RM2_Practice_Question_Set2_solution
pdf
keyboard_arrow_up
School
Clyde Savannah High School *
*We aren’t endorsed by this school
Course
5385
Subject
Accounting
Date
Nov 24, 2024
Type
Pages
20
Uploaded by 1250A
STAT3058/6058 Practice Questions Solutions
Topic 2 - Aggregate Claims Modelling
1. Evaluate the MGF of the aggregate claim amount at
t
= 0
.
01
, if the number of claims is binomial with
parameters
n
= 100
and
p
= 0
.
01
, and individual claims sizes are Gamma distributed with parameters
α
= 10
and
θ
= 5
.
Solution:
Since
N
∼
Bin(100
,
0
.
01)
and
X
∼
Gamma(10
,
5)
, we have:
M
N
(
t
) =
(
0
.
99 + 0
.
01
e
t
)
100
and
M
X
(
t
) = (1
−
5
t
)
−
10
So:
M
S
(
t
) =
M
N
[ln
M
X
(
t
)] =
h
0
.
99 + 0
.
01
e
ln
M
X
(
t
)
i
100
= [0
.
99 + 0
.
01
M
X
(
t
)]
100
=
0
.
99 + 0
.
01(1
−
5
t
)
−
10
100
and:
M
S
(0
.
01) =
0
.
99 + 0
.
01(1
−
5(0
.
01))
−
10
100
= 1
.
95
2. The random variable
S
has a compound Poisson distribution with Poisson parameter 4 . The individual claim
amounts are either 1 , with probability 0.3, or 3 , with probability 0.7. Calculate the probability that
S
= 4
.
Solution:
We can get an aggregate claim amount of 4 in only two ways: a) 2 claims, with one claim of amount 1
and one claim of amount 3 , or b) 4 claims, with each of amount 1 .
The probability of this happening is:
P
(
S
= 4) =
P
(
N
= 2)
P
(
X
1
= 1)
P
(
X
2
= 3) +
P
(
N
= 2)
P
(
X
1
= 3)
P
(
X
2
= 1)
+
P
(
N
= 4)
P
(
X
1
= 1)
P
(
X
2
= 1)
P
(
X
3
= 1)
P
(
X
4
= 1)
The
X
’s are identical, so this simplifies to:
P
(
S
= 4) = 2
P
(
N
= 2)
P
(
X
= 1)
P
(
X
= 3) +
P
(
N
= 4)
P
(
X
= 1)
4
= 2
×
e
−
4
4
2
2!
×
0
.
3
×
0
.
7 +
e
−
4
4
4
4!
×
0
.
3
4
= 0
.
06312
1
3. Let
S
1
and
S
2
be independent random variables having compound Poisson distributions with parameters
λ
1
= 3
and
F
1
(
x
)
and
λ
2
= 1
and
F
2
(
x
)
, respectively. Suppose that the support of both
F
1
(
x
)
and
F
2
(
x
)
is
the set
{
1
,
2
,
3
,
4
,
5
}
and the CDFs are given in the following table:
x
1
2
3
4
5
F
1
(
x
)
0
.
1
0
.
3
0
.
3
0
.
7
1
.
0
F
2
(
x
)
0
.
2
0
.
4
0
.
6
0
.
8
1
.
0
Note the support of a random variable, and therefore its CDF, is the set of its possible outcomes.
(a) What is the distribution of
S
=
S
1
+
S
2
? Find
E
[
S
]
,
V
[
S
]
and the mgf of
S
.
(b) Find
P
(
S
≤
3)
.
Solution:
(a) From course notes, we know that
S
has a compound Poisson distribution with parameters
Λ =
λ
1
+
λ
2
= 4
and
F
(
x
) =
3
4
F
1
(
x
) +
1
4
F
2
(
x
)
, so that
F
(
x
)
is given by the table:
x
1
2
3
4
5
F
(
x
)
0
.
125
0
.
325
0
.
375
0
.
725
1
.
0
From this information, we can calculate the
pmf
of a random variable with a
CDF
of
F
(
x
)
as
p
(
x
) =
F
(
x
)
−
F
(
x
−
1)
:
x
1
2
3
4
5
p
(
x
)
0
.
125
0
.
2
0
.
05
0
.
35
0
.
275
So, the mean and raw second moment of a random variable
X
with
pmf
p
(
x
)
are:
µ
1
=
E
[
X
] = 1(0
.
125) + 2(0
.
2) + 3(0
.
05) + 4(0
.
35) + 5(0
.
275) = 3
.
45
µ
2
=
E
[
X
2
] = 1
2
(0
.
125) + 2
2
(0
.
2) + 3
2
(0
.
05) + 4
2
(0
.
35) + 5
2
(0
.
275) = 13
.
85
.
Therefore, we have
E
[
S
] = Λ
µ
1
= 4(3
.
45) = 13
.
8
and
V
[
S
] = Λ
µ
2
= 4(13
.
85) = 55
.
4
.
Finally,
we know that the
mgf
of
S
is given by
m
S
(
t
) = exp [Λ
{
m
X
(
t
)
−
1
}
]
where
m
X
(
t
) =
E
(
e
tX
)
=
0
.
125
e
t
+ 0
.
2
e
2
t
+ 0
.
05
e
3
t
+ 0
.
35
e
4
t
+ 0
.
275
e
5
t
. So, we get
m
S
(
t
) = exp
(
0
.
5
e
t
+ 0
.
8
e
2
t
+ 0
.
2
e
3
t
+ 1
.
4
e
4
t
+ 1
.
1
e
5
t
−
4
)
.
(b) Since the minimum value of the support of both
F
1
(
x
)
and
F
2
(
x
)
is 1 , it must be the case that
S
≥
N
. Therefore,
S
≤
3
implies
N
≤
3
. Hence, we can see that:
P
(
S
≤
3) =
∞
X
n
=0
P
(
S
≤
3
|
N
=
n
)
P
(
N
=
n
)
=
3
X
n
=0
P
(
S
≤
3
|
N
=
n
)
e
−
4
4
n
n
!
=
P
(
S
= 0
|
N
= 0)
e
−
4
+
3
X
n
=1
P
n
X
i
=1
X
i
≤
3
N
=
n
!
e
−
4
4
n
n
!
=
e
−
4
+ 4
P
(
X
1
≤
3)
e
−
4
+ 8
P
(
X
1
+
X
2
≤
3)
e
−
4
+
32
3
P
(
X
1
+
X
2
+
X
3
≤
3)
e
−
4
=
1
3
e
−
4
n
3 + 12
F
(3) + 24
F
∗
(2)
(3) + 32
F
∗
(3)
(3)
o
.
2
Now, clearly
F
(3) = 0
.
125+0
.
2+0
.
05 = 0
.
375
and
F
∗
(3)
(3) =
P
(
X
1
+
X
2
+
X
3
≤
3) =
P
(
X
1
= 1
, X
2
=
1
, X
3
= 1) = (0
.
125)
3
(since
X
i
≥
1
). Finally, we have:
F
∗
(2)
(3) =
∞
X
n
=0
F
(3
−
n
)
p
(
n
) =
2
X
n
=1
F
(3
−
n
)
p
(
n
) =
F
(2)
p
(1) +
F
(1)
p
(2)
= (0
.
125 + 0
.
2)
×
0
.
125 + 0
.
125
×
0
.
2 = 0
.
065625
.
Therefore,
P
(
S
≤
3) =
1
3
e
−
4
3 + 12(0
.
375) + 24(0
.
065625) + 32(0
.
125)
3
= 0
.
0558
.
4. Let
S
=
∑
N
i
=1
X
i
be a random sum with
N
having
mgf
m
N
(
t
)
. Also, assume that the
X
i
’s are independent
of
N
and are
i.i.d.
such that
P
(
X
i
= 0) =
p >
0
(i.e., the
X
i
’s can take the value zero).
Show that
P
(
S
= 0) =
m
N
(ln
p
)
.
Solution:
Since the
X
i
’s are non-negative, the only way for
∑
n
i
=1
X
i
to be equal to zero is for all the
X
i
’s
(
i
= 1
, . . . , n
)
to be 0 . Therefore, we have
P
(
S
= 0) =
P
N
X
i
=1
X
i
= 0
!
=
∞
X
n
=0
P
N
X
i
=1
X
i
= 0
N
=
n
!
p
N
(
n
)
=
∞
X
n
=0
P
n
X
i
=1
X
i
= 0
N
=
n
!
p
N
(
n
)
=
∞
X
n
=0
P
n
X
i
=1
X
i
= 0
!
p
N
(
n
)
=
P
(0 = 0)
p
N
(0) +
∞
X
n
=1
P
(
X
1
= 0
, . . . , X
n
= 0)
p
N
(
n
)
=
p
N
(0) +
∞
X
n
=1
n
Y
i
=1
P
(
X
i
= 0)
p
N
(
n
)
=
p
0
p
N
(0) +
∞
X
n
=1
p
n
p
N
(
n
)
=
∞
X
n
=0
p
n
p
N
(
n
)
=
E
(
p
N
)
=
E
(
e
N
ln
p
)
=
m
N
(ln
p
)
.
