook solutions
- 24/7 expert homework help
Figure 5.22
Using a variety of indices, HR professionals can estimate the number and type of
human capital needed. [12]
Your preview ends here
Eager to read complete document? Join bartleby learn and gain access to the full version
- Access to all documents
- Unlimited textbook solutions
- 24/7 expert homework help
Steeping some tea...
5.4 Combining Quantitative and Qualitative Methods
Quantitative and qualitative methods are not exclusive methods where the forecaster decides to use one method at the expense of the other. These two basic methods can both be used in order to test the reliability of the forecast. If both methods arrive at similar forecasts, then planners can be more certain of the results. However, if one method leads to dramatically different results from the other, then it would be reasonable
to question the assumptions of both methods to try to understand where differences in assumptions lead to discrepancies in the forecasts.
HR Planning Notebook 5.4.1 demonstrates that the different types of information that quantitative and qualitative methods use as inputs to the forecast can arrive at complementary findings. By using both methods of analysis, planners can arrive at a richer, more detailed understanding of the demand for labour and of how the factors that affect demand can change those estimates. In addition to using both quantitative and qualitative methods in parallel, as discussed above, these methods can be combined to form a very powerful method of forecasting known as simulation.
HR Planning Notebook 5.4.1: Differences between Qualitative and Quantitative Forecasting Data
Quantitative analysis and qualitative analysis can each provide information that complements the other. The kinds of data that are used for each type of analysis are different, and these differences lead to different perspectives on issues. Ideally, both qualitative and quantitative analyses should suggest similar demand forecasts. When this occurs, planners can have more confidence in their processes. However, when the two methods offer very different solutions, planners should investigate where these differences are coming from in their data, and seek to resolve the differences (refer to
Your preview ends here
Eager to read complete document? Join bartleby learn and gain access to the full version
- Access to all documents
- Unlimited textbook solutions
- 24/7 expert homework help
Table 5.6 and Table 5.7). In general, quantitative and qualitative methods can be compared based on the types of data that are used for each method, and the kinds of results that each method tends to produce.
Table 5.6 Qualitative and Quantitative Forecasting Data Differences: Type of Data
Quantitative
Qualitative
Economic Outlook
Economic Outlook
Labour-force growth
Demographics
Labour-force projections
Political and regulatory environment
Industry projections
Technological change
Occupational projections
Societal change
Table 5.7 Qualitative and Quantitative Forecasting Data Differences: Type of Results
Quantitative
Qualitative
Detailed, numeric estimates
More specific information that can easily be used to produce HR system development plans
Source: Bartlett, Johnson, and Schneider (2016, 440–461).
5.4.1 Simulation
Simulation
is a powerful blend of quantitative and qualitative analysis. It is based on the creation of a set of assumptions around the variables or inputs that are expected to affect demand followed by a quantitative representation of those assumptions and their interactions. These assumptions start at the macro level (e.g., the economy, changes in technology, political or legislative outcomes, or demographics), and then incorporate organizational variables that could also influence demand such as the impact of technology or process changes in production. Simulation can be used to model both demand and supply, so models can also include supply-related variables such as the amount of time required for training someone to become competent at a job level, and seniority levels of employees in the job.
The assumptions that are used to build a simulation model employ mathematical algorithms that reflect how variables are expected to react dynamically with the other variables in the model. For example, the stock of employees in a job may not accurately represent the level of skilled employees in that job at any given point in time. If a job requires a lengthy training or mentoring period, then the flow of employees may be suitable, but stocks of suitably skilled employees may be low or high for the firm’s needs depending on where employees are in the training cycle. These types of delays and
Your preview ends here
Eager to read complete document? Join bartleby learn and gain access to the full version
- Access to all documents
- Unlimited textbook solutions
- 24/7 expert homework help
accumulation processes lead to relationships with other demand characteristics such as revenues or production that are not linear. Simulation can model these nonlinear dependencies, and so simulation has an advantage over regression and trend-based models for these types of applications.
The simulation process can be broken down into several steps:
1.
Using qualitative methods, collect the relevant variables. This process can use interviews, focus groups, Delphi technique, or NGT to collect the information.
2.
Describe how these variables interact together to by developing a process model to map the relationships between variables. Line managers who have direct experience with the manner and ways in which the demand for human capital changes over time and with sales are in an excellent position to provide insights for this process.
