HW#3
pdf
keyboard_arrow_up
School
Texas A&M University *
*We aren’t endorsed by this school
Course
652
Subject
Statistics
Date
Apr 3, 2024
Type
Pages
12
Uploaded by BailiffRaven2568
Farzaneh Hashemabadi HW#3 STAT 652-Spring 2024 UIN: 233005386 Email: Farzaneh.hashemabadi@tamu.edu Problem 1. (1)
Are there any collinearity problems based on the above data? Please look at the scatter plot matrix to answer this question. -
AGE and COLLEGE: There seems to appear to be a relationship but not linear realtionship. -
AGE and INCOME: No strong linear relationship is visible. -
AGE and GENDER: Gender is typically a categorical variable, and there's no linear relationship evident. -
COLLEGE and INCOME: There is a positive trend indicating that as college level increases, income tends to increase as well. But there is no significant linear relationship. -
COLLEGE and GENDER: Like AGE and GENDER, there's no linear relationship as GENDER is categorical. -
INCOME and GENDER: There's no clear linear relationship visible.
Farzaneh Hashemabadi HW#3 STAT 652-Spring 2024 UIN: 233005386 Email: Farzaneh.hashemabadi@tamu.edu Which pairs have statistically significant correlations? Be 95% confident. • none of the pairs • college and income
• all
of the pairs • college and age
(correct) • income and gender
• age and gender
• college and gender • age and income
(2)
Use the output from the individual regression (fit these explanatory variables at a time with the response) to determine which explanatory variables should be included in the model.
Farzaneh Hashemabadi HW#3 STAT 652-Spring 2024 UIN: 233005386 Email: Farzaneh.hashemabadi@tamu.edu The p-values less than 0.05 imply the value of each predictor in the model. Given the results from effect summary table, p-value for Income = 0.0000<0.05, for Gender = 0.00248 < 0.05. So these ones should be included in the model. • gender
• income
(3)
Use the output from a stepwise regression to determine which explanatory variables should be included in the model. Compare the results of your conclusions from the stepwise to your results from the complete regression. Are they different?
Your preview ends here
Eager to read complete document? Join bartleby learn and gain access to the full version
- Access to all documents
- Unlimited textbook solutions
- 24/7 expert homework help
Farzaneh Hashemabadi HW#3 STAT 652-Spring 2024 UIN: 233005386 Email: Farzaneh.hashemabadi@tamu.edu Based on the stepwise regression output the good model should include age, income, and gender. And the results are slightly different from the individual regression because age doesn’t have significant value as a predictor in that one. (4)
Use the Cp value to determine which explanatory variables should be included in the model. Does it agree with the previous models? What is the corresponding Cp value in decision making?
Farzaneh Hashemabadi HW#3 STAT 652-Spring 2024 UIN: 233005386 Email: Farzaneh.hashemabadi@tamu.edu For each predictor that the Cp is less than p, we can conclude that is the best number for explanatory of variables. Therefore, Gender with Cp = 3.02 <4 implies the best model has p-1 = 3 parameters, which are income, age and gender. And yes it is same with the previous models. Problem 2. (1)
Use scatter plots and VIF to determine if there is evidence of collinearity in the explanatory variables. (a) Is there an evidence for collinearity according to the scatter plots? Based on the scatter plots, correlation coefficients and p-values. There is a collinearity between y and X1. But no evidence for collinearity between independent variables. Correlation coefficient, Y & X1 = 0.764
Farzaneh Hashemabadi HW#3 STAT 652-Spring 2024 UIN: 233005386 Email: Farzaneh.hashemabadi@tamu.edu P-value < 0.0001 (b) Is there an evidence for collinearity according to the VIF? Given the VIF there is no evidence for collinearity between parameters. Because all of them are around 1. (2) Use a variable selection procedure with maximum R square or any other criterion to formulate a model. Which variables are in the model? - Model for Y and X1: - Model for Y and X2:
Your preview ends here
Eager to read complete document? Join bartleby learn and gain access to the full version
- Access to all documents
- Unlimited textbook solutions
- 24/7 expert homework help
Farzaneh Hashemabadi HW#3 STAT 652-Spring 2024 UIN: 233005386 Email: Farzaneh.hashemabadi@tamu.edu - Model for Y and X3: - Model for Y and X4: By comparing the Adj RSquare, the maximum is for model formulated for Y and X1, we can conclude that this model is the best one among the individual regression models. By running the stepwise regression the model will be different. See below:
Farzaneh Hashemabadi HW#3 STAT 652-Spring 2024 UIN: 233005386 Email: Farzaneh.hashemabadi@tamu.edu Given the stepwise regression model, the model with x1, x2 and x4 predictors is the most fitted one. Also, the Adj RSquare for this model is 0.7096 which is more than the same parameter for model related to Y and x1. In conclusion, x1, x2 and x4 are the significant value predictors. (3) Fit the model with all the given independent variables. (a) What are the coefficients in the regression model y = β
0 + β
1 ∗
x1 + β
2 ∗
x2 + β
3 ∗
x3 + β
4 ∗
x4? β
0 = 80.42, β
1 = 6.18, β
2 = -2.90, β
3 = 0.11, β
4 = 0.017 (b) Is there evidence in the residual plots of a violation of the constant variance condition?