5. For a certain insurance portfolio, the individual claim amounts,
X
1
, X
2
, . . .
, are exponentially distributed with
mean
θ
. Further, the number of claims made on the portfolio during some fixed period,
N
, is independent of
3
Your preview ends here
Eager to read complete document? Join bartleby learn and gain access to the full version
- Access to all documents
- Unlimited textbook solutions
- 24/7 expert homework help
the
X
i
’s and follows a distribution with
pmf
:
p
N
(
n
) = P(
N
=
n
) =
2
n
n
!6
.
389
,
n
= 1
,
2
,
3
, . . .
The aggregate claim amount is given by the random sum
S
=
∑
N
i
=1
X
i
.
Find
E
(
S
)
and
V
(
S
)
.
Solution:
Here we have:
E
(
N
) =
∞
X
n
=1
n
2
n
n
!6
.
389
=
2
6
.
389
∞
X
n
=1
2
n
−
1
(
n
−
1)!
=
2
6
.
389
∞
X
m
=0
2
m
m
!
=
2
6
.
389
e
2
= 2
.
313
,
since
∑
∞
m
=0
2
m
m
!
=
e
2
= 7
.
389
. Similarly, we have
E
{
N
(
N
−
1)
}
=
∞
X
n
=1
n
(
n
−
1)2
n
n
!6
.
389
=
2
2
6
.
389
∞
X
n
=2
2
n
−
2
(
n
−
2)!
=
4
6
.
389
∞
X
m
=0
2
m
m
!
=
4
6
.
389
e
2
= 4
.
626
,
which implies that
V(
N
) =
E
(
N
2
)
− {
E
(
N
)
}
2
=
E
{
N
(
N
−
1)
}
+
E
(
N
)
− {
E
(
N
)
}
2
= 4
.
626 + 2
.
313
−
2
.
313
2
= 1
.
589
.
Therefore, we get
E
(
S
) =
E
(
N
)
E
(
X
1
) = 2
.
313
θ,
and
V(
S
) =
E
(
N
) V (
X
1
) +
{
E
(
X
1
)
}
2
V(
N
) = 2
.
313
θ
2
+ 1
.
589
θ
2
= 3
.
902
θ
2
.
6. Suppose that we have a portfolio of insurance policies for which claim amounts are always of size
$100
,
000
.
Further, suppose that
N
, the number of claims made in a specified period, has an expectation of
E
(
N
) = 10
.
We are trying to decide whether to purchase an excess-of-loss reinsurance scheme with retention level
M
, the
premium charge of which is set equal to
Premium
=
E
(
S
Z
)
1 +
10000
M
,
where
Z
i
= (
X
i
−
M
)
I
(
X
i
>M
)
represents the amount of the
i
th
claim for which the reinsurer is liable and
E
(
S
Z
) =
E
∑
N
i
=1
Z
i
. In other words, the premium charged is equal to the expected liability of the reinsurer
plus a surcharge which depends on the size of the retention level.
(a) What is the expected claim amount for which we are liable if we employ the reinsurance?
What is
our total expected cost, including the reinsurance premium? Does it seem worthwhile to purchase the
reinsurance on the grounds of reducing our expected costs?
(b) Suppose we have a capital reserve of
$1
,
150
,
000
. What level of reinsurance should we choose in order
to minimise the chance that our total costs exceed our reserve?
4
Solution:
(a) As long as
M <
100000
, the amount of any claim for which we are liable is
Y
i
=
M
and we see that
S
Y
=
∑
N
i
=1
Y
i
=
NM
. Thus, our expected liability is
E
(
Y
i
) =
M
and our expected total liability
is
E
(
S
Y
) =
E
(
N
)
×
M
= 10
M
. Of course, if
M
≥
100000
then
Y
i
= 100000
,
E
(
Y
i
) = 100000
and
S
Y
= 100000
N
and
E
(
S
Y
) = 1000000
. On the other hand,
E
(
S
Z
) =
E
∑
N
i
=1
Z
i
=
E
{
N
(100000
−
M
)
}
= 1000000
−
10
M
, provided
M <
100000 [
and
E
(
S
Z
) = 0
otherwise]. Thus, the total cost to
us under a reinsurance scheme with a retention level of
M <
100000
is
10
M
+ (1000000
−
10
M
)
1 +
10000
M
= 1000000 +
1000000
M
−
100000
>
1000000
since
M <
100000
implies
1000000
M
>
100000
. Thus, since 1000000 is the expected cost for no rein-
surance (or, equivalently, reinsurance schemes with
M
≥
100000
), there is no benefit to reinsurance
from the perspective of expected costs.
(b) We have:
P
(
Total Cost
>
Reserve
) =
P
NM
+
E
(
S
Z
)
1 +
10000
M
>
1150000
=
P
NM >
1150000
−
(1000000
−
10
M
)
1 +
10000
M
=
P
N >
10 +
250000
M
−
10
10
M
2
.
So, we need to make
10+
250000
M
−
10
10
M
2
as large as possible (since such a choice will make the probability
in question as small as possible). Taking derivatives and setting to zero yields the equation:
−
250000
M
2
+
2
×
10
10
M
3
= 0
=
⇒
M
= 80000
So, it appears we should choose a reinsurance retention level of
M
= $80
,
000
. [NOTE: The fact that
this critical point is a maximum instead of a minimum can easily be seen by taking second derivatives
and noting that they are negative at the point in question.] However, since the distribution of
N
is
discrete and
10 +
250000
80000
−
10
10
80000
2
= 11
.
5625
, we see that any value of
M
which makes
10 +
250000
M
−
10
10
M
2
>
11
will actually produce the same chance that the total cost will exceed our reserve in this
case. Therefore, as
10 +
250000
50000
−
10
10
50000
2
= 11
and
10 +
250000
100000
−
10
10
100000
2
= 11
.
5
>
11
, we see that any
50000
< M
≤
100000
will be equivalent in this case. Thus, it again appears that the choice of no
reinsurance is the best option, as it has minimum risk and minimum cost in this case.
7. Consider a portfolio of insurance policies where the number of claims has a binomial distribution with pa-
rameters
n
= 1
,
000
and
p
= 0
.
01
. Claim severities are independent of claim numbers, and the claim severity
distribution is assumed to be exponential with mean
$100
. The insurer takes out an individual excess of loss
reinsurance contract with a retention level of
$200
.
Calculate the mean of
S
Y
, where
S
Y
is the aggregate
claims paid by the insurer net of reinsurance (i.e., after the XoL scheme is applied).
Solution:
5
One way to find
E
(
S
Y
)
is to use:
E
(
S
Y
) =
E
(
S
X
)
−
E
(
S
Z
)
E
(
N
) = 1000
×
0
.
01 = 10
E
(
X
) = 100
The reinsurer’s mean claim amount per claim, E(Z), is:
E
(
Z
) =
Z
∞
200
(
x
−
200)
f
(
x
)
dx
=
Z
∞
200
(
x
−
200)0
.
01
e
−
0
.
01
x
dx
Using the substitution
u
=
x
−
200
:
E
(
Z
) =
Z
∞
0
u
0
.
01
e
−
0
.
01(
u
+200)
du
=
e
−
2
Z
∞
0
u
0
.
01
e
−
0
.
01
u
du
The integral part of the expression is the mean of a exponential distribution with mean 100, so:
E
(
Z
) = 100
e
−
2
Therefore,
E
(
S
Y
) =
E
(
S
X
)
−
E
(
S
Z
) =
E
(
N
)(
E
(
X
)
−
E
(
Z
)) = 10
(
100
−
100
e
−
2
)
= 864
.
66
Alternatively, we could find
E
(
S
Y
)
directly as
E
(
N
)
E
(
Y
)
.
8. Suppose that
S
represents the aggregate claim amount from a portfolio which makes a negative binomial
number of claims with parameters
k
= 20
and
q
= 0
.
5
and that these claims are Gamma distributed with
shape parameter
α
= 3
and scale parameter
θ
= 0
.
05
.
(a) Estimate the probability that
S
is less than
4
.
5
using the normal approximation.
(b) Repeat this exercise assuming that the portfolio makes a Poisson number of claims with rate parameter
λ
= 20
. Compare your approximate answer to the "exact" answer
0
.
9654
. Furthermore, compare your
approximate answers here with those from part (a). Discuss your findings.
Solution:
(a) We have
E
(
X
i
) =
µ
1
=
αθ
= 3(0
.
05) = 0
.
15
and
E
(
X
2
i
)
=
µ
2
=
α
(
α
+ 1)
θ
2
= 3(4)
(
0
.