3.
Use simulation software and develop the algorithms to estimate the model. The range of assumptions developed qualitatively are used to run the simulation several thousand times. The output of the simulation presents a range of outcomes that occur most of the time. This range of
outcomes represents the envelope of estimates over which a demand estimate is likely to occur given the current set of assumptions.
4.
Test the model using historical data to validate the assumptions used in
its development.
5.
Different assumptions can be easily tested in the model by inputting new scenarios and re-running the simulation.
The main benefit of simulation in forecasting demand (and supply) is not in arriving at a more accurate forecast, but in developing a better understanding of what factors influence demand and supply, and what processes may be causing bottlenecks in the flow of human capital. In this sense, simulation is considered to be more
descriptive
of processes than
prescriptive
(Grossler and Zock 2010, 829–848)
.
The advantage of simulation is in providing knowledge around how demand estimates might react to changes in environmental factors, in customer characteristics, in the training or skills requirements of employees, or in any of the assumptions that are used to build the simulation model. While simulation forecasting is relatively new to the HR field, it has been used for a decade or more in the areas of operations and finance. HR practitioners in large organizations might be able to team with operations and/or the finance department to develop integrated simulation models that incorporate product demand with manufacturing constraints and human capital demand and supply to streamline the
Your preview ends here
Eager to read complete document? Join bartleby learn and gain access to the full version
- Access to all documents
- Unlimited textbook solutions
- 24/7 expert homework help
flow of resources across the firm. This kind of integration between customer needs and organizational resources can lead to faster response times for organizations to provide services or products to customers, which has the potential to provide a source of competitive advantage through human capital planning, as discussed in HR Planning Today 5.4.1.
HR Planning Today 5.4.1: Simulation in the Skilled Services Industries
Industries such as electric power utilities, home and industrial heating and cooling, electrical, and plumbing all require highly skilled workers, and all involve unpredictable and volatile demand. When the Quebec ice storm of 1998 downed over 1,000 electrical transmission towers, it left over a million Canadians without heat and water for days, and
presented a large threat to the Canadian dairy industry, which was unable to feed or milk
cows.
Shocks such as these may be rare, but the industries that deal with such events must be
able to provide the services necessary to recover quickly. Planning must include scenarios for these rare occurrences. At a smaller scale, service providers for those services we consider to be necessary, such as heating and air conditioning, are in a better position to service their customers when they have skilled technicians available whenever their customers have a need. However, the more ready a company is with technicians, the higher the potential for lost revenues from a surplus of technicians when demand is not at its peak. Therefore, having the right number of technicians available is a complex capability that could act as a source of strategic competitive advantage.
In the home heating/cooling industry in Canada, the demand for services is growing at a relatively stable rate, as demand is a function of the number of homes. However, the time required to develop a skilled technician to the point of being available for active service can be quite long, when considering the time to recruit and provide basic and on-
the-job training. Lead times can extend beyond 24 months for some jobs for highly skilled technicians. Modelling the demand for such jobs must therefore account for not only the stock of technicians, but also the flow of technicians from recruitment to the point of being fully active in the job. A simulation model will include variables such as the recruitment rate (the number of technicians being hired), the number of employees taking basic training as well as the number of active employees working as trainers, the number of employees in the on-the-job training phase, the number of active employees, and the time it takes for employees to move from one phase to the next. Additional complications to this model include estimating the number of employees lost at each point of the cycle, as well as the number of trainers who must be removed from active service to perform basic training. As the demand for employees increases, the demand for trainers will increase to respond to the need to train the increased numbers of
Your preview ends here
Eager to read complete document? Join bartleby learn and gain access to the full version
- Access to all documents
- Unlimited textbook solutions
- 24/7 expert homework help
employees in basic training. Each phase of this model will have a mathematical equation
that is intended to simulate changes in the variables over time, and their relation to other variables in the model.
Simulation models require skills in mathematics and logistics to develop the algorithms used, but the conceptual basis of the model and the assumptions around the building of the model require as much involvement from HR and the business line.
Source: Grossler and Zock (2010, 829–848).
Summary
L.O. 5.1
While demand forecasts will never be exact, the dramatic increase in the kinds of data available to HR planners today offers the potential to increase the precision (and complexity) of quantitative demand models. There are various quantitative, qualitative, and blended techniques that organizations use to forecast future requirements for HR demand.