Farzaneh Hashemabadi HW#3 STAT 652-Spring 2024 UIN: 233005386 Email: Farzaneh.hashemabadi@tamu.edu From the "Residual by Predicted Plot," the residuals do not show a clear pattern; they seem to be randomly dispersed around the horizontal line that represents a residual value of zero. There is no obvious pattern such as a funnel shape or a curve which would suggest increasing or decreasing variance of the residuals as the predicted values change. There is a slight concentration of residuals around the center of the predicted value range, but without a clear increase in the spread of residuals as the predicted values increase or decrease. This slight concentration does not necessarily indicate a violation of the constant variance condition. Therefore, based on this plot alone, there is no strong evidence of a violation of the constant variance condition (no clear signs of heteroscedasticity) Problem 3 (1) Fit the linear model with both independent variables. (a) What are the coefficients of the regression model y = β0 + β1 ∗
x1 + β
2 ∗
x2? y = 20.00 -0.29 ∗
x1 + 1.40 ∗
x2 (b) Is the model significant? Be 95% confident. H0: all of the model coefficients are zero. Ha: at least one of the β
i is not equal to zero (model is significant).
Your preview ends here
Eager to read complete document? Join bartleby learn and gain access to the full version
- Access to all documents
- Unlimited textbook solutions
- 24/7 expert homework help
Farzaneh Hashemabadi HW#3 STAT 652-Spring 2024 UIN: 233005386 Email: Farzaneh.hashemabadi@tamu.edu Since the p-value <0.0001, we can reject the null hypothesis that all of the model coefficients are zero (meaning the model has no predictive capability). Therefore, we conclude that the regression model is statistically significant at the 95% confidence level. (c) Now look at the individual parameters. i. Is x1 a significant predictor? p-value = 0.8378 > 0.05, so no significant predictor. ii. Is x2 a significant predictor? p-value = 0.0019 < 0.05, so significant predictor. iii. Is there a collinearity problem according to VIF? Since VIF < 10, there is no collinearity problem. (2) Fit the following new model, y = β0 + β1 ∗
x1 + β
2 ∗
x2 + β
3 ∗
x1
2
+ β
4 ∗
x2
2
+ β
5 ∗
x1 ∗
x2 + ε
to the aphid data. Compare the linear model (reduced model) with the new model (complete model). (a)
What is the value of the test statistics and p value? F-test = 9.2219, p-value < 0.0001
Farzaneh Hashemabadi HW#3 STAT 652-Spring 2024 UIN: 233005386 Email: Farzaneh.hashemabadi@tamu.edu (b)
Did you reject H0? Be 95% confident. H0: all of the model coefficients are zero. Ha: at least one of the β
i is not equal to zero (model is significant). p-value < 0.0001, so we can reject the null. (c)
Which model has a higher adjusted R square? Model with x1 and x2: adjusted R square = 0.5200 Model with 5 predictors: adjusted R square = 0.5473, is higher than the previous model. (3) Calculate a new response variable ty = log(y), the natural logarithm of the aphid count. Fit the new model, ty = β
0 + β
1 ∗
x1 + β
2 ∗
x2 + ε
to the aphid data.