05
2
)
= 0
.
03
.
So via applying the relevant formulas from course notes, we have:
E
(
S
) =
k
(
q
−
1
−
1
)
µ
1
= 20(2
−
1)(0
.
15) = 3
V
(
S
) =
k
(
q
−
1
−
1
)
µ
2
+
(
q
−
1
−
1
)
µ
2
1
= 20(2
−
1)
0
.
03 + (2
−
1)
(
0
.
15
2
)
= 1
.
05
Thus, we obtain
P
(
S
≤
4
.
5) =
P
S
−
3
√
1
.
05
≤
4
.
5
−
3
√
1
.
05
≈
Φ
4
.
5
−
3
√
1
.
05
= Φ(1
.
46385) = 0
.
9283825
.
(b) We again have
E
(
X
i
) =
µ
1
=
αθ
= 3(0
.
05) = 0
.
15
and
E
(
X
2
i
) =
µ
2
=
α
(
α
+ 1)
θ
2
= 3(4)
(
0
.
05
2
)
=
0
.
03
. So, in the case of a Poisson number of claims, we have:
E
(
S
) =
λµ
1
= 20(0
.
15) = 3
V
(
S
) =
λµ
2
= 20(0
.
03) = 0
.
6
6
Your preview ends here
Eager to read complete document? Join bartleby learn and gain access to the full version
- Access to all documents
- Unlimited textbook solutions
- 24/7 expert homework help
Thus, we obtain
P
(
S
≤
4
.
5) =
P
S
−
3
√
0
.
6
≤
4
.
5
−
3
√
0
.
6
≈
Φ
4
.
5
−
3
√
0
.
6
= Φ(1
.
9365) = 0
.
9735968
.
As given in the question, the "exact" value is
0
.
9654
, indicating that the normal approximation
is rather accurate in this case.
Moreover, we see that the approximate probability in this case is
noticeably higher than in the case of negative binomial claim numbers, despite the fact that the
expected number of claims is the same in each case. This is due to the greater variance in claim
numbers under the negative binomial model, which indicates that larger total claim amounts are
somewhat more likely in this model than under the Poisson claim number model.
9. The employees of a company are covered by group life insurance which pays a specified benefit amount if an
employee dies. There are two types of employee, and the life insurance benefits differ by employee type.
For
junior
employees, the benefit amount is
$20
,
000
, and the probability of dying during a year is
0
.
012
.
There are 250 junior employees.
For
senior
employees, the benefit amount is
$50
,
000
, and the probability of dying during a year is
0
.
008
.
There are 1,250 senior employees.
The aggregate claims payable during the year are assumed to conform to the individual risk model.
Calculate the probability that the aggregate claims payable in a given year will exceed
$900
,
000
, using a
normal approximation.
Solution:
We need to first find
E
(
S
)
and
V
(
S
)
:
E
(
S
) =
X
i
q
i
µ
i
= 1
,
250
×
50
,
000
×
0
.
008 + 250
×
20
,
000
×
0
.
012 = 560
,
000
V
(
S
) =
X
i
q
i
(
σ
2
i
+ (1
−
q
i
)
µ
2
i
)
=
X
i
q
i
(1
−
q
i
)
µ
2
i
= 1
,
250
×
50
,
000
2
×
0
.
008
×
0
.
992 + 250
×
20
,
000
2
×
0
.
012
×
0
.
988
= 2
.
59856
×
10
10
We now assume:
S
∼
N
(560
,
000
,
2
.
59856
×
10
10
)
.
So we have
P
(
S >
900
,
000) =
P
N
(0
,
1)
>
900
,
000
−
560
,
000
√
2
.
59856
×
10
10
= 1
−
P
(
N
(0
,
1)
<
2
.
109175)
= 0
.
0175
.
7
10. Suppose that
X
has a Gamma distribution with parameters
α
and
θ
.
(a) Suppose that
k
= 2
α
is a positive integer. Show that
Y
= 2
X/θ
has a chi-squared distribution with
k
degrees of freedom. Note that the chi-squared distribution
pdf
is as follows:
f
(
x
) =
1
2
k/
2
Γ(
k/
2)
x
(
k/
2)
−
1
e
−
x/
2
.
(b) Suppose that you wanted to approximate the probability that a compound distributed quantity
S
ex-
ceeded some value using the translated Gamma method, but you only had chi-squared tables at your
disposal. Discuss how your result from part (a) would be useful.
(c) Suppose that
E
(
S
) = 10
,
V
(
S
) = 30
and
Skew(
S
) = 270
.
Use the translated Gamma approximation
and chi-squared tables to estimate
P
(
S
≤
20
.
9)
. Also, estimate the value of
s
which solves the equation
P
(
S
≤
s
) = 0
.
99
.
Solution:
(a) We have
Y
= 2
θ
−
1
X
, so that
X
=
1
2
θY
. Thus, a simple change of variable shows that:
f
Y
(
y
) =
f
X
1
2
θy
dx
dy
=
1
θ
α
Γ(
α
)
1
2
θy
α
−
1
e
−
θ
−
1
(
1
2
θy
)
1
2
θ
=
1
θ
α
Γ(
α
)
1
2
α
θ
α
y
α
−
1
e
−
1
2
y
=
1
2
α
Γ(
α
)
y
α
−
1
e
−
1
2
y
=
1
2
k/
2
Γ(
k/
2)
y
(
k/
2)
−
1
e
−
1
2
y
where
k
= 2
α
. Thus,
Y
has a chi-squared distribution with
k
= 2
α
degrees of freedom.
(b) As noted in lectures, if
Y
∼
G
(
α
g
, θ
g
)
and
X
=
Y
+
k
is the translated Gamma approximation to
S
, we have:
P
(
S
≤
s
)
≈
P
(
X
≤
s
) =
P
(
Y
≤
s
−
k
) =
P
(
2
θ
−
1
g
Y
≤
2
θ
−
1
g
(
s
−
k
)
)
=
P
(
χ
2
2
α
≤
2
θ
−
1
g
(
s
−
k
)
)
(c) From the given information, we can calculate the coefficient of skewness for
S
as
ρ
S
=
270
30
3
/
2
= 1
.
643
.
Thus, the appropriate translated Gamma distribution for use in approximating
S
has parameters
k, α
g
and
θ
g
which solve the equations:
1
.
643 =
ρ
S
=
2
√
α
g
,
30 =
α
g
θ
2
g
,
10 =
k
+
α
g
θ
g
Solving the first equation shows that
α
g
= 4
/
(1
.
643)
2
= 1
.
482
, which implies that
θ
g
=
p
30
/
1
.
482 =
4
.
5
and
k
= 10
−
4
.
5(1
.
482) = 3
.
333
. So, letting
Y
∼
G
(1
.
482
,
4
.
5)
, we have
P
(
S
≤
20
.
9)
≈
P
(
Y
+ 3
.
333
≤
20
.
9) =
P
(
Y
≤
17
.
567) =
P
2
4
.
5
Y
≤
7
.
808
≈
P
(
χ
2
3
≤
7
.
808
)
≈
0
.
95
[NB: The actual value of
P
(
Y
≤
17
.
567)
is
0
.
9512
.] Similarly, the 99th percentile of
S
is the value
s
0
.
99
which satisfies:
0
.
99 =
P
(
S
≤
s
0
.
99
)
≈
P
(
Y
≤
s
0
.
99
−
3
.
333)
≈
P
χ
2
3
≤
2
4
.
5
(
s
0
.
99
−
3
.
333)
8
Now, the 99th percentile of a chi-squared distribution with three degrees of freedom is
11
.
34
, so that
we can estimate the 99 th percentile of
S
as the solution to:
2
4
.
5
(
s
0
.
99
−
3
.
333) = 11
.
34
=
⇒
s
0
.
99
= 28
.
85
[NB: The 99th percentile of
Y
is actually
25
.
36
, which implies that a direct translated Gamma
approximation (i.e., without using the chi-squared distribution as an intermediary) yields
s
0
.
99
=
25
.
36 + 3
.
333 = 28
.
69
.]
11.
An
insurance
portfolio
consists
of
n
policies.
For
each
policy,
the
number
of
claims
made
during
the
term
of
coverage
has
a
Poisson
distribution
with
parameter
q
(i.e.,
the
same
for
each
policy).
Furthermore,
the
individual
claim
amounts
have
mean
µ
and
standard
deviation
σ
(again,
the
same
for
each
policy).
Finally,
suppose
that
q
is
random
and
comes
from
a
distribution
having
mean
η
1
and
second
raw
moment
η
2
.
Calculate
the
mean
and
variance
of
S
,
the
aggregate
claim
amount
for
this
portfolio.