L.O. 5.2
Quantitative models are most useful when the past is expected to be a good predictor of the future. Models can range from a simple trend analysis or regression analysis to more complex machine learning methods that incorporate data about customers, employees, and production. While some of these models can be complex, the key to their success is understanding what the drivers of demand are and how HR practices and employee attitudes and behaviours influence these processes.
L.O. 5.3
Qualitative methods range from a single manager’s estimate to methods that challenge current assumptions like scenario planning, to models that increase the validity
and reliability of expert judgments such as Delphi technique and nominal group technique (NGT). Delphi and NGT are versatile methods that can also be used to help groups brainstorm or achieve consensus. HR practitioners should consider using these methods not only for planning but also to gather the kinds of information that could otherwise be collected using focus groups or surveys.
LO. 5.4
Demand forecasts are imperfect. HR planners must understand the assumptions
that form the basis of their forecasts and the sensitivity of the forecast to deviations from these assumptions. Ideally, deviations from the forecast can be managed by HR practices such as flexible work arrangements, part-time workers, contract workers, etc. However, when the assumptions are incorrect by a significant amount, contingency forecasts and plans must be put in place. Good planning involves the development of contingency plans, the ability to detect as early as possible when the current forecast
Your preview ends here
Eager to read complete document? Join bartleby learn and gain access to the full version
- Access to all documents
- Unlimited textbook solutions
- 24/7 expert homework help
can no longer satisfy actual demand using current HR practices, and when a contingency plan must be put into action.
Glossary
Correlation
Describes the relationship between two variables.
Delphi technique
A process in which the forecasts and judgments of a selected group of experts are solicited and summarized in an attempt to determine the future HR demand.
HR budgets
Quantitative, operational, or short-run demand estimates that contain the number and types of jobs required by the organization as a whole and for each subunit, division, or department.
Neural networks
Made up of many nodes, where a node is a point at which information arrives and is acted upon before being passed on as another piece of information for another node.
Nominal group technique (NGT)
A long-run forecasting technique utilizing expert assessments.
Ratio analysis
A quantitative method of projecting HR demand by analyzing the historical relationship between an operational index and the number of employees required.
Regression analysis
A forecasting method that predicts the value of a variable from what is known historically about the relationship between that variable and one or more other variables.
Over-trained
Also called over-fitting, occurs when the model has been trained to almost perfectly predict the training data but has trained itself so well for the nuances of those particular data that the model does a poor job of predicting different data.
Scenario planning
A method for imagining future possible organizational states and the
resulting capabilities, activities, or strategies that are necessary to be successful in those
future states.
Shared variance
The correlation coefficient squared; allows us to understand how much
of one variable can be predicted by the other variable.
Simulation
A blend of qualitative and quantitative modelling that incorporates a set of assumptions about relationships among variables in a mathematical algorithm. Simulation can simultaneously model demand and supply, and is very useful for testing the impact of assumptions on the outcome of the model.
Staffing table
Total HR demand requirement for operational or short-run time periods.
S
tructural equation modelling (SEM)
A statistical technique that permits the testing of multiple relationships simultaneously in a theoretically derived model.
Trend analysis
A forecasting method that extrapolates from historical trends.
Discussion Questions
Your preview ends here
Eager to read complete document? Join bartleby learn and gain access to the full version
- Access to all documents
- Unlimited textbook solutions
- 24/7 expert homework help
Steeping some tea...
Steeping some tea...
Steeping some tea...
Exercise
Question 5.31
Show Correct Answer
The question: Will undergraduate university courses in Canada move more toward online course offerings in the next five years, or will there be a gradual reduction in online courses?
a.
On your own, or in groups of four to five people, write down all the factors that you can think of that might influence the move toward increasing or decreasing the use of online courses. Write each factor on a separate sticky note. Think of how political, economic, environmental, social, technological, demographic, and legal/regulatory issues could influence the move toward or away from online courses over the next five years. Write each factor down as you think of it, and do not critically examine the factors yet.
Your preview ends here
Eager to read complete document? Join bartleby learn and gain access to the full version
- Access to all documents
- Unlimited textbook solutions
- 24/7 expert homework help
b.
Consider your factors, and move the sticky notes into groups of factors that form natural clusters. Give each cluster a title.
c.