Farzaneh Hashemabadi HW#3 STAT 652-Spring 2024 UIN: 233005386 Email: Farzaneh.hashemabadi@tamu.edu (a) Which has the highest Adjusted R square? •
y = β
0 + β
1 ∗
x1 + β
2 ∗
x2 + ε
•
y = β
0 + β
1 ∗
x1 + β
2 ∗
x2 + β
3 ∗
x12 + β
4 ∗
x22 + β
5 ∗
x1 ∗
x2 + ε
•
ty = β
0 + β
1 ∗
x1 + β
2 ∗
x2 + ε
(b) Which has a lowest RMSE? •
y = β
0 + β
1 ∗
x1 + β
2 ∗
x2 + ε
: RMSE = 23.11 •
y = β
0 + β
1 ∗
x1 + β
2 ∗
x2 + β
3 ∗
x12 + β
4 ∗
x22 + β
5 ∗
x1 ∗
x2 + ε
: RMSE = 22.44 •
ty = β
0 + β
1 ∗
x1 + β
2 ∗
x2 + ε
: RMSE = 0.52 (c) Which has the lowest PRESS? (You get PRESS by pressing the red triangle button at the top, selecting Row Diagnostics and clicking on PRESS) •
y = β
0 + β
1 ∗
x1 + β
2 ∗
x2 + ε
•
y = β
0 + β
1 ∗
x1 + β
2 ∗
x2 + β
3 ∗
x12 + β
4 ∗
x22 + β
5 ∗
x1 ∗
x2 + ε
•
ty = β
0 + β
1 ∗
x1 + β
2 ∗
x2 + ε
Your preview ends here
Eager to read complete document? Join bartleby learn and gain access to the full version
- Access to all documents
- Unlimited textbook solutions
- 24/7 expert homework help
Related Questions
If data set has a relationtionship that is best described by a linear model, then the residual plot will
arrow_forward
Year
2011
2012
2013
2014
2015
Number of federal credit unions
4432
4260
4100
3925
3760
Find a linear model for these data with x=11 correspoding to the year 2011
a) The linear model for these data is y=( )x+( )
b) assuming the trend continues, estimate the number of federal credit unions in the year 2018
arrow_forward
Which of the following is true about the direction of the relationship between education and health? In other words, is it positive, negative, or nonexistent?
Group of answer choices
There is no significant relationship between education and health.
As education goes up, health gets better.
As education goes up, health gets worse
arrow_forward
20) Which of the following is not an assumption about the data when using CVP analysis?
Total fixed costs remain at a constant level and variable costs per unit remain unchanged at all sales levels.
More inventory is purchased than sold.
A linear relationship exists between variable costs and sales activity over the relevant range of interest.
All costs can be easily and accurately separated into fixed and variable components.
arrow_forward
NO chatgpt, solve by hand, and provide all graphs , tables, and visualzaions.
arrow_forward
MAPEH-10-Q3-Week-4.pdfX
Filipino-10-Q3-Week-4.pdf X
TLE CSS 10 Quarter3 Week X
ESP-10-03-Weeks-3-4.pdf
Math 10 Q3 Week 5.p
sers/L%20E%2ON%200%20V%200%20-%20P%20C/Downloads/Math%2010%20Q3%20Week%205.pdf
Facebook
- +
E| D Page view A Read aloud | Draw
E Highlight
b. 362 880
b. 181 440
C. 60 480
d. 30 240
3. Abby is selecting 3 desserts from 10 possible choices displayed on the dessert cart at a restaurant. How
many selections are there?
b. 120
b. 240
C. 360
d. 720
4. A mother, a father, and their 4 children will dine in a restaurant with circular tables. In how many ways
can the family be seated in a table?
b. 24
b. 56
C. 120
d. 720
5. From a Grade 10 class of 28 students, five representatives are to be chosen for academic competition.
In how many ways can students be chosen to represent their class?
b. 12 285
b. 19 565
C. 49 140
d. 98 280
6. Mr. Reyes has a vault with four-digit combination lock. He can set the combination himself. He can use
the digits 0 - 9. Each digit can be used…
arrow_forward
These question need to be solved using R with the given data, please do not provide AI solution , also i need detailed solution , do everything in detail which is required, answer it as soon as possible.
arrow_forward
A pediatrician records the age x (in yr) and average height y (in inches) for girls between the ages of 2 and 10.
Height (in.)
50-
y
Height of Girls vs. Age
(7,48)
40-
(3,38)
30-
20
10-
2
6
8
10
Age(yr)
X
(a) Use the points (3, 38) and (7, 48) to write a linear model for these data.
(b) Interpret the meaning of the slope in the context.
(c) Use the model to forecast the average height of 11-yr-old girls. Round all calculations to the nearest hundredth of an inch, if necessary.
(d) If the height of a girl at age 11 is 90% of her full-grown adult height, use the result of part (c) to estimate the average height of adult women. Round to the
nearest tenth of an inch.
CYDIANATION.
arrow_forward
In order for a linear model between variable and age to be appropriate, the plot must have no pattern right?
arrow_forward
The data in the following table indicate that between the ages of 1 and 11, the human brain does not grow linearly, or steadily. A scatter plot for the data is shown
below the table.
The graphing calculator screen to the right displays the percentage of an adult brain, y, for a child at age x, where 1 sxs11. Use this
information to complete parts (a) through (c) below.
LnReg
y = a+b ln x
a = 29.67892724
b= 29.78535801
E Click the icon to view the table.