Compare
your
results
with
those
of
the
case,
discussed
in
Section
2.4.2
of
the
course
notes
(i.e.,
Parameter
Variability),
in
which
each
policy
has
a
different
(random)
claim
rate
q
i
each
independently
distributed
with
common
mean
η
1
and
second
raw
moment
η
2
(but
still
assume
that
the
policies
have
the
same
claim
amount
distribution).
Discuss
the
reasons
for
any
differences.
Solution:
N/A (The content in this question is no longer examinable.)
12. An insurance company offers three types of policy.
Claims are binomially distributed, and all policies are
assumed to be independent.
For policy type A there are 500 policies, claim amounts follow a Lognormal distribution with parameters
µ
= 5
and
σ
2
= 1
.
2
, and the probability of a claim being made per annum by any one policy is
q
1
= 0
.
03
.
For policy type B there are 300 policies, claim amounts follow a Gamma distribution with parameters
α
= 0
.
15
and
θ
= 2000
and the probability of a claim being made per annum by any one policy is
q
2
= 0
.
02
.
For policy type C there are 100 policies, claim amounts are fixed at
$300
and the probability of a claim being
made per annum by any one policy is
q
3
= 0
.
05
.
Let
S
be the total claim amount on the entire portfolio.
Using the normal approximation, calculate the
probability that
S
will exceed
$12
,
000
.
Solution:
E
(
S
) =
n
X
i
=1
q
i
µ
i
=
500
X
A
0
.
03 exp
µ
+
σ
2
2
+
300
X
B
0
.
02
αθ
+
100
X
C
0
.
05(300)
=
500
X
A
0
.
03 exp
5 +
1
.
2
2
+
300
X
B
0
.
02(0
.
15)(2000) +
100
X
C
0
.
05(300)
=
500
X
A
0
.
03(270
.
4264) +
300
X
B
0
.
02(300) + 1500 = 7356
.
396
9
Your preview ends here
Eager to read complete document? Join bartleby learn and gain access to the full version
- Access to all documents
- Unlimited textbook solutions
- 24/7 expert homework help
V
(
S
) =
n
X
i
=1
q
i
(
σ
2
i
+ (1
−
q
i
)
µ
2
i
)
⇒
V
(
S
) =
500
X
A
0
.
03
(
σ
2
A
+ (0
.
97)270
.
426
2
)
+
300
X
B
0
.
02
(
σ
2
B
+ (0
.
98)300
2
)
+
100
X
C
0
.
05(0
.
95)300
2
σ
2
A
= exp
(
2
µ
+ 2
σ
2
)
−
270
.
4264
2
= 169
,
671
.
2
σ
2
B
=
αθ
2
= 0
.
15(2000)
2
σ
2
C
= 0
⇒
V
(
S
) =
500
X
A
0
.
03(240607
.
5) +
300
X
B
0
.
02
(
0
.
15(2000)
2
+ (0
.
98)(300)
2
)
+ 427
,
500
⇒
V
(
S
) = 3
,
609
,
112 +
300
X
B
0
.
02
(
0
.
15(2000)
2
+ (0
.
98)(300)
2
)
+ 427
,
500 = 8
,
165
,
812
Hence, we get
P
(
S >
12
,
000) = 1
−
P
(
S
≤
12
,
000) = 1
−
P
Z
≤
12
,
000
−
E
(
S
)
p
V
(
S
)
!
= 1
−
P
(
Z
≤
1
.
625) = 0
.
052
.
13. A portfolio of term-life assurances is composed of 10,000 independent policies, each with probability of death
during the period of cover of
q
i
= 0
.
001
. For 4000 policies, the death benefit is
$100
,
000
and the premium
charged is
$150
. On the remaining 6000 policies, the death benefit is
$500
,
000
and the premium charged is
$750
. Let
S
be the total claim amount made on the entire portfolio.
(a) Calculate
E
(
S
)
and
V
(
S
)
. Also, use the normal approximation to estimate the probability that
S
exceeds
the total premiums charged,
4000($150) + 6000($750) = $5
,
100
,
000
.
(b) Suppose you have available only
χ
2
-tables. Use the translated Gamma method applied to
e
S
, the col-
lective risk model approximation to
S
, to estimate the chance that the aggregate claim amount exceeds
$5
,
100
,
000
. Discuss whether this estimate is reliable.
(c) Based on the estimate of part
(
a
)
, the risk of the portfolio is deemed too high. To combat this, premiums
are to be raised by
$
P
for policies with benefit
$500
,
000
and by
$2
P
for policies with benefit
$100
,
000
.
However, policyholders with benefit
$500
,
000
will be given the option to switch their policy to the
$100
,
000
benefit level.
It is believed that for every
$9
increase in the premium, 450 policyholders
will choose to switch their policy type, while 170 policyholders with the
$100
,
000
benefit and 110
policyholders with the
$500
,
000
benefit will discontinue their policies. Find the value of
P
which will
reduce the chance of
S
exceeding total premiums to
5%
(as measured by normal approximation method).
Solution:
(a) We have:
E
(
S
) =
∑
10000
i
=1
q
i
µ
i
= 4000(0
.
001)(100000) + 6000(0
.
001)(500000) = 3400000
V
(
S
) =
10000
X
i
=1
q
i
σ
2
i
+ (1
−
q
i
)
µ
2
i
= 4000(0
.
001)(0
.
999)
(
100000
2
)
+ 6000(0
.
001)(0
.
999)
(
500000
2
)
= 1
.
53846
×
10
12
.
10
Therefore,
P
(
S >
5100000) =
P
S
−
3400000
√
1
.
53846
×
10
12
>
5100000
−
3400000
√
1
.
53846
×
10
12
≈
1
−
Φ
5100000
−
3400000
√
1
.
53846
×
10
12
= 1
−
Φ(1
.
37)
≈
0
.
0853
.
So, there is approximately an
8
.
5%
chance that the total claim costs will exceed the total premiums
collected.
(b) The collective risk model approximation
˜
S
is a compound Poisson quantity with rate
Q
=
∑
10000
i
=1
q
i
=
4000(0
.
001) + 6000(0
.
001) = 4 + 6 = 10
and an individual term distribution which has first three
moments:
E
(
Z
) = 0
.
4(100000) + 0
.
6(500000) = 340000
E
(
Z
2
)
= 0
.
4
(
100000
2
)
+ 0
.
6
(
500000
2
)
= 1
.
54
×
10
11
E
(
Z
3
)
= 0
.
4
(
100000
3
)
+ 0
.
6
(
500000
3
)
= 7
.
54
×
10
16
.
So,
E
(
˜
S
) =
Q
×
E
(
Z
) = 3400000
, V
(
˜
S
) =
Q
×
E
(
Z
2
)
= 1
.
54
×
10
12
and
ρ
˜
S
=
Skew(
˜
S
)
{
V
(
˜
S
)
}
3
/
2
=
Q
×
E
(
Z
3
)
{
1
.
54
×
10
12
}
3
/
2
= 0
.
39454
.
Solving the system of equations:
ρ
˜
S
= 2
/
√
α
g
;
V
(
˜
S
) =
α
g
θ
2
g
;
E
(
˜
S
) =
k
+
α
g
θ
g
leads to the parameters
α
g
= 25
.
6968
, θ
g
= 244805
.
195
and
k
=
−
2890716
.
18
for the approximating
translated Gamma random variable
X
=
Y
+
k
. Thus, we have
P
(
S >
5100000)
≈
P
(
˜
S >
5100000)
≈
P
(
Y >
5100000 + 2890716
.
18)
=
P
(2
Y/
244805
.
195
>
2(7990716
.
18)
/
244805
.
195)
≈
P
χ
2
(51
.
4)
>
65
.
28
Now, there are various ways of getting at this probability. One is to just use the
χ
2
(50)
distribution,
note that
65
.
28
is almost exactly half-way between
63
.
17
and
67
.
50
, and thus approximate the desired
probability using a linear interpolation of 0.075. An alternative, more accurate, linear interpolation,
would first find the
0
.
9
-quantiles and
0
.
95
-quantiles for
51
.
4
degrees of freedom as
63
.
17 +
51
.
4
−
50
60
−
50
(74
.
40
−
63
.
17) = 64
.
74
and
67
.
50 +
51
.
4
−
50
60
−
50
(79
.
08
−
67
.
50) = 69
.
12
.
Thus, the linear interpolation for the actual probability is:
0
.
05 +
65
.
28
−
64
.
74
69
.
12
−
64
.
74
(0
.
1
−
0
.
05) = 0
.
938
.
Alternatively, we could use a normal approximation to the
χ
2
(51
.
4)
distribution to see that:
P
χ
2
(51
.
4)
>
65
.
28
≈
P
N
(0
,
1)
>
65
.
28
−
51
.
4
√
102
.
8
= Φ(1
.
37) = 0
.
0853
,
11
which, not surprisingly, is the same as for the original normal approximation of part (a). Using a
computer (which is not an option for the examination, of course), we see that the actual answer is
0
.
