Tutorial Wk 8 Simple Linear Reg
pdf
keyboard_arrow_up
School
Royal Melbourne Institute of Technology *
*We aren’t endorsed by this school
Course
1607
Subject
Economics
Date
May 29, 2024
Type
Pages
12
Uploaded by BarristerGalaxy7447
1 File: Tutorial Wk 8 Simple Linear Reg ECON1607 Tutorial Simple Linear Regression Questions for in-class tutorial: The questions covered in the tutorial relate to the material covered in Chapter 12 of Berenson et al. Problems from Berenson et al. 12.3 P420 Different #s in 2010 12.11 P427 [2 Problems involving interpreting Excel output] 1. It seems logical that the more bank accounts there are, the more Automated Teller Machine (ATM) withdrawals there will be. The Reserve Bank of Australia (RBA) has performed a simple regression analysis to predict the number of ATM withdrawals by the number of bank accounts. The Excel output is given below. SUMMARY OUTPUT Regression Statistics Multiple R 0.504 R Square 0.254 Adjusted R Square 0.179 Standard Error 2889.685 Observations 12 ANOVA df SS MS F Significance F Regression 1 28403158.08 28403158.08 3.401461041 0.094926942 Residual 10 83502817.59 8350281.759 Total 11 111905975.7 Coefficients Standard Error t Stat P-value Lower 95% Upper 95% Intercept b
0
-47109.624 63582.514 -0.741 0.476 -188780.292 94561.045 Number of accounts ('000) b
1
4.357 2.362 1.844 0.095 -0.907 9.620 a. Write down the estimated regression equation. b. Interpret the slope and intercept coefficients. c. What is the value of r
2
? Interpret the result. What is the value of adjusted r
2
? Interpret the result. d. Is “Number of accounts” a significant predictor (use the 0.05 significance level)?
e. Predict the number of ATM withdrawals if the number of accounts is 27,700,000.
2 File: Tutorial Wk 8 Simple Linear Reg [Problem involving interpreting Excel output] What are we trying to predict? y = ATM Withdrawals What are we using to predict y? x = number of accounts 1a)
Write down the estimated regression equation.
P414 Slide 10
= - 47109.624 + 4.357 x number of accounts (‘000) 1b
) Interpret the slope and intercept coefficients. - The intercept term is not meaningful here. When the number of accounts is zero the number of ATM withdrawals is -47,109.624.
Obviously, this is not possible. The intercept shows things other than x that affect y. Also, the y intercept is not in the range of observable values so we so not focus on it. S 18 Interpolate not extrapolate - In statistics we are more interested in the slope than the intercept. For the variable number of accounts, the slope means that when the number of accounts is increased by 1(000), the number of ATM withdrawals increases by 4.357
(slope). 1c) What is the value of r
2
? Interpret the result. On Excel output R Square = r
2 r
2
= 0.254. It means that 25.4% of the variation in the number of ATM withdrawals can be explained by variability in the number of bank accounts. In Finance 25% is considered to be a good r
2
. r
2
is the coefficient of determination p 424 and measures the proportion of the variation in Y that is explained by X r
2
= Amount of y explained by x
. r
2
is the portion of the total variation in the dependent variable y that is explained by variation in the independent variable x. Slide 21. How well can x explain y? It is between zero and one, the closer to one, the better the model at prediction. This is related to the correlation coefficient concept. What is the value of adjusted r
2
? Interpret the result. r
2
adj = 0.179 17.9% of the variation in the number of ATM withdrawals can be explained by variability in the number of bank accounts taking into account the number of independent variables and sample size. 1
d) Is “Number of accounts” a significant predictor (use the 0.05 significance level)? ALTERNATIVE METHOD If the p value < α then reject H
0
. p value is instead of doing the hypothesis test α = 0.05 so if p value < 0.05 then reject H
0 Find and add Reject areas on Z table The p value = 0.095 on the excel output so 0.095 > 0.05
