Data Feminism Activity
docx
keyboard_arrow_up
School
University of Colorado, Boulder *
*We aren’t endorsed by this school
Course
1101
Subject
Computer Science
Date
Feb 20, 2024
Type
docx
Pages
2
Uploaded by maddie6638
Your preview ends here
Eager to read complete document? Join bartleby learn and gain access to the full version
- Access to all documents
- Unlimited textbook solutions
- 24/7 expert homework help
Recommended textbooks for you
Np Ms Office 365/Excel 2016 I Ntermed
Computer Science
ISBN:9781337508841
Author:Carey
Publisher:Cengage
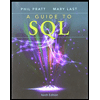
A Guide to SQL
Computer Science
ISBN:9781111527273
Author:Philip J. Pratt
Publisher:Course Technology Ptr
COMPREHENSIVE MICROSOFT OFFICE 365 EXCE
Computer Science
ISBN:9780357392676
Author:FREUND, Steven
Publisher:CENGAGE L
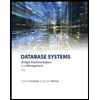
Database Systems: Design, Implementation, & Manag...
Computer Science
ISBN:9781305627482
Author:Carlos Coronel, Steven Morris
Publisher:Cengage Learning
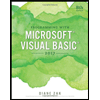
Programming with Microsoft Visual Basic 2017
Computer Science
ISBN:9781337102124
Author:Diane Zak
Publisher:Cengage Learning
Recommended textbooks for you
- Np Ms Office 365/Excel 2016 I NtermedComputer ScienceISBN:9781337508841Author:CareyPublisher:CengageA Guide to SQLComputer ScienceISBN:9781111527273Author:Philip J. PrattPublisher:Course Technology PtrCOMPREHENSIVE MICROSOFT OFFICE 365 EXCEComputer ScienceISBN:9780357392676Author:FREUND, StevenPublisher:CENGAGE L
- Database Systems: Design, Implementation, & Manag...Computer ScienceISBN:9781305627482Author:Carlos Coronel, Steven MorrisPublisher:Cengage LearningProgramming with Microsoft Visual Basic 2017Computer ScienceISBN:9781337102124Author:Diane ZakPublisher:Cengage Learning
Np Ms Office 365/Excel 2016 I Ntermed
Computer Science
ISBN:9781337508841
Author:Carey
Publisher:Cengage
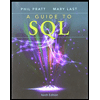
A Guide to SQL
Computer Science
ISBN:9781111527273
Author:Philip J. Pratt
Publisher:Course Technology Ptr
COMPREHENSIVE MICROSOFT OFFICE 365 EXCE
Computer Science
ISBN:9780357392676
Author:FREUND, Steven
Publisher:CENGAGE L
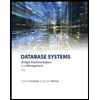
Database Systems: Design, Implementation, & Manag...
Computer Science
ISBN:9781305627482
Author:Carlos Coronel, Steven Morris
Publisher:Cengage Learning
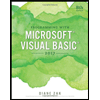
Programming with Microsoft Visual Basic 2017
Computer Science
ISBN:9781337102124
Author:Diane Zak
Publisher:Cengage Learning