0922
, which agrees quite well with the "double" linear interpolation based on the chi-squared tables.
Finally, we note that the difference in the variance estimate for
e
S
and for
S
itself is
∑
10000
i
=1
q
2
i
µ
2
i
=
1
.
54
×
10
9
. This seems like a large value in absolute terms; however, it represents only
0
.
1%
of the
overall variance of
S
, and thus the collective risk model approximation should give quite reliable
estimates for the individual risk model.
(c) For the given situation, we see that the new total premiums collected will be:
4000 + 50
P
−
170
9
P
(150 + 2
P
) +
6000
−
50
P
−
110
9
P
(750 +
P
) = 5100000
−
28000
P
Similarly, the expected total claims is given by:
E
(
S
) =
4000 + 50
P
−
170
9
P
(0
.
001)(100000)
+
6000
−
50
P
−
110
9
P
(0
.
001)(500000)
=3400000
−
28000
P
and the variance in total claims is:
V
(
S
) =
4000 + 50
P
−
170
9
P
(0
.
001)(0
.
999)
(
100000
2
)
+
6000
−
50
P
−
110
9
P
(0
.
001)(0
.
999)
(
500000
2
)
=1
.
53846
×
10
12
−
1
.
52292
P
×
10
10
Now, in order for the normal approximation method to give us an outcome of
5%
, we need:
Z
=
Premiums
−
E
(
S
)
p
V
(
S
)
= 1
.
645
=
⇒
1700000
√
1
.
53846
×
10
12
−
1
.
52292
P
×
10
10
= 1
.
645
Solving this equation yields
P
= 30
.
89
.
14. An insurance portfolio is composed of 10,000 independent policies which can make at most one claim during
the period of cover.
For 6000 policies, the probability of a claim being made is
q
1
= 0
.
007
and the claim
amount is Gamma distributed with shape parameter
α
1
= 2
and scale parameter
θ
1
= 1000
. For the remaining
4000 policies, the probability of a claim being made is
q
2
= 0
.
021
and the claim amount is Gamma distributed
with shape parameter
α
2
= 4
and scale parameter
θ
2
= 600
. Let
S
be the total claim amount made on the
entire portfolio.
(a) Using the individual risk model, calculate
E
(
S
)
and
V
(
S
)
. Also, use the normal approximation method
to assess whether there is any chance that the total claim amount,
S
, will exceed
$400
,
000
.
(b) Let
˜
S
be the collective risk model approximation to
S
. Calculate the mean, variance and standardised
coefficient of skewness of
˜
S
.
(c) Use the translated Gamma method applied to
˜
S
to estimate the chance that the total aggregate claim
amount exceeds
$300
,
000
. Do you think that this estimate is reliable in this instance? Why or why not?
[NOTE: For large values of the shape parameter, the Gamma distribution is approximately normal.]
12
Your preview ends here
Eager to read complete document? Join bartleby learn and gain access to the full version
- Access to all documents
- Unlimited textbook solutions
- 24/7 expert homework help
(d)
Suppose
that
we
believe
that
the
two
claim
probabilities,
q
1
and
q
2
are
actually
random,
such
that
E
(
q
1
) = 0
.
007
and
E
(
q
2
) = 0
.
021
while
V
(
q
1
) =
V
(
q
2
) = 0
.
000004
.
Under
this
assumption,
discuss
how
the
values
of
E
(
S
)
and
V
(
S
)
will
change
from
those
calculated
in
part
(a)
(NOTE:
you
need
not
actually
calculate
the
new
mean
or
variance).
Solution:
(a) We have:
E
(
S
) =
10000
X
i
=1
q
i
µ
i
= 6000(0
.
007)(2)(1000) + 4000(0
.
021)(4)(600)
=285600
V
(
S
) =
10000
X
i
=1
q
i
σ
2
i
+ (1
−
q
i
)
µ
2
i
=6000(0
.
007)
(2)
(
1000
2
)
+ (0
.
993)
(
2
2
) (
1000
2
)
+ 4000(0
.
021)
(4)
(
600
2
)
+ (0
.
979)
(
4
2
) (
600
2
)
=845463360
.
Therefore,
P
(
S >
400000) =
P
S
−
285600
√
84546330
>
400000
−
285600
√
845463360
≈
1
−
Φ
400000
−
285600
√
845463360
= 1
−
Φ(3
.
93)
≈
0
.
So, there is essentially no chance that
S
will exceed
$400
,
000
.
(b) The collective risk model approximation
˜
S
is a compound Poisson quantity with rate
Q
=
∑
10000
i
=1
q
i
=
6000(0
.
007) + 4000(0
.
021) = 42 + 84 = 126
and an individual term distribution which has first three
moments:
E
(
Z
) =
42
126
E
(
X
1
) +
84
126
E
(
X
2
) =
1
3
(2)(1000) +
2
3
(4)(600) = 2266
.
67
E
(
Z
2
)
=
42
126
E
(
X
2
1
)
+
84
126
E
(
X
2
2
)
=
1
3
(2)(3)
(
1000
2
)
+
2
3
(4)(5)
(
600
2
)
= 6800000
E
(
Z
3
)
=
42
126
E
(
X
3
1
)
+
84
126
E
(
X
3
2
)
=
1
3
(2)(3)(4)
(
1000
3
)
+
2
3
(4)(5)(6)
(
600
3
)
= 2
.
528
×
10
10
.
So,
E
(
˜
S
) =
Q
×
E
(
Z
) = 285600
, V
(
˜
S
) =
Q
×
E
(
Z
2
)
= 856800000
and
ρ
˜
S
=
Skew(
˜
S
)
{
V
(
˜
S
)
}
3
/
2
=
Q
×
E
(
Z
3
)
{
856800000
}
3
/
2
= 0
.
127
.
(c) Solving the system of equations:
ρ
˜
S
= 2
/
√
α
g
;
V
(
˜
S
) =
α
g
θ
2
g
;
E
(
˜
S
) =
k
+
α
g
θ
g
leads to the parameters
α
g
= 247
.
972
, θ
g
= 1858
.
82353
and
k
=
−
175336
.
19
for the approximating
translated Gamma random variable
X
=
Y
+
k
. Thus, we have
P
(
S >
300000)
≈
P
(
˜
S >
300000)
≈
P
(
Y >
300000 + 175336
.
19)
=
P
Y
−
247
.
972(1858
.
82353)
1858
.
82353
×
√
247
.
972
>
475336
.
19
−
247
.
972(1858
.
82353)
1858
.
82353
×
√
247
.
972
≈
P
(
Z >
0
.
49) = 0
.
3121
,
13
where
Z
has a standard normal distribution. [NOTE: The actual probability that a Gamma dis-
tribution with shape
247
.
972
and scale
1858
.
82353
exceeds
300000 + 175336
.
19
is 0.3057.] Finally,
we note that
∑
10000
i
=1
q
2
i
µ
2
i
= 11336640
, which is just
1
.
34%
of
V
(
S
)
, so the collective risk model
approximation is likely to be reasonably accurate.
(d)
N/A (The content in this question is no longer examinable.)
15. An insurance portfolio is composed of 7000 independent policies each of which can make at most one claim
during the period of cover. For 3000 policies, the probability of a claim being made is
q
1
= 0
.
009
and the claim
amount is Weibull distributed with parameters
γ
1
= 0
.
5
and
θ
1
= 50
. For the remaining 4000 policies, the
probability of a claim being made is
q
2
= 0
.
03
and the claim amount is Weibull distributed with parameters
γ
2
= 1
and
θ
2
= 1500
. Let
S
be the total claim amount made on the entire portfolio.
(a) Using the individual risk model, calculate
E
(
S
)
and
V
(
S
)
.
(b) We are considering changing the composition of our portfolio in order to decrease its chance of a large
total claim amount.
To do so, we plan to decrease the number of the (riskier) first type of policies.
However, we still want our portfolio to contain 7000 overall policies. Using the normal approximation
to the individual risk model, determine the number of policies of each type we should include in the
modified portfolio so that the approximate probability of the total claim amount exceeding
$450
,
000
is
1%
. Why do we consider the first type of policy riskier even though it has the lower probability of
making a claim?
(c) Let
˜
S
be the collective risk model approximation to
S
(based on the original portfolio of 3000 policies
of the first type and 4000 policies of the second type). Use the translated Gamma method applied to
˜
S
to estimate the chance that the total aggregate claim amount made on the portfolio exceeds
$250
,
000
.
Do you think that this estimate is reliable in this instance? Why or why not?
Solution:
(a) The expected value and variance of a Weibull distribution with
γ
= 0
.
5
and
θ
= 50
can be calculated
as
E
(
X
i
) = 2(50)
2
= 5000
and
V
(
X
i
) = 24(50)
4
−
(5000)
2
= 1
.