then do not
reject H
0
. There is insufficient evidence
to conclude that there is a linear relationship between ATM 0
1
ˆ
i
i
Y
b
b X
=
+
ˆ
y
3 File: Tutorial Wk 8 Simple Linear Reg withdrawals and number of accounts . So number of accounts is not a significant predictor at the .05 level of significance. HASCCC = Hypothesis, Alpha, Statistical test, Critical values, Calculate, Conclusion Hypothesis testing is to see if Beta
1
does not equal 0. If the slope equals 0 it means that there is no relationship between x and y
. If we reject the null hypothesis we conclude there is evidence of a linear relationship. P437 H
0
: ᵦ
1
= 0 H
1
:
ᵦ
1
≠ 0 Level of significance alpha = 0.05 α/2 = 0.025 We always use t test when we test for slope and note that we use n-2 for df Critical values: t
crit 10, 0.025
= ± 2.2281 Use n - 2 = 12 – 2 = 10 df and 0.025 column Decision rule: reject H
0
if t
calc
> 2.2281 or t
calc
< - 2.2281 Testing a hypothesis for a population slope using the t
-test: Slide 45 P438
b
1
is the slope, S
b1
is the standard error t
calc
= ( 4.357 – 0) / 2.362 = 1.844 = t
calc
This is given in the t stat column
. You can find all of this on the Excel output. Since 1.844 < 2.2281 do not reject H
0
. There is insufficient evidence to conclude that there is a linear relationship between ATM withdrawals and number of accounts . So number of accounts is not a significant predictor at the .05 level of significance. Do Not Reject Reject _______________________________ t -2.2281 1.844 2.2281 t calc tcrit 1d) What is the standard error of the estimate, s
yx
? What does this value tell us? The standard error of the estimate, s
yx
= 2889.685. It measures variation about the prediction line and is measured in the same units as the dependent (outcome) variable y which is the number of ATM withdrawals. P427 Slide 25 By itself s
yx
is meaningless. It must be compared to Y values. 0
b
1
1
1
b
b
t
S
b
-
=
Your preview ends here
Eager to read complete document? Join bartleby learn and gain access to the full version
- Access to all documents
- Unlimited textbook solutions
- 24/7 expert homework help
4 File: Tutorial Wk 8 Simple Linear Reg This is another measure of how good the model is. The smaller it is in comparison to the Y values the better. In this case we don’t show Y values so there is nothing to compare it to. 1e)
Predict the number of ATM withdrawals if the number of accounts is 27,700,000.
= -47,109.624 + 4.357*27,700 = 73,579.28 Remember x is in (000) 2) Port Kembla Golf Club
wishes to predict the number of golfers per weekend based upon weather conditions. The number of golfers and temperature is measured over a sample of weekends covering all seasons. SUMMARY OUTPUT Regression Statistics Multiple R 0.380016868 R Square 0.14441282 Adjusted R Square 0.078598421 Standard Error 20.33164649 Observations 15 ANOVA df SS MS F Significance F Regression 1 907.0472938 907.0472938 2.194243557 0.162354813 Residual 13 5373.88604 413.3758492 Total 14 6280.933333 Coefficients Standard Error t Stat P-value Lower 95% Upper 95% Intercept 64.18876657 19.45167504 3.299909465 0.005748721 22.16597751 106.2115556 Temperature 1.304603453 0.880716449 1.48129793 0.162354813 -0.598068759 3.207275664 a. State the estimated simple linear regression equation. b. Interpret the meaning of the intercept term, b
0,
, and the slope, b
1
, in this problem. c. Construct a 95% confidence interval estimate of the population slope, . d. At the 0.05 level of significance, determine whether there is a significant relationship between the number of golfers and weather conditions. Use both, critical and p-value approach. e. Predict the mean number of golfers if the temperature is 25 degrees Celsius. f. Interpret the coefficient of determination, r
2
. What are we trying to predict? y = number of golfers What are we using to predict y? x = weather conditions or temperature a. State the estimated simple linear regression equation P414 Slide 10
ˆ
y
0
1
ˆ
i
i
Y
b
b X
=
+
1
b
0
1
ˆ
