Exam1Sol
pdf
keyboard_arrow_up
School
Southern Methodist University *
*We aren’t endorsed by this school
Course
6324
Subject
Statistics
Date
Apr 3, 2024
Type
Pages
6
Uploaded by DeanSeaUrchinPerson1125
Exam 1 for STAT 6324
Steven Chiou
Instructions:
•
This exam is open-book, open-notes, and open-internet, but it must be completed individually.
•
You have 80 minutes to complete the exam, but you do not have to use up the whole period.
•
Please submit your answers via the Canvas submission system.
•
Please submit a
Rmd
file and a
pdf
file generated by
Rmarkdown
.
•
Name your file
LastName6324exam1.pdf
and
LastName6324exam1.Rmd
.
•
Please indicate by number which questions you are answering.
•
Start a question in a new page, i.e., use
\newpage
.
•
There are 5 questions, and each question is worth 25 points. Only 4 out of 5 questions will be graded (for a
total of 100 points). You are required to answer #4, but you can choose the remaining questions to answer.
•
Please indicate the questions that you want me to grade in the following table. If you do not indicate the
questions in the following table, I will assume you want me to grade questions 1 to 4.
Question
Q1
Q2
Q3
Q4
Q5
Grade?
✓
Note:
•
If I cannot find your answer or follow your work, then you will receive a 0 for that question.
•
If you cannot compile your code, consider exploring the following compilation options:
–
eval = FALSE
to display your code without executing it.
–
error = TRUE
to display error messages instead of stopping the rendering process when an error occurs.
•
You are limited to using standard
R
packages, with the exception of
microbenchmark
,
Rcpp
, and
RcppArmadillo
.
•
You are not required to answer questions #1, 2, 3, and 5 in
Rcpp
.
Please read the following statement before you proceed:
By completing my submission, I understand that violation of this agreement and the SMU Student Code of Conduct
will result in an
0
on this exam, and it cannot be replaced, it will be averaged in (as a 0%) with my other scores.
Violations will be turned in to the Dean of Students Office.
Name:
Signature:
Questions
1. (25 points) For integers
n
≥
r
, the combination formula is
n
r
=
n
!
r
!(
n
−
r
)!
.
Define
f
(
x
) =
(
3
x
)
x
+
(
4
x
)
x
+
· · ·
+
(
40
x
)
x
.
Find the last four digits (the rightmost four digits) of
f
(1)
,
f
(2)
, and
f
(3)
.
2. (25 points) In homework 2, we considered the sample linear regression model that has the form
y
i
=
β
0
+
β
1
x
i
+
ϵ
i
, i
= 1
, . . . , n,
where
ϵ
i
is the error term with mean 0 and variance
σ
2
. As an alternative to the least-squares method, the
slope (
β
1
) can be estimated by the solution to
U
1
(
b
|
x, y
) = 0
, where the estimating function
U
1
(
b
|
x, y
)
is defined
as follows:
U
1
(
b
|
x, y
) =
1
n
n
YYYYYYY
i
=1
x
i
−
QQQQQQQ
n
j
=1
I
(
e
j
≥
e
i
)
x
j
QQQQQQQ
n
j
=1
I
(
e
j
≥
e
i
)
,
where
e
i
=
y
i
−
bx
i
. Implement
U
1
(
b
|
x, y
)
in
R
and name it
U1()
. The function
U1()
should take three arguments,
b
for the slope,
x
for the independent variable, and
y
for the response variable. Following Homework 2, we will
use the following values for
x
and
y
.
>
x
<-
mtcars
$
wt; y
<-
mtcars
$
mpg
a. Print
U1(-1, x, y)
,
U1(0, x, y)
, and
U1(1, x, y)
.
b. Plot
U1(b)
for
b
between -10 and 10, and guess where the root is. Here is a template you may use.
>
b0
<-
seq
(
-
10
,
10
,
1e-3
)
>
plot
(b0,
sapply
(b0, U1,
x =
x,
y =
y),
'
l
'
)
>
abline
(
h =
0
)
3. (25 points) Another estimating function that can be used to estimate the slope in #
2
is
U
2
(
b
|
x, y
) =
1
n
2
n
YYYYYYY
i
=1
n
YYYYYYY
j
=1
(
x
i
−
x
j
)
I
(
e
j
≥
e
i
)
.