Select the two clusters that you think have the potential to have the most impact on the decision to
increase online course offerings and that are also unpredictable.
d.
Now stretch these two clusters out. Place the two extremes of the first cluster at either end of the horizontal axis, and the two extremes of the second cluster at each end of the vertical axis. This will leave you with four quadrants: the top right quadrant will represent a world in which both clusters are
at the extreme positive end of their scales. The bottom right quadrant will represent a world in which the horizontally placed cluster is at the top end of its scale, but the vertically placed cluster is at the negative end of its scale, and so on.
e.
Consider the world that is represented by each quadrant, and describe what each world would be like. How does online education fit into this world? Give each world its own representative name.
f.
Describe the types of strategies, resources, and activities that would be necessary for a university course to be successful in each of these worlds.
g.
Consider the strategies, resources, and activities that are common to all four worlds. Any attributes that are common to all four worlds are likely to lead to success in any outcome that is close to what your scenario planning model presents. Therefore, these are the attributes that universities should be focusing on today so that they can be successful in presenting strong programs and class environments to students in the near future.
h.
Discuss your findings with other groups or individuals. How are they similar, and how do they differ? What were the critical differences in your assumptions that affected the outcomes?
Upload a PDF to submit the exercise.
Students can browse their computer or drag and drop file
Max file size:
50MB
Accepted file types:
PDF, JPG, JPEG, PNG
References
Akl, A.M., S. El Sawah, R. K.Chakrabortty, and H. H. Turan. 2022. "A Joint Optimization of Strategic Workforce Planning and Preventive Maintenance Scheduling: A Simulation–
Optimization Approach."
Reliability Engineering & System Safety
219
:
108175. https://doi.org/10.1016/j.ress.2021.108175.
Al-Subhi Al-Harbi, K. M. 2000. “Optimization of Staff Numbers in the Process Industries: An Application of DEA.”
International Journal of Manpower
21 (1): 47–55. https://doi.org/10.1108/01437720010319453.
Andersen, B., and T. Fagerhaug. 2000. “The Nominal Group Technique.”
Quality Progress
22 (2): 144–145.
Anonymous. 2005. “Tool Forecasts Labour Needs.”
On-Site
49 (6): 10–11.
Bartlett, K. R., K. R. Johnson, and I. E. Schneider. 2016. “Comparing Strategic Human Resource Development Approaches for Tourism and Hospitality Workforce Planning.”
Journal of Human Resources in Hospitality and Tourism
15 (4): 440–461. https://doi.org/10.1080/15332845.2016.1148569.
Your preview ends here
Eager to read complete document? Join bartleby learn and gain access to the full version
- Access to all documents
- Unlimited textbook solutions
- 24/7 expert homework help
Birdir, K., and T. Pearson. 2000. “Research Chefs’ Competencies: A Delphi Approach.”
International Journal of Contemporary Hospitality Management
12 (3): 205–
209. https://doi.org/10.1108/09596110010309989.
Boudreau, J. W. 2020. "Jobs Are Melting into Fluid Work."
USC Center for Effective Organizations
. Center for Effective Organizations. Accessed August 19, 2022. https://ceo.usc.edu/2020/09/29/jobs-are-melting-jobs-into-fluid-work/.
Bramwell, L., and E. Hykawy. 1999. “The Delphi Technique: A Possible Tool for Predicting Future Events in Nursing Education.”
Canadian Journal of Nursing Research
30 (4): 47–
58.
Cascio, W.F. 1991.
Applied Psychology in Personnel Management
, 4th ed. Englewood Cliffs, NJ: Prentice Hall.
Chan, C. et al. 2004. “Nursing Crisis: Retention Strategies for Hospital Administrators.”
Research and Practice in Human Resource Management
12 (2): 31–56.
Coffin, B. 2000. “Forecasting Terrorism Losses.”
Risk Management
49 (11): 8–9.
Cooper, J., and S. Jackson. 2017.
Workforce Planning Practices in Canada: Human Resources Trends and Metrics
, 4th ed. The Conference Board of Canada.
Delbecq, A.L., A. H. Van de Ven, and D. H. Gustafson. 1975.
Group Techniques for Program Planning
. Glenview, IL: Scott Foresman.
Elbo, R. 2000. “In the Workplace: Competing with Poaching Employers.”