25
×
10
8
. Also, a Weibull distribution
with
γ
= 1
and
θ
= 1500
is just an exponential distribution with
θ
= 1500
, and thus has
E
(
X
i
) =
1500
and
V
(
X
i
) = 2
.
25
×
10
6
. So,
E
(
S
) =3000(0
.
009)(5000) + 4000(0
.
03)(1500) = 315000
V
(
S
) =3000(0
.
009)
1
.
25
×
10
8
+ (1
−
0
.
009)
(
5000
2
)
+ 4000(0
.
03)
2
.
25
×
10
6
+ (1
−
0
.
03)
(
1500
2
)
=4575825000
(b) The expected claim amount on each policy is
(0
.
009)(5000) = (0
.
03)(1500) = 45
, thus changing the
make-up of the portfolio will not effect the expected value of
S
. Now, we would like to choose our
portfolio make-up so that
0
.
01 =
P
(
S >
450000)
≈
1
−
Φ
450000
−
315000
√
v
,
where
v
=(3000
−
n
)(0
.
009)
1
.
25
×
10
8
+ (1
−
0
.
009)
(
5000
2
)
+ (4000 +
n
)(0
.
03)
2
.
25
×
10
6
+ (1
−
0
.
03)
(
1500
2
)
14
is the variance of
S
when we have replaced
n
type I policies with
n
type II policies. So, we need
Φ
−
1
(1
−
0
.
01) =
450000
−
315000
√
v
=
⇒
2
.
33
√
v
= 135000
=
⇒
v
= 3357033653
.
Thus, we need to choose
n
to solve:
3357033653 = (3000
−
n
)(1347975) + (4000 +
n
)(132975)
,
which yields
n
= 1003
.
12
. In other words, we should reconstruct our portfolio with
3000
−
1003 =
1997
type I policies and
4000 + 1003 = 5003
type II policies. Finally, we note while the expected
claim amount is the same for both types of policies, the variance of the claim amount for a type-I
policy is readily seen to be
(0
.
009)
1
.
25
×
10
8
+ (1
−
0
.
009)
(
5000
2
)
= 1347975
while the variance
of a type-II policy is only 132975, and thus the type-I policies are more variable, and thus riskier.
(c) The collective risk model approximation
˜
S
is a compound Poisson quantity with rate
Q
=
∑
7000
i
=1
q
i
=
3000(0
.
009) + 4000(0
.
03) = 27 + 120 = 147
and an individual term distribution which has first three
moments:
E
(
Z
) =
27
147
E
(
X
1
) +
120
147
E
(
X
2
) =
9
49
(5000) +
40
49
(1500) = 2142
.
857
E
(
Z
2
)
=
27
147
E
(
X
2
1
)
+
120
147
E
(
X
2
2
)
=
9
49
(24)
(
50
4
)
+
40
49
(2)
(
1500
2
)
= 31224489
.
8
E
(
Z
3
)
=
27
147
E
(
X
3
1
)
+
120
147
E
(
X
3
2
)
=
9
49
(720)
(
50
6
)
+
40
49
(6)
(
1500
3
)
= 2
.
168954
×
10
12
.
So,
E
(
˜
S
) =
Q
×
E
(
Z
) = 315000
, V
(
˜
S
) =
Q
×
E
(
Z
2
)
= 4590000000
and
ρ
¯
S
=
Skew(
˜
S
)
{
V
(
˜
S
)
}
3
/
2
=
Q
×
E
(
Z
3
)
{
4590000000
}
3
/
2
= 1
.
0253
.
Solving the system of equations:
ρ
¯
S
= 2
/
√
α
g
;
V
(
˜
S
) =
α
g
θ
2
g
;
E
(
˜
S
) =
k
+
α
g
θ
g
leads to the parameters
α
g
= 3
.
805
, θ
g
= 34731
.
94
and
k
= 182844
.
97
for the approximating trans-
lated Gamma random variable
X
=
Y
+
k
. Thus, we have
P
(
S >
250000)
≈
P
(
˜
S >
250000)
≈
P
(
Y >
250000
−
182844
.
97)
=
P
(2
Y/
34731
.
94
>
2(250000
−
182844
.
97)
/
34731
.
94)
≈
P
χ
2
(7
.
61)
>
3
.
87
≈
1
2
P
χ
2
(7)
>
3
.
87
+
1
2
P
χ
2
(8)
>
3
.
87
which from the given tables appears to a bit bigger than
0
.
1
, say about
0
.
15
to
0
.
2
.
[NOTE:
The actual probability that a random variable with a
Gamma(3
.
805
,
34731
.
94)
distribution exceeds
250000
−
182844
.
97
is
0
.
157
, but the above "guestimate" will suffice for full marks.] Finally, we note
that
∑
7000
i
=1
q
2
i
µ
2
i
= 14175000
, which is just
0
.
31%
of
V
(
S
)
, so the collective risk model approximation
is likely to be reasonably accurate.
16. Suppose that
S
represents the aggregate claim amount from a portfolio which makes a Poisson number of
claims with rate parameter
λ
= 20
and that these claims are Weibull distributed with parameters
θ
= 100
and
γ
= 0
.
5
.
15
Your preview ends here
Eager to read complete document? Join bartleby learn and gain access to the full version
- Access to all documents
- Unlimited textbook solutions
- 24/7 expert homework help
(a) Use the normal approximation to estimate the probability that
S
exceeds 750,000.
(b) Use the normal approximation to estimate the 98th percentile of the distribution of
S
.
(c) Repeat parts (a) and (b) using the translated Gamma approximation method.
Solution:
(a) We first note that for a Weibull distribution we saw:
E
(
X
k
i
)
=
θ
k/γ
Γ
k
γ
+ 1
Thus, in this case, we have
µ
1
=
E
(
X
i
) =
θ
2
Γ(3) =
(
100
2
)
(2!) = 20000
and
µ
2
=
E
(
X
2
i
) =
θ
4
Γ(5) =
(
100
4
)
(4!) = 2400000000
. Therefore,
E
(
S
) =
λµ
1
= 400000
and
V
(
S
) =
λµ
2
= 48000000000
. So,
using the normal approximation, we have:
P
(
S >
750000)
≈
1
−
Φ
750000
−
400000
√
48000000000
= 1
−
0
.
9449 = 0
.
0551
(b) We know that the 98th percentile of the standard normal distribution is
z
0
.
98
= 2
.
05375
. Therefore,
the approximate 98th percentile of
S
in this case is:
s
0
.
98
≈
E
(
S
) +
z
0
.
98
p
V
(
S
) = 400000 + 2
.
05375
√
48000000000 = 849954
(c) In order to use the translated Gamma approximation, we will need
µ
3
=
E
(
X
3
i
) =
θ
6
Γ(7) =
(
100
6
)
(6!) = 7
.
2
×
10
14
. This implies that the coefficient of skewness of
S
is
ρ
S
=
Skew(
S
)
V
(
S
3
/
2
)
=
λµ
3
(
λµ
2
)
3
/
2
=
µ
3
µ
3
/
2
2
√
λ
=
7
.
2
×
10
14
(24
×
10
8
)
3
/
2
√
20
=
7
.
2
×
10
2
24
3
/
2
√
20
= 1
.
369
.
Therefore, the appropriate parameters for the translated Gamma approximation are
α
g
=
4
1
.
369
2
=
2
.
133
,
θ
g
=
p
48000000000
/
2
.
133 = 150000
and
k
= 400000
−
2
.
133(150000) = 80000
. So,
P
(
S >
750000)
≈
P
(
Y
+
k >
750000) =
P
(
Y >
670000) = 0
.
0742
.
[NB:
P
(
χ
2
4
>
2
(
150000
−
1
)
(670000)
)
=
P
(
χ
2
4
>
8
.
933
)
= 0
.
0628
and
P
(
χ
2
5
>
8
.
933
)
= 0
.
1118
, and
since
2
α
g
= 4
.
266
, we might approximate
P
(
Y >
670000)
by interpolation as
0
.
0628+0
.
266(0
.
1118
−
0
.
0628) = 0
.
0758
.] Similarly, the 98th percentile of
S, s
0
.
98
, satisfies:
0
.
98 =
P
(
S
≤
s
0
.
98
)
≈
P
(
Y
+
k
≤
s
0
.
98
) =
P
(
Y
≤
s
0
.
98
−
80000)
Now, the 98th percentile of
Y
can be calculated as 910078.8. [NB:
χ
2
4
(0
.
98) = 11
.
668
and
χ
2
5
(0
.
98) =
13
.
388
, so that we could approximate the 98th percentile of
Y
by interpolation as:
1
2
×
150000
×
(11
.
668 + 0
.
266
×
(13
.
388
−
11
.
668)) = 909414
.] Therefore,
s
0
.
98
≈
910078
.
8 + 80000 = 990078
.
8
.