Implement
U
2
(
b
|
x, y
)
in
R
and name it
U2()
. The function
U2()
should take three arguments,
b
for the slope,
x
for the independent variable, and
y
for the response variable. Use the
x
and
y
from #
2
to
a. print
U2(-1, x, y)
,
U2(0, x, y)
, and
U2(1, x, y)
;
b. plot
U2(b)
for
b
between -10 and 10, and guess where the root is.
4.
(25 points) Re-implement one of your implementations from #
2
and #
3
in
Rcpp
. Then, use the following
template to compare the two versions (one in
R
and the other in
Rcpp
).
>
all.equal
(
sapply
(b0, U1,
x =
x,
y =
y),
sapply
(b0, U1c,
x =
x,
y =
y))
>
microbenchmark
(
U1
(
1
,
x =
x,
y =
y),
U1c
(
1
,
x =
x,
y =
y))
5.
(25 points) The Bradley-Terry model is a simple probability model useful for comparing the relative strength of
two teams. Specifically, the Bradley-Terry model assumes that the probability that Team 1 beats Team 2 in a
head-to-head competition is given by:
P
(
Team 1 beats Team 2
) =
θ
1
θ
1
+
θ
2
,
where
θ
1
and
θ
2
are positive real-valued scores assigned to Team 1 and Team 2, respectively.
In light of
recent events, let Team 1 be the Texas Rangers and Team 2 be the Arizona Diamondbacks, we will use the
Bradley-Terry model to simulate the remainder of the World Series, which is decided by the first team to win 4
out of 7 games.
Let
θ
i
=
d
i
+
h
i
+
ϵ
i
for
i
= 1
,
2
, where
d
i
is the number of postseason wins minus the number of postseason
losses,
h
i
is the home advantage indicator (0.5 if there is a home field advantage and 0 otherwise), and
ϵ
i
is a
random error to account for uncertainties. In our case, indices
i
= 1
and 2 correspond to the Rangers and the
Diamondbacks, respectively. For simplicity, we assume
ϵ
i
is an independent normal random variable with a
mean of 0 and a standard deviation of 0.1 and is assumed to change after each game.
As of today (November 1),
d
1
= 8
and
d
2
= 4
with up to 3 games to go. The home team for each game is
pre-determined so
h
1
= 0
, h
2
= 0
.
5
for Game 5 and
h
1
= 0
.
5
, h
2
= 0
for Game 6 and potentially Game 7.
Following these criteria, the estimated probability that the Rangers will win Game 5 is 62.3%, as illustrated by
the following codes.
>
set.seed
(
5
)
>
e1
<-
rnorm
(
1
,
0
,
0.1
); e2
<-
rnorm
(
1
,
0
,
0.1
);
>
mean
(
sample
(
1
:
0
,
1000
, T,
c
(
8
+
0
+
e1,
4
+
0.5
+
e2)))
[
1
]
0.623
Use the above criteria to simulate the remainder of the series 1000 times, and then use the outcomes to estimate
the probability of the Diamondbacks making a comeback and winning the series.
Here is a potential algorithm to initiate the concept:
1. Simulate Game 5 with
d
1
= 8
and
d
2
= 4
.
2. Update
d
1
and
d
2
based on Game 5’s outcome from Step 1.
3. Simulate Game 6 with the updated
d
1
and
d
2
from Step 2.
4. Determine if Game 7 is necessary and update
d
1
and
d
2
accordingly.
5. Simulate Game 7 with the updated
d
1
and
d
2
from Step 4.
6. Repeat Steps 1 to 5 1000 times and estimate the desired probability.
Your preview ends here
Eager to read complete document? Join bartleby learn and gain access to the full version
- Access to all documents
- Unlimited textbook solutions
- 24/7 expert homework help
Solution:
1. Here is an implementation of
f
(
x
)
, along with the last four digits of
f
(1)
,
f
(2)
, and
f
(3)
.
>
f
<-
function
(x)
sum
(
choose
(
choose
(
3
:
40
, x), x))
%%
10000
>
sapply
(
1
:
3
, f)
[
1
]
817 2004 7957
2. Here is an implementation of
U
1
(
b
|
x, y
)
, along parts a and b.
>
U1
<-
function
(b, x, y) {
+
e
<-
y
-
x
*
b
+
tmp
<-
outer
(e, e,
">="
)
+
mean
(x
-
colSums
(tmp
*
x)
/
colSums
(tmp))
+
}
>
sapply
(
-
1
:
1
, U1,
x =
x,
y =
y)
[
1
]
0.6795455 0.6956203 0.7134226
>
b0
<-
seq
(
-
10
,
10
, .