Businessworld
1–
3.
Employment and Social Development Canada. 2020.
2016 Employment Equity Data Report
. www.canada.ca. Table 1: National labour market availability (percent).
November 4, 2020. https://www.canada.ca/en/employment-social-
development/corporate/portfolio/labour/programs/employment-equity/reports/2016-
annual.html#es.
Fraser, C., and A. Fraser. 2000. “Measuring the Performance of Retail Managers in Australia and Singapore.”
International Journal of Retail & Distribution Management
28 (6): 228–243. https://doi.org/10.1108/09590550010328409.
Fraser, C. 2000. “The Influence of Personal Characteristics on Effectiveness of Construction
Site Managers.”
Construction Management & Economics
18 (1): 29–36. https://doi.org/10.1080/014461900370924.
Your preview ends here
Eager to read complete document? Join bartleby learn and gain access to the full version
- Access to all documents
- Unlimited textbook solutions
- 24/7 expert homework help
Gatewood, R. D., and E. J. Gatewood. 1983. “The Use of Expert Data in Human Resource Planning: Guidelines from Strategic Forecasting.”
Human Resource Planning
6 (2): 83–
94.
Green, T. B. 1975. “An Empirical Analysis of Nominal and Interacting Groups.”
Academy of Management Journal
18 (1): 63–73. https://doi.org/10.2307/255625.
Grossler, A., and A. Zock. 2010. "Supporting Long-Term Workforce Planning with a Dynamic Aging Chain Model: A Case Study from the Service Industry."
Human Resource Management
49 (5): 829–848. https://doi.org/10.1002/hrm.20382.
Guimaraes, T. A, J. E. Borges
‐
Andrade, M. dos Santos Machado, and M. Ramos Maia Vargas. 2001. “Forecasting Core Competencies in an R&D Environment.”
R&D Management
31 (3): 249–255. https://doi.org/10.1111/1467-9310.00213.
Hampton, D.R., C. E. Summer, and R. A. Webber. 1987.
Organizational Behavior and the Practice of Management
, 5th ed. Glenview, IL: Scott Foresman.
Heaven, P. 2022. "Canada Faces Record Wave of Retirements as It Grapples with Historic Labour Shortage
." Financial Post
. Accessed August 19, 2022. https://financialpost.com/news/economy/canada-faces-a-record-wave-of-retirements-as-
it-grapples-with-historic-labour-shortages.
Helmer, O., cited in Bramwell, L., and E. Hykawy. 1999. “The Delphi Technique: A Possible Tool for Predicting Future Events in Nursing Education.”
Canadian Journal of Nursing Research
30 (4): 47–58.
Kang, I. G., B. Croft, and B. A. Bichelmeyer. 2020. "Predictors of Turnover Intention in U.S. Federal Government Workforce: Machine Learning Evidence that Perceived Comprehensive HR Practices Predict Turnover Intention."
Public Personnel Management
50 (4): 538–558. https://doi.org/10.1177/0091026020977562.
Korte, R. F., and T. J. Chermack. 2006. “Changing Organizational Culture with Scenario Planning.”
Futures
39 (6) : 645–656. https://doi.org/10.1016/j.futures.2006.11.001.
Langford, B. E., G. Schoenfeld, and G. Izzo. 2002. “Nominal Grouping Sessions vs. Focus Groups."
Qualitative Market Research
5 (1): 58–70. https://doi.org/10.1108/13522750210414517.
Loo, R., and K. Thorpe. 2003. “A Delphi Study Forecasting Management Training and Development for First-Line Nurse Managers.”
The Journal of Management Development
22 (9/10): 824–825. https://doi.org/10.1108/02621710310495801.
Your preview ends here
Eager to read complete document? Join bartleby learn and gain access to the full version
- Access to all documents
- Unlimited textbook solutions
- 24/7 expert homework help
Loo, R., and K. Thorpe. 2004. “Making Female First-Line Nurse Managers More Effective: A
Delphi Study of Occupational Stress.”
Women in Management Review
19 (2): 88–97. https://doi.org/10.1108/09649420410525298.
Lundy, M. 2021. “The ‘Great Resignation’? It’s Not Happening in Canada.” Eastern Workforce Innovation Board. Accessed August 19, 2022. https://www.workforcedev.ca/the-great-resignation-its-not-happening-in-canada/.