i
i
Y
b
b X
=
+
5 File: Tutorial Wk 8 Simple Linear Reg = 64.189 + 1.304 x Temperature b. Interpret the meaning of the intercept term b
0
, and the slope b
1
, in this problem. The intercept term = 64.189 which means when the X variable of temperature = 0 the y variable, number of golfers will be 64.189. For the slope, it means that when the temperature is increased by 1, the number of golfers increases by 1.304. c. Construct a 95% confidence interval estimate of the population slope, . Confidence interval estimate of the slope : Slide 51 P440 1.304 ± (2.1604 x 0.8807) = -0.598 ≤ ᵦ
1
≤ 3.207 This is on the Excel output
d. At the 0.05 level of significance, determine whether there is a significant relationship between the number of golfers and weather conditions. Use both, critical and p-value approach. HASCCC = Hypothesis, Alpha, Statistical test, Critical values, Calculate, Conclusion Hypothesis testing is to see if Beta
1
does not equal 0. If the slope equals 0 it means that there is no relationship between x and y
. If we reject the null hypothesis we conclude there is evidence of a linear relationship. P437 H
0
: ᵦ
1
= 0 H
1
:
ᵦ
1
≠ 0 Level of significance alpha = 0.05 α/2 = 0.025 We always use t test when we test for slope and note that we use n-2 for df Critical values: t
crit 13, 0.025
= ± 2.1604 Use n - 2 = 15 – 2 = 13 df and 0.025 column Decision rule: reject H
0
if t
calc
> 2.1604 or t
calc
< - 2.1604 Testing a hypothesis for a population slope using the t
-test: Slide 45 P438
b
1
is the slope, S
b1
is the standard error t
calc
= ( 1.305 – 0) / 0.881 = 1.481 = t
calc
This is given in the t stat column . You can find all of this on the Excel output. Since 1.48 < 2.1604 do not reject H
0
. There is insufficient evidence
to conclude
that there is a linear relationship
between golfers and temperature
. Do Not Reject Reject ˆ
y
1
b
1
b
1
2
1
n
b
b
t
S
-
±
0
b
1
1
1
b
b
t
S
b
-
=
6 File: Tutorial Wk 8 Simple Linear Reg ________________________________ t -2.1604 1.481 2.1604 tcalc tcrit If the p value < α then reject H
0
. p value is instead of doing the hypothesis test α = 0.05 so if p value < 0.05 then reject H
0 Find and add Reject areas on Z table The p value = 0.1623 on the excel output so 0.16 > 0.05
then do not reject H
0
. This is the same answer as above. e. Predict the mean number of golfers if the temperature is 25 degrees Celsius. = 64.189 + 1.304 x 25 = 96.789 = 97 golfers f. Interpret the coefficient of determination, r
2
. r
2
= 0.1444. It means that 14.4% of the variation in golfers can be explained by variability in the temperature. r
2
= 907.0472/6280.933 = 0.1444 This is on the Excel output.
r
2
= SSR
/
SST Slide 21 SST = SSR + SSE Slide 19 r
2
is the coefficient of determination p 424 and measures the proportion of the variation in Y that is explained by X. r
2
= Amount of y explained by x. r
2
is the portion of the total variation in the dependent variable y that is explained by variation in the independent variable x. Slide 21. How well can x explain y? It is between zero and one, the closer to one, the better the model at prediction. This is related to the correlation coefficient concept. 2. A transport consultant wishes to explore the relationship between air travel and ticket prices. She collects data over a 12-month period and estimated the following output: 0
1
ˆ
i
i
Y
b
b X
=
+
Your preview ends here
Eager to read complete document? Join bartleby learn and gain access to the full version
- Access to all documents
- Unlimited textbook solutions
- 24/7 expert homework help
7 File: Tutorial Wk 8 Simple Linear Reg a) Interpret the meaning of the slope. b) Predict the number of air passengers if the average ticket price is $110. c) Interpret the adj. r
2
value. d) At the 0.05 level of significance, is there evidence of a linear relationship between passenger numbers and ticket price? e) What other independent variables might you consider for inclusion in the model? Write down the estimated regression equation.