001
)
>
plot
(b0,
sapply
(b0, U1,
x =
x,
y =
y),
'
l
'
,
xlab =
""
,
ylab =
""
,
main =
"2b"
)
>
abline
(
h =
0
)
>
abline
(
v =
-
5.5
)
-10
-5
0
5
10
-0.5
0.0
0.5
2b
The root of
U
1
(
b
|
x, y
) = 0
seems to be around -5.5.
3. Here is an implementation of
U
2
(
b
|
x, y
)
, along parts a and b.
>
U2
<-
function
(b, x, y) {
+
e
<-
y
-
x
*
b
+
-
sum
(
outer
(x, x,
"-"
)
*
outer
(e, e,
">="
))
/
length
(x)
/
length
(x)
+
}
>
sapply
(
-
1
:
1
, U2,
x =
x,
y =
y)
[
1
]
0.4526621 0.4805781 0.4928008
>
plot
(b0,
sapply
(b0, U2,
x =
x,
y =
y),
'
l
'
,
xlab =
""
,
ylab =
""
,
main =
"3b"
)
>
abline
(
h =
0
)
>
abline
(
v =
-
5.3
)
-10
-5
0
5
10
-0.4
-0.2
0.0
0.2
0.4
3b
The root of
U
2
(
b
|
x, y
) = 0
seems to be around -5.3. The roots of both
U
1
(
b
|
x, y
) = 0
and
U
2
(
b
|
x, y
) = 0
are
approximately equal to the least-squares slope.
>
coef
(
lm
(mpg
~
wt, mtcars))
(Intercept)
wt
37.285126
-
5.344472
4. The objective functions
U
1
(
b
|
x, y
)
and
U
2
(
b
|
x, y
)
are related in the following way
U
2
(
b
|
x, y
) =
1
n
n
YYYYYYY
i
=1
w
i
x
i
−
QQQQQQQ
n
j
=1
I
(
e
j
≥
e
i
)
x
j
QQQQQQQ
n
j
=1
I
(
e
j
≥
e
i
)
,
when
w
i
=
QQQQQQQ
n
j
=1
I
(
e
j
≥
e
i
)
n
.
Using this relationship, the implementations of the objective functions in
Rcpp
are as follows.
>
library
(Rcpp)
>
library
(microbenchmark)
>
sourceCpp
(
code =
'
+
#include <RcppArmadillo.h>
+
// [[Rcpp::depends(RcppArmadillo)]]
+
using namespace arma;
+
// [[Rcpp::export]]
+
double U1c(double b, vec x, vec y) {
+
double U = 0;
+
int n = y.n_elem;
+
vec e = y - x * b;
+
for (int i = 0; i < n; i++) {
+
vec x2 = x.elem(find(e >= e[i]));
+
U += x[i] - sum(x2) / x2.n_elem;
+
}
+
return U / n;
+
}
+
// [[Rcpp::export]]
+
double U2c(double b, vec x, vec y) {
+
double U = 0;
+
int n = y.n_elem;
+
vec e = y - x * b;
+
for (int i = 0; i < n; i++) {
+
vec x2 = x.elem(find(e >= e[i]));
+
int n2 = x2.n_elem;
+
U += x[i] * n2 - sum(x2);
+
}
+
return U / n / n;
+
}
'
)
When there are no ties in
e
i
, the implementation can be further simplified.
>
U1R2
<-
function
(b, x, y) {
+
n
<-
length
(x)
+
x2
<-
x[
order
(y
-
x
*
b,
decreasing =
T)]
+
sum
(x2
-
cumsum
(x2)
/
1
:
n)
/
n
+
}
>
U2R2
<-
function
(b, x, y) {
+
n
<-
length
(x)
+
x2
<-
x[
order
(y
-
x
*
b,
decreasing =
T)]
+
sum
(x2
*
1
:
n
-
cumsum
(x2))
/
n
/
n
+
}
>
all.equal
(
sapply
(b0, U1,
x =
x,
y =
y),
sapply
(b0, U1c,
x =
x,
y =
y))
[
1
]
TRUE
>
all.equal
(
sapply
(b0, U1,
x =
x,
y =
y),
sapply
(b0, U1R2,
x =
x,
y =
y))
[
1
]
"Mean relative difference: 0.003993193"
>
all.equal
(
sapply
(b0, U2,
x =
x,
y =
y),
sapply
(b0, U2c,
x =
x,
y =
y))
[
1
]
TRUE
>
all.equal
(
sapply
(b0, U2,
x =
x,
y =
y),
sapply
(b0, U2R2,
x =
x,
y =
y))
[
1
]
"Mean relative difference: 7.140102e-06"
There are some non-negligible differences between
U1()
and
U1R2()
(and
U1()
vs
U2R2()
) due to ties in
e
i
for
certain values of
b0
.