Note that both the probability and the quantile are somewhat different than the estimates based on
the normal approximation, and this is not surprising since the coefficient of skewness for
S
in this
case was quite large, indicating that the distribution of
S
is quite skewed and thus not amenable to
very accurate normal approximation.
16
17. An insurance company offers two types of policy.
Claims are binomially distributed, and all policies are
assumed to be independent.
For policy type A, there are 100 policies, claims amounts follow a Gamma distribution with parameters
α
= 24355
and
θ
= 0
.
25
, and the probability of a claim being made is
q
A
= 0
.
02
.
For policy type
B
, there are 200 policies, each claim is either
$1000
with probability
60%
, or
$3000
with
probability
40%
, and the probability of a claim being made is
q
B
= (0
.
015)
.
Let
S
be the total claim amount on the entire portfolio and assume that we have a cash reserve of
$
K
.
You are given that the third raw moment for a Gamma distributed random variable is:
αθ
3
(
α
+ 1)(
α
+ 2)
.
(a) Using the individual risk model and the normal approximation, estimate
K
, such that
S
will exceed
K
with probability
1%
.
(b) Let
˜
S
be the collective risk model approximation to
S
. Calculate the mean, variance, and standardised
coefficient of skewness of
˜
S
.
(c) Use the translated Gamma method applied to
˜
S
to estimate
K
, such that the chance that the total
aggregate claim amount made on the portfolio exceeds
K
is
1%
. Suppose you only have
chi-square tables
available.
Solution:
(a) In order to use the normal approximation, first we need to find the mean and variance of
S
.
E
(
S
) =
n
X
i
=1
q
i
µ
i
=
100
X
A
0
.
02(24355)(0
.
25) +
200
X
B
0
.
015(1000(0
.
6) + 3000(0
.
4))
=
100
X
A
0
.
02(6088
.
75) +
200
X
B
0
.
015(1800) = $17
,
577
.
5
σ
2
A
=
αθ
2
= 24355
(
0
.
25
2
)
E X
2
B
=
(
1000
2
)
0
.
6 +
(
3000
2
)
0
.
4
= 4
,
200
,
000
σ
2
B
=
E X
2
B
−
(
E
[
X
B
])
2
= 4
,
200
,
000
−
1800
2
= 960
,
000
V
(
S
) =
n
X
i
=1
q
i
σ
2
i
+ (1
−
q
i
)
µ
2
i
=
100
X
A
0
.
02
(
(24355)0
.
25
2
+ (1
−
0
.
02)
(
24355
2
)
0
.
25
2
)
+
200
X
B
0
.
015
(
960
,
000 + (1
−
0
.
015)1800
2
)
=
100
X
A
726
,
658
.
8244 +
200
X
B
62
,
271 = $85
,
120
,
082
.
44
.
17
Hence,
P
(
S > K
) = 0
.
01
P
Z >
K
−
E
(
S
)
p
V
(
S
)
!
= 0
.
01
=
⇒
K
−
E
(
S
)
p
V
(
S
)
= 2
.
33
=
⇒
K
=
E
(
S
) + 2
.
33
p
V
(
S
) = 17
,
577
.
5 + 2
.
33
p
85
,
120
,
082
.
44 = $39
,
074
.
21
.
(b) We know that
˜
S
is compound Poisson with
Q
=
n
X
i
=1
q
i
= 100(0
.
02) + 200(0
.
015) = 5
E
(
Z
) =
100(0
.
02)
5
E
(
X
A
) +
200(0
.
015)
5
E
(
X
B
) =
2
5
6088
.
75 +
3
5
1800 = 3515
.
5
E
(
Z
2
)
=
2
5
E
(
X
2
A
)
+
3
5
E
(
X
2
B
)
=
2
5
α
(
α
+ 1)
θ
2
+
3
5
4
,
200
,
000
= 17
,
349
,
759
.
5
E X
3
B
=
(
1000
3
)
0
.
6 +
(
3000
3
)
0
.
4
= 1
.
14
×
10
10
E
(
Z
3
)
=
2
5
E
(
X
3
A
)
+
3
5
E
(
X
3
B
)
=
2
5
αθ
3
(
α
+ 1)(
α
+ 2) +
3
5
E
(
X
3
B
)
= 9
.
714
×
10
10
E
(
˜
S
) =
QE
(
Z
) = 17
,
577
.
5
V
(
˜
S
) =
QE
(
Z
2
)
= 86
,
748
,
797
.
5
˜
ρ
S
=
Skew(
˜
S
)
{
V
(
˜
S
)
}
3
/
2
=
QE
(
Z
3
)
{
QE
(
Z
2
)
}
3
/
2
=
4
.
857106
×
10
11
8
.
079699
×
10
11
≈
0
.
6011
(c)
0
.
60114931 =
2
√
α
g
⇒
α
g
= 11
.
0687
86
,
748
,
797
.
5 =
α
g
θ
2
g
⇒
θ
g
= 2799
.
5233
17
,
577
.
5 =
k
+
α
g
θ
g
⇒
k
=
−
13409
.
4886
P
(
˜
S > K
)
≈
P
(
Y > K
−
k
) = Pr
χ
2
(2
α
g
)
>
2(
K
−
k
)
/θ
g
= 0
.
01
Pr
χ
2
(22)
>
2(
K
+ 13409
.
4886)
/
2799
.
5233
= 0
.
01
Pr
χ
2
(22)
>
40
.
289
= 0
.
01
2(
K
+ 13409
.
4886)
/
2799
.
5233 = 40
.
289
K
≈
$42
,
985
.
51
18
Your preview ends here
Eager to read complete document? Join bartleby learn and gain access to the full version
- Access to all documents
- Unlimited textbook solutions
- 24/7 expert homework help
18.
(a) A compound Poisson distribution has
λ
= 5
and claim amount distribution
Pr(100) = 0
.
7
,
Pr(500) =
0
.
21
,
Pr(1000) = 1
−
0
.
7
−
0
.
21
. Determine the probability that the aggregate claims will be exactly
600
.
(b) For an insurance portfolio, the number of claims is a compound binomial distribution with
m
= 100
,
and
q
= 0
.
1
. Claim amounts have a Weibull distribution with
θ
= 2
, and
γ
= 1
. Find the variance of
the aggregate claims.
(c) Aggregate claims from a risk have a compound Poisson distribution with
λ
= 200
and an individual
claim amount distribution with is Pareto with parameters
α
= 3
and
δ
= 305
. The insurer has taken
out excess-of-loss reinsurance with a retention level of 400 .
Calculate the mean and variance of the reinsurer’s aggregate claims.
Solution:
(a) We know
P
(
S
= 600) =
P
(
X
1
= 600
|
N
= 1)
P
(
N
= 1)
+
P
(
X
1
+
X
2
= 600
|
N
= 2)
P
(
N
= 2) +
. . .
Now, given the information, there are only 2 ways to get 600 exactly:
2 claims
: 100, 500 (or 500, 100)
6 claims
: 100, 100, 100, 100, 100, 100
P
(
S
= 600) =
P
(
X
1
+
X
2
= 600
|
N
= 2)
P
(
N
= 2) +
P
6
X
i
=1
X
i
= 600
|
N
= 6
!
P
(
N
= 6)
where
P
(
N
=
n
) =
5
n
e
−
5
n
!
. Hence,
P
(
X
1
+
X
2
= 600
|
N
= 2)
P
(
N
= 2) = [(0
.
7)(0
.
21) + (0
.
21)(0
.
7)]
5
2
e
−
5
2!
= 0
.
024762
P
6
X
i
=1
X
i
= 600
|
N
= 6
!
P
(
N
= 6) = (0
.
7)
6
e
−
5
5
6
6!
= 0
.
017203
P
(
S
= 600) = 0
.
024762 + 0
.
017203
≈
0
.
0420
(b)
V
[
S
] =
mq
(
µ
2
−
qµ
2
1
)
V
[
S
] = 100(0
.
1)
(
µ
2
−
(0
.
1)
µ
2
1
)
For Weibull distribution, we have::
E
θ,γ
(
X
k
)
=
θ
k/γ
Γ
k
γ
+ 1
= 2
k
Γ(
k
+ 1)
E
θ,γ
(
X
) = 2Γ(2) = 2
E
θ,γ
(
X
2
)
= 2
2
Γ(3) = 8
V
[
S
] = 100(0
.
1)(8
−
(0
.
1)4) = 76
(c) Let
S
R
be the reinsurer’s loss distribution. Then,
E
(
S
R
) =
E
(
N
)
E
(
Z
)
= 200
Z
∞
M
(
x
−
M
)
f
X
(
x
)
dx
= 200
Z
∞
M
(
x
−
M
)
(3)305
3
(
x
+ 305)
4
dx
19
Let
y
=
x
−
M
E
(
S
R
) = 200
Z
∞
0
y
(3)305
3
(
y
+
M
+ 305)
4
dy
=
305
3
×
200
(305 +
M
)
3
Z
∞
0
y
(3)(305 +
M
)
3
(
y
+
M
+ 305)
4
dy
=
305
3
×
200
(305 +
M
)
3
E
(
Y
)
=
305
3
×
200
(305 +
M
)
3
305 +
M
2
= 5
,
708
.