Luthans, F. 1992.
Organizational Behavior
, 6th ed. New York, NY: McGraw-Hill.
Magnusson, K. 2022. “Interpreting Correlations: An interactive visualization” Rpsychologist.com. June 9, 2020. Accessed September 1, 2022. https://rpsychologist.com/correlation/.
Martel, L. 2019. “The Labour Force in Canada and Its Regions: Projections to 2036.” Statistics Canada. Accessed August 19, 2022. https://www150.statcan.gc.ca/n1/pub/75-
006-x/2019001/article/00004-eng.htm.
McBeath, G. 1992.
The Handbook of Human Resource Planning: Practical Manpower Analysis Techniques for HR Professionals
. Oxford: Blackwell.
Meehan, R., and B.S. Ahmed. 1990. “Forecasting Human Resources Requirements: A Demand Model.”
Human Resource Planning
13 (4): 297–307.
Milkovich, G. T, A. Annoni, and T. Mahoney. 1972. “The Use of Delphi Procedures in Manpower Forecasting.”
Management Science
19 (4): 381–388. https://doi.org/10.1287/mnsc.19.4.381.
Milkovich, G.T., and T. A. Mahoney. 1978. “Human Resource Planning Models: A Perspective.”
Human Resource Planning
1 (1): 19–30.
Moore, T., and E. Bokelberg. 2019. "How IBM Incorporates Artificial Intelligence into Strategic Workforce Planning."
People & Strategy
42
(4), 52–55.
Navarro D. J., and D. R. Foxcroft. 2022. "Learning Statistics with Jamovi: A Tutorial for Psychology Students and Other Beginners. (Version 0.75)." DOI: 10.24384/hgc3-7p15. http://learnstatswithjamovi.com.
Oswald, F. L., T. S. Behrend, D. J. Putka, and E. Sinar. 2020. "Big Data in Industrial-
Organizational Psychology and Human Resource Management: Forward Progress for Organizational Research and Practice."
Annual Review of Organizational Psychology and Organizational Behavior
7
(1): 505–533. https://doi.org/10.1146/annurev-orgpsych-
032117-104553.
Your preview ends here
Eager to read complete document? Join bartleby learn and gain access to the full version
- Access to all documents
- Unlimited textbook solutions
- 24/7 expert homework help
Fusfeld, A. R., and R. N. Foster. 1971. “The Delphi Technique: Survey and Comment.”
Business Horizons
14 (3): 63–74. https://doi.org/10.1016/0007-
6813(71)90120-0.
Passmore, D., E. Cebeci, and R. Baker. 2005. “Market-based Information for Decision Support in Human Resource Development.”
Human Resource Development Review
4 (1): 33–49. https://doi.org/10.1177/1534484304273843.
Roeden, J. M., M. A. Maaskant, and L.M. Curfs. 2012. “The Nominal Group Technique as an Evaluation Tool for Solution-focused Coaching.”
Journal of Applied Research in Intellectual Disabilities
25 (6): 588–593. https://doi.org/10.1111/j.1468-
3148.2012.00696.x.
Rohrbaugh, J. 1981. “Improving the Quality of Group Judgement: Social Judgement Analysis and the Nominal Group Technique.”
Organizational Behavior and Human Performance
28 (2): 272–288. https://doi.org/10.1016/0030-5073(81)90025-8.
Sobaih, A.E., C. Ritchie, and E. Jones. 2012. “Consulting the Oracle? Applications of Modified Delphi Technique to Qualitative Research in the Hospitality Industry.”
International Journal of Contemporary Hospitality Management
24 (6): 886–
906. https://doi.org/10.1108/09596111211247227.
Statistics Canada. 2022. “The Daily — Labour Force Survey, December 2021.” Accessed August 19, 2022. https://www150.statcan.gc.ca/n1/daily-quotidien/220107/dq220107a-
eng.htm.
Surowiecki, J. 2005.
The Wisdom of Crowds
. Toronto: Random House.
Tetlock, P. 2005.
Expert Political Judgment: How Good Is It? How Can We Know?
Princeton, NJ: Princeton University Press.
Thomas, D. A., and R. J. Ely. 1996. "Making Differences Matter: A New Paradigm for Managing Diversity."
Harvard Business Review
74 (5): 79–90.