P414 Slide 10
= 2070.61 – 11.83 x X Variable 1 (ticket prices) a) Interpret the meaning of the slope. - For X Variable 1 (ticket prices), it means that when X is increased by 1, the amount (number) of air travel passengers decreases by 11.83 (slope). b) Predict the number of air passengers if the average ticket price is $110. = 2070.61 – 11.83 x X Variable 1 (ticket prices) = 2070.61 – (11.83 x $110) = 2070.61 – 1301.30 = 769.31 passengers c) Interpret the adj. r
2
value
. r
2
adj = 0.87 87.0% of the variation in the number of air passengers can be explained by ticket prices taking into account the number of independent variables and sample size. SUMMARY OUTPUT
Regression Statistics
Multiple R
0.94
R Square
0.88
Adjusted R Square
0.87
Standard Error
57.57
Observations
12
ANOVA
df
SS
MS
F
Significance F
Regression
1
247024.6
247024.6
74.53907
6.0035E-06
Residual
10
33140.29
3314.0
Total
11
280164.9
Coefficients
andard Erro
t Stat
P-value
Lower 95%
Upper 95%
Intercept
2070.61
150.40
13.77
0.00
1735.50
2405.72
X Variable 1
-11.83
1.37
-8.63
0.00
-14.88
-8.78
0
1
ˆ
i
i
Y
b
b X
=
+
ˆ
y
ˆ
y
ˆ
y
8 File: Tutorial Wk 8 Simple Linear Reg d) At the 0.05 level of significance, is there evidence of a linear relationship between passenger numbers and ticket price? HASCCC = Hypothesis, Alpha, Statistical test, Critical values, Calculate, Conclusion Hypothesis testing is to see if Beta
1
does not equal 0. If the slope equals 0 it means that there is no relationship between x and y
. If we reject the null hypothesis we conclude there is evidence of a linear relationship. P437 H
0
: ᵦ
1
= 0 H
1
:
ᵦ
1
≠ 0 Level of significance alpha = 0.05 α/2 = 0.025 We always use t test when we test for slope and note that we use n-2 for df Critical values: t
crit 10, 0.025
= ± 2.2281 Use n - 2 = 12 – 2 = 10 df and 0.025 column Decision rule: reject H
0
if t
calc
> 2.2281 or t
calc
< - 2.2281 Testing a hypothesis for a population slope using the t
-test: P438
b
1
is the slope, S
b1
is the standard error t
calc
= ( -11.83 – 0) / 1.37 = -8.63 = t
calc
This is given in the t stat column . You can find all of this on the Excel output. Since -8.63 < -2.2281 reject H
0
. There is sufficient evidence to conclude that there is a linear relationship between passenger numbers and ticket price
. Do Not Reject Reject ________________________________ t - 8.63 -2.2281 2.2281 ALTERNATIVE METHOD If the p value < α then reject H
0
. p value is instead of doing the hypothesis test α = 0.05 so if p value < 0.05 then reject H
0 Find and add Reject areas on Z table The p value = 0 on the excel output so 0 < 0.05 then reject H
0
. There is sufficient evidence to conclude that there is a linear relationship between passenger numbers and ticket price.