>
head
(y
+
5.624
*
x,
20
)
[
1
]
35.73488 37.16900 35.84768 39.48116 38.04656 37.55904 34.37768 42.34056 40.51560 38.54656
[
11
]
37.14656 39.28968 38.27752 36.45872 39.92600 40.90458 44.76028 44.77280 39.48276 44.22004
Finally, here is the timing comparison.
>
microbenchmark
(
m1 =
U1
(
1
,
x =
x,
y =
y),
m2 =
U2
(
1
,
x =
x,
y =
y),
+
m3 =
U1c
(
1
,
x =
x,
y =
y),
m4 =
U2c
(
1
,
x =
x,
y =
y))
Unit
:
microseconds
expr
min
lq
mean median
uq
max neval cld
m1
13.646 14.1910 15.04264 14.527 14.963 47.088
100
a
m2
14.887 15.4795 16.04841 15.975 16.371 20.970
100
b
m3
2.324
2.5750
2.72783
2.670
2.810
5.160
100
c
m4
2.254
2.4995
2.69961
2.580
2.685 12.885
100
c
5.
Without sacrificing generality, we will use different random normal errors in each of the replication; using the
same random normal distribution can be easily adjusted if needed. We first generate the 1000 replicates of
possible game 5 results:
>
set.seed
(
1
)
>
sim5
<-
sample
(
1
:
0
,
1000
, T,
c
(
8
+
0
+
rnorm
(
1
,
0
, .
1
),
4
+
.
5
+
rnorm
(
1
,
0
, .
1
)))
We then simulate games 6 and 7 using the parameters when they can actually happen.
>
sim6
<-
sample
(
1
:
0
,
1000
, T,
c
(
7
+
.
5
+
rnorm
(
1
,
0
, .
1
),
5
+
0
+
rnorm
(
1
,
0
, .
1
)))
>
sim7
<-
sample
(
1
:
0
,
1000
, T,
c
(
6
+
.
5
+
rnorm
(
1
,
0
, .
1
),
6
+
0
+
rnorm
(
1
,
0
, .
1
)))
Finally, to estimate the probability of the Diamondbacks making a comeback and winning the series, we need to
take into account the results of games 5, 6, and 7. Following our settings, this corresponds to a 0 for game 5, 6,
and 7. The estimated probability is calculated as follows:
>
mean
(sim5
+
sim6
+
sim7
==
0
)
[
1
]
0.064
Your preview ends here
Eager to read complete document? Join bartleby learn and gain access to the full version
- Access to all documents
- Unlimited textbook solutions
- 24/7 expert homework help
Related Documents
Recommended textbooks for you
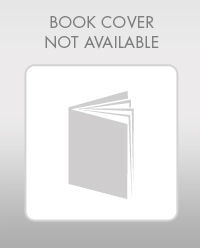
Elementary Algebra
Algebra
ISBN:9780998625713
Author:Lynn Marecek, MaryAnne Anthony-Smith
Publisher:OpenStax - Rice University
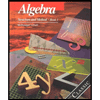
Algebra: Structure And Method, Book 1
Algebra
ISBN:9780395977224
Author:Richard G. Brown, Mary P. Dolciani, Robert H. Sorgenfrey, William L. Cole
Publisher:McDougal Littell
Recommended textbooks for you
- Elementary AlgebraAlgebraISBN:9780998625713Author:Lynn Marecek, MaryAnne Anthony-SmithPublisher:OpenStax - Rice UniversityAlgebra: Structure And Method, Book 1AlgebraISBN:9780395977224Author:Richard G. Brown, Mary P. Dolciani, Robert H. Sorgenfrey, William L. ColePublisher:McDougal Littell
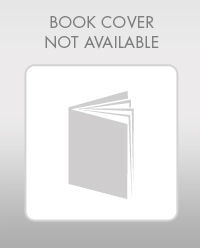
Elementary Algebra
Algebra
ISBN:9780998625713
Author:Lynn Marecek, MaryAnne Anthony-Smith
Publisher:OpenStax - Rice University
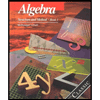
Algebra: Structure And Method, Book 1
Algebra
ISBN:9780395977224
Author:Richard G. Brown, Mary P. Dolciani, Robert H. Sorgenfrey, William L. Cole
Publisher:McDougal Littell