49
where the third and second last equality follows from
Y
∼
Pareto
(3
,
305 +
M
)
. Hence,
Z
∞
0
y
(3)(305 +
M
)
3
(
y
+
M
+ 305)
4
dy
=
E
(
Y
) =
305 +
M
2
V
(
S
R
) =
305
3
(305 +
M
)
3
200
Z
∞
0
y
2
(3)(305 +
M
)
3
(
y
+
M
+ 305)
4
dy
=
305
3
200
(305 +
M
)
3
E
(
Y
2
)
=
305
3
200
(305 +
M
)
3
2(305 +
M
)
2
2(1)
= 8
,
048
,
971
.
63
Again for
Y
∼
Pareto
(3
,
305 +
M
)
, we have used
Z
∞
0
y
2
(3)(305 +
M
)
3
(
y
+
M
+ 305)
4
dy
=
E
(
Y
2
)
=
2(305 +
M
)
2
2(1)
.
20
Related Documents
Related Questions
SHOW YOUR WORK IN THE SPACES PROVIDED BELOW FOR FULL CREDIT.
1) Understanding Normal Distribution (2p)
a. Which normal curve has a greater mean?
b. Which normal curve has a greater standard deviation?
arrow_forward
Net Present Value
(NPV)
■ Definition
• Formula & Calculation in 6 Steps
. 2 Illustrative Examples
Advantages and Disadvantages
Net Present
Value
NPV =
= Σ
NCFt
t=0 (1+i)
arrow_forward
REWARD-TO-RISK RATIO:DEFINITION AND EXAMPLE• The reward-to-risk ratio is the slope of theline illustrated in the previous example▪ Slope = (E(RA) – Rf) / (A – 0)▪ Reward-to-risk ratio for the previous example = (20 – 8) / (1.6 – 0) = 7.5
• What if an asset has a reward-to-risk ratioof 8 (implying that the asset plots abovethe line)?
• What if an asset has a reward-to-risk ratioof 7 (implying that the asset plots belowthe line)?
arrow_forward
Applying the DDM model utilizing the
following inputs: PO, d1, d2, r, g.
When rearranging the price expression so
that we can solve for g,
g= r - d2/((PO*(1+r)-d1))
True
False
arrow_forward
Which of the following is sometimes called a currently attainable standard?
a. par
b. normal standard
Oc. theoretical standard
O d. ideal standard
arrow_forward
7. Given the following marginal revenue functions, R' (Q)
terms of R(0). Hint: Lets say u=1+Q
Oa. R(Q)
40
11Q
O b. R(Q)=R(0)+40
40(1+Q), find total revenue function in
O c. R(Q)
40
+R(0)
11Q
40
O d. R(Q)
R(0) +40
14Q
O e. R(Q)
+R(0)
1+Q
arrow_forward
Give typing answer with explanation and conclusion
arrow_forward
Which of the following correctly orders the investment rules of average accounting return (AR), internal rate of return (IRR), and net present value (NPV) from the most desirable to the least desirable?
a.
IRR, AR, NPV.
b.
AR, IRR, NPV.
c.
NPV, AR, IRR.
d.
AR, NPV, IRR.
e.
NPV, IRR, AR.
arrow_forward
Why would you use mean absolute deviation as opposed to the mean average?3. Describe a specific scenario of how you would use a pro forma financial statement?4. List and describe three time series models, and explain when would you use it? (be specific)
arrow_forward
K
Which of the following statements about rational expectations is true?
O A. Rational expectations are always accurate.
B. Rational expectations theory suggests that forecasts errors of expectations are sizable and can be predicted.
OC. Rational expectations are the same as adaptive expectations.
OD. Rational expectations are identical to optimal forecasts.
arrow_forward
Show that the lower semi-standard deviation corrected expectation has properties (UT) und (UC)
arrow_forward
what are the major assumptions in making this estimate for REV?
arrow_forward
H
Consider the three stocks in the following table. Pe represents price at time t, and Qe represents shares outstanding at time t
Stock C splits two-for-one in the last period.
Pe
81
41
82
lo
100
200
200
Rate of return
P₁
86
36
92
li
100
200
200
%
P₂
86
36
46
Required:
Calculate the first-period rates of return on the following indexes of the three stocks: (Do not round intermediate calculations.
Round your answers to 2 decimal places.)
a. A market value-weighted index
2₂
100
200
400
arrow_forward
SEE MORE QUESTIONS
Recommended textbooks for you
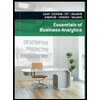
Essentials Of Business Analytics
Statistics
ISBN:9781285187273
Author:Camm, Jeff.
Publisher:Cengage Learning,
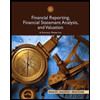
Financial Reporting, Financial Statement Analysis...
Finance
ISBN:9781285190907
Author:James M. Wahlen, Stephen P. Baginski, Mark Bradshaw
Publisher:Cengage Learning
Related Questions
- SHOW YOUR WORK IN THE SPACES PROVIDED BELOW FOR FULL CREDIT. 1) Understanding Normal Distribution (2p) a. Which normal curve has a greater mean? b. Which normal curve has a greater standard deviation?arrow_forwardNet Present Value (NPV) ■ Definition • Formula & Calculation in 6 Steps . 2 Illustrative Examples Advantages and Disadvantages Net Present Value NPV = = Σ NCFt t=0 (1+i)arrow_forwardREWARD-TO-RISK RATIO:DEFINITION AND EXAMPLE• The reward-to-risk ratio is the slope of theline illustrated in the previous example▪ Slope = (E(RA) – Rf) / (A – 0)▪ Reward-to-risk ratio for the previous example = (20 – 8) / (1.6 – 0) = 7.5 • What if an asset has a reward-to-risk ratioof 8 (implying that the asset plots abovethe line)? • What if an asset has a reward-to-risk ratioof 7 (implying that the asset plots belowthe line)?arrow_forward
- Applying the DDM model utilizing the following inputs: PO, d1, d2, r, g. When rearranging the price expression so that we can solve for g, g= r - d2/((PO*(1+r)-d1)) True Falsearrow_forwardWhich of the following is sometimes called a currently attainable standard? a. par b. normal standard Oc. theoretical standard O d. ideal standardarrow_forward7. Given the following marginal revenue functions, R' (Q) terms of R(0). Hint: Lets say u=1+Q Oa. R(Q) 40 11Q O b. R(Q)=R(0)+40 40(1+Q), find total revenue function in O c. R(Q) 40 +R(0) 11Q 40 O d. R(Q) R(0) +40 14Q O e. R(Q) +R(0) 1+Qarrow_forward
- Give typing answer with explanation and conclusionarrow_forwardWhich of the following correctly orders the investment rules of average accounting return (AR), internal rate of return (IRR), and net present value (NPV) from the most desirable to the least desirable? a. IRR, AR, NPV. b. AR, IRR, NPV. c. NPV, AR, IRR. d. AR, NPV, IRR. e. NPV, IRR, AR.arrow_forwardWhy would you use mean absolute deviation as opposed to the mean average?3. Describe a specific scenario of how you would use a pro forma financial statement?4. List and describe three time series models, and explain when would you use it? (be specific)arrow_forward
- K Which of the following statements about rational expectations is true? O A. Rational expectations are always accurate. B. Rational expectations theory suggests that forecasts errors of expectations are sizable and can be predicted. OC. Rational expectations are the same as adaptive expectations. OD. Rational expectations are identical to optimal forecasts.arrow_forwardShow that the lower semi-standard deviation corrected expectation has properties (UT) und (UC)arrow_forwardwhat are the major assumptions in making this estimate for REV?arrow_forward
arrow_back_ios
SEE MORE QUESTIONS
arrow_forward_ios
Recommended textbooks for you
- Essentials Of Business AnalyticsStatisticsISBN:9781285187273Author:Camm, Jeff.Publisher:Cengage Learning,Financial Reporting, Financial Statement Analysis...FinanceISBN:9781285190907Author:James M. Wahlen, Stephen P. Baginski, Mark BradshawPublisher:Cengage Learning
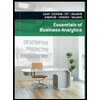
Essentials Of Business Analytics
Statistics
ISBN:9781285187273
Author:Camm, Jeff.
Publisher:Cengage Learning,
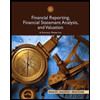
Financial Reporting, Financial Statement Analysis...
Finance
ISBN:9781285190907
Author:James M. Wahlen, Stephen P. Baginski, Mark Bradshaw
Publisher:Cengage Learning