0
b
1
1
1
b
b
t
S
b
-
=
9 File: Tutorial Wk 8 Simple Linear Reg e) What other independent variables might you consider for inclusion in the model? THIS COULD BE ANYTHING. WHICH AIRLINE WITH THE BEST REPUTATION OR ADVT, MOST # OF FLIGHTS PER DAY, CUSTOMER SERVICE, LEG ROOM. 2. It is widely believed in the real estate trade that house rental prices are inversely related to vacancy rates. Rusty Real Estate has collated rental prices and vacancy rates for a number of Sydney suburbs and performed a simple regression analysis to predict the relationship between rent and vacancy rates. The Excel output is given below. SUMMARY OUTPUT Regression Statistics Multiple R 0.970866825 R Square ………. Adjusted R Square 0.936202657 Standard Error ………… Observations 11 ANOVA df SS MS F Significance F Regression SSR 1 255504.1 255504.095 147.7463 6.9E-07 Residual SSE 9 15564.087 1729.34297 Total SST 10 -------- Coefficients Standard Error t Stat P-value Lower 95% Upper 95% Intercept 1045.387 47.561 21.980 0.000 937.796 1152.978 vacancy rate(%) -65.960 5.427 -12.155 0.000 -78.235 -53.684 a. State the estimated linear regression equation. b. Interpret the meaning of the slope and intercept coefficients. c. Calculate the coefficient of determination, r
2
, and interpret its meaning. d. What is the standard error of the estimate, s
yx
? e. Predict the house rental price of the suburb, Bondi, which has a vacancy rate of 9%. What are we trying to predict? y = rental prices What are we using to predict y? x = vacancy rates 1a)
State the estimated linear regression equation.
Your preview ends here
Eager to read complete document? Join bartleby learn and gain access to the full version
- Access to all documents
- Unlimited textbook solutions
- 24/7 expert homework help
10 File: Tutorial Wk 8 Simple Linear Reg Rental Prices =
= 1045.387 – 65.960 x vacancy rate (%) P414 Slide 10
1b
) Interpret the meaning of the slope and intercept coefficients.
The intercept term = 1045 which means when the X variable of vacancy rate = 0 the rent will be 1045.387. For the vacancy rate, it means that when the vacancy rate is increased by 1(%), the rent decreases by 65.960. 1c)
Calculate the coefficient of determination, r
2
, and interpret its meaning. r
2
= 0.94. It means that 94% of the variation in rent can be explained by variability in the vacancy rate. r
2
= 255,504.1/(255,504.1 + 15564.087) = 0.9423
r
2
= SSR
/
SST Slide 21 SST = SSR + SSE Slide 19 r
2
is the coefficient of determination p 424 and measures the proportion of the variation in Y that is explained by X. r
2
= Amount of y explained by x. r
2
is the portion of the total variation in the dependent variable y that is explained by variation in the independent variable x. Slide 21. How well can x explain y? It is between zero and one, the closer to one, the better the model at prediction. This is related to the correlation coefficient concept. 1d) What is the standard error of the estimate, s
yx
?
Standard error of the estimate: S
YX
= √ 15,564.087/ (11-2) = √1729.43 = 41.586 = S
YX
1e)
Predict the house rental price of the suburb, Bondi, which has a vacancy rate of 9%. = 1045.387 – 65.960 x 9 = 1045.387 – 593.64 = 451.74 House rental price
2013 EDITION 12.3 Fitting a straight line to a set of data yields the following prediction line: Yhat = 24 – 2.5 X (a) Interpret the meaning of the Y intercept, b
0
. (b) Interpret the meaning of the slope b
1
. (c) Predict the mean value of Y for X = 6. (a) Interpret the meaning of the Y intercept, b
0
. ˆ
y
0
1
ˆ
i
i
Y
b
b X
=
+
2
1
ˆ
(
)
2
2
n
i
i
i
YX
Y
Y
SSE
S
n
n
=
-
=
=
-
-
å
0
1
ˆ
i
i
Y
b
b X
=
+
ˆ
y
ˆ
y
ˆ
y
11 File: Tutorial Wk 8 Simple Linear Reg When X = 0, the estimated expected value of Y is 24. (b) Interpret the meaning of the slope b
1