U.S. Bureau of Labor Statistics. 2022. “Number of Quits at All-Time High in November 2021:
The Economics Daily: U.S. Bureau of Labor Statistics.” Accessed August 19, 2022. https://www.bls.gov/opub/ted/2022/number-of-quits-at-all-time-high-in-november-
2021.htm.
Van de Ven, A. H. 1974.
Group Decision-making Effectiveness
. Kent, OH: Kent State University Center for Business and Economic Research Press.
Ward, D. 1996. “Workforce Demand Forecasting Techniques.”
Human Resource -
Planning
19 (1): 54–55.
Your preview ends here
Eager to read complete document? Join bartleby learn and gain access to the full version
- Access to all documents
- Unlimited textbook solutions
- 24/7 expert homework help
Credits
[1] Image by fizkes/Shutterstock.com
[2] Video courtesy of Global News/YouTube
[3] Image by haryanta.p/Shutterstock.com
[4] Image by Pink Candy/Shutterstock.com
[5] Magnusson, K. 2022. “Interpreting Correlations: An interactive visualization” Rpsychologist.com. June 9, 2020. Accessed September 1, 2022. https://rpsychologist.com/correlation/.
[6] Video courtesy of freeCodeCamp.org/YouTube
[7]
Image
by Scott Platt
CC0
[8] Image by Pakpoom Makpan/Shutterstock.com
[9]
Image
by Possessed Photography on
Unsplash
[10]
Image
by Cedric Fauntleroy
CC0
[11] © iStock/Thinkstock.com
[12] Image by wutzkohphoto/Shutterstock.com
Your preview ends here
Eager to read complete document? Join bartleby learn and gain access to the full version
- Access to all documents
- Unlimited textbook solutions
- 24/7 expert homework help
Related Documents
Recommended textbooks for you
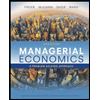
Managerial Economics: A Problem Solving Approach
Economics
ISBN:9781337106665
Author:Luke M. Froeb, Brian T. McCann, Michael R. Ward, Mike Shor
Publisher:Cengage Learning
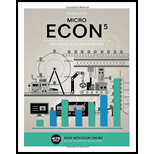
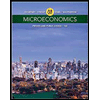
Microeconomics: Private and Public Choice (MindTa...
Economics
ISBN:9781305506893
Author:James D. Gwartney, Richard L. Stroup, Russell S. Sobel, David A. Macpherson
Publisher:Cengage Learning
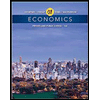
Economics: Private and Public Choice (MindTap Cou...
Economics
ISBN:9781305506725
Author:James D. Gwartney, Richard L. Stroup, Russell S. Sobel, David A. Macpherson
Publisher:Cengage Learning
Recommended textbooks for you
- Managerial Economics: A Problem Solving ApproachEconomicsISBN:9781337106665Author:Luke M. Froeb, Brian T. McCann, Michael R. Ward, Mike ShorPublisher:Cengage Learning
- Microeconomics: Private and Public Choice (MindTa...EconomicsISBN:9781305506893Author:James D. Gwartney, Richard L. Stroup, Russell S. Sobel, David A. MacphersonPublisher:Cengage LearningEconomics: Private and Public Choice (MindTap Cou...EconomicsISBN:9781305506725Author:James D. Gwartney, Richard L. Stroup, Russell S. Sobel, David A. MacphersonPublisher:Cengage Learning
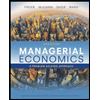
Managerial Economics: A Problem Solving Approach
Economics
ISBN:9781337106665
Author:Luke M. Froeb, Brian T. McCann, Michael R. Ward, Mike Shor
Publisher:Cengage Learning
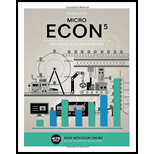
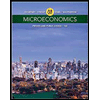
Microeconomics: Private and Public Choice (MindTa...
Economics
ISBN:9781305506893
Author:James D. Gwartney, Richard L. Stroup, Russell S. Sobel, David A. Macpherson
Publisher:Cengage Learning
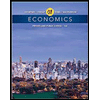
Economics: Private and Public Choice (MindTap Cou...
Economics
ISBN:9781305506725
Author:James D. Gwartney, Richard L. Stroup, Russell S. Sobel, David A. Macpherson
Publisher:Cengage Learning