. For each increase in the value X by 1 unit, you can expect a decrease of an estimated 2.5 units in the value of Y
. (c) Predict the mean value of Y for X = 6. ˆ
Y = 24 – 2.5X
= 24 – 2.5(6) = 9 2010 EDITION 12.3 Yhat = 16 – 0.5X (a) When X = 0, the estimated expected value of Y is 16. (b) For each increase in the value X by 1 unit, you can expect a decrease in an estimated 0.5 units in the value of Y
. DIFFERENT #S (c) ˆ
Y = 16 – 0.5
X = 16 – 0.5(6) = 13 12.11 P427) If SSR = 57 and SSE = 12 find SST and then calculate the coefficient of determination r
2
. SST = SSR + SSE Slide 19 57 + 12 = 69 = SST R
2
= SSR
/
SST Slide 21 Page 424 = 57/69 = 0.8261= r
2 82.61% of the variation in Y is explained by variation in X
. Chapter 12 Simple Linear Regression Topic 9 Estimation and prediction is the goal of statistics. Last week we talked about the hypothesis test which is an assumption about the population parameters or proportions. Linear Regression is the last part of the course. It is not related to the normal distribution. Simple linear regression is the best tool for prediction. It is called simple because we use only one variable. Predict a specific variable using another variable. Variable 1 is y: predict a student’s Mark on an exam Variable 2 is x: # of Hours of study preparation. Use this to predict the exam Mark predicts
12 File: Tutorial Wk 8 Simple Linear Reg (X) Hour s Mark (Y) Independent Variable Dependent Variable Use X to predict Y Linear relationship (a straight line) between 2 variables. Slide 9 Want to draw a line that goes through the data in the closest possible way to all points of data on graph. The difference between the actual observation and the predicted observation is the error. This is similar to the standard deviation. The smaller the standard error to the y value the better the model. Yhat indicates a predicted value Slide 10
Yhat = y intercept + slope * independent variable X The y intercept or b
0
is the starting point on the y axis. Y intercept = value of y when x = 0 Slide 16 Slope b
1
= change in value of y when x increases by one unit Slope is how y changes in response to changes in X All you need to know is how to interpret b
0
and b
1
In our example for predicting a student’s Mark on an exam b
0
= 60 b
1
= 5 Mark Yhat = Mark = b
0
+ b
1
x Hours 90 = 60 + (5x 3) = 75 80 . 70 60 Data 50 1 2 3 4 5 6 7 Hours 0
1
ˆ
i
i
Y
b
b X
=
+
Your preview ends here
Eager to read complete document? Join bartleby learn and gain access to the full version
- Access to all documents
- Unlimited textbook solutions
- 24/7 expert homework help
Related Documents
Recommended textbooks for you
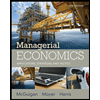
Managerial Economics: Applications, Strategies an...
Economics
ISBN:9781305506381
Author:James R. McGuigan, R. Charles Moyer, Frederick H.deB. Harris
Publisher:Cengage Learning
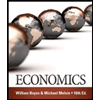
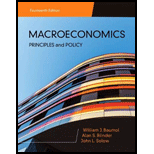
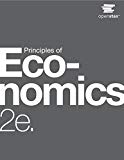
Principles of Economics 2e
Economics
ISBN:9781947172364
Author:Steven A. Greenlaw; David Shapiro
Publisher:OpenStax
Recommended textbooks for you
- Managerial Economics: Applications, Strategies an...EconomicsISBN:9781305506381Author:James R. McGuigan, R. Charles Moyer, Frederick H.deB. HarrisPublisher:Cengage Learning
- Principles of Economics 2eEconomicsISBN:9781947172364Author:Steven A. Greenlaw; David ShapiroPublisher:OpenStax
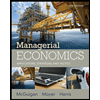
Managerial Economics: Applications, Strategies an...
Economics
ISBN:9781305506381
Author:James R. McGuigan, R. Charles Moyer, Frederick H.deB. Harris
Publisher:Cengage Learning
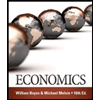
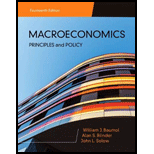
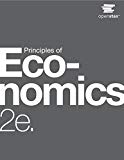
Principles of Economics 2e
Economics
ISBN:9781947172364
Author:Steven A. Greenlaw; David Shapiro
Publisher:OpenStax