W Click the icon to view the scatterplot.
a. Explain why a logarithmic function was used to model the data. Choose the correct answer below.
O A. The data increase rapidly and then continue to increase even more rapidly.
B. The data increase at a steady rate.
OC. The data increase rapidly and then begin to decrease.
D. The data increase rapidly and then begin to level off.
b. Use the graphing calculator screen to express the model in function notation, with numbers rounded to the nearest whole number.
f(x) =
(Type an expression using x…
arrow_forward
A study was conducted to see if a person's income will affect their well-being. We want to create a model with Y = well-being index, and X =
income in $1,000. The dependent variable in this study is:
Income in $1,000
Well-being index
Neither
arrow_forward
An individual's adjusted gross income is the amount of income that is subject to federal income tax. The following table shows the total adjusted gross income (AGI), in trillions of dollars, reported to the IRS in
the given year.
2009
2010 2011
2012
9.1
Year
AGI (trillions)
7.6 8.1
8.6
(a) Show that the data are linear.
, the difference from 2010 to 2011 is
and the difference from 2011 to 2012 is
The difference in the total AGI (in trillions) from 2009 to 2010 is
Because these values are all the same v , the function is linear.
(b) Let t denote the time in years since 2009, and let A denote the total adjusted gross income. Find a linear model for A as a function of t.
A(t) =
(c) Identify the slope of the linear model you found in part (b).
trillion dollars per year
Explain its meaning in practical terms.
O The slope is the initial amount, in trillion of dollars, of the AGI.
O The slope is the number of months it will take the AGI to increase by one trillion dollars.
O The slope is the…
arrow_forward
Explain what is meant when two variables are positively linesrly related. What would scatter plott look like? Two variables x and y are postively linearly related if when the x value increases , the y value increases or decrease? The points on a scatter plot fall approximately in a-an ascending straight line b- a descending curve c- a descending straight line or d- an ascending curve from left to right?
arrow_forward
The table below show data that has been collected from different fields from various farms in a certain valley. The table contains the grams of raspberries tested and the amount of their vitamin c content in mg. Find a linear model that expresses vitamin c content as a function of the weight of the raspberries.
arrow_forward
Several months ago, John O'Hagan investigated the effect on the popularity of OHaganBooks.com of placing banner ads at well-known Internet portals.
When OHagan Books.com actually went ahead and increased Internet advertising from $5,000 per month to $6,000 per month it was noticed that the
number of new visits increased from an estimated 2,030 per day to 2,090 per day. Use this information to construct a linear model giving the average
number v of new visits per day as a function of the monthly advertising expenditure c.
(a) What is the model?
v(c) =
(b) Based on the model, how many new visits per day could be anticipated if OHaganBooks.com budgets $7,000 per month for Internet advertising?
visits
(c) The goal is to eventually increase the number of new visits to 2,600 per day. Based on the model, how much should be spent on Internet
advertising to accomplish this?
$
arrow_forward
I need help with number 14, please.
arrow_forward
Ozone (O3) is a major component of air pollution in many cities. Atmospheric ozone levels
are influenced by many factors, including weather. In one study, the mean percent relative
humidity (x) and the mean ozone levels (y) were measured for 120 days in a western city.
Mean ozone levels were measured in ppb. The following output (from MINITAB) describes
the fit of a linear model to these data. Assume that assumptions 1 through 4 on page 549
hold.
The regression equation is Ozone = 88.8 - 0.752 Humidity
Predictor
Coef SE Coef
тР
Constant
88.761
7.288 12.18 0.000
Humidity -0.7524 0.13024 -5.78 0.000
S= 11.43R-Sq = 22.0°%R-Sq(adj) = 21.4%
%3D
Predicted Values for New Observations
New Obs
FitSE Fit
95.0% CI
95.0% PI
43.62 1.20(41.23 46.00) (20.86, 66.37)
Values of Predictors for New Observations
New Obs Humidity
60.0
What are the slope and intercept of the least-squares line?
Is the linear model useful for predicting ozone levels from relative humidity? Explain.
c. Predict the ozone level…
arrow_forward
Solve only a and b manually using formulas. Show complete solution.
arrow_forward
"Does the relationship between the independent variable and the dependent variable changeas a function of the sector in which an organization operates?" is a core question that we willask in this class. Which of the following variables is best suited to answer this question?a.Correlator variableb.Independent variablec.Moderator variabled.Dependent variable
arrow_forward
First sub-orparto?
arrow_forward
A) Compute the last-squares regression line for predicting US emission from NON US - emissions.
b) If the non-US emission differ by 0.2 from one year to the next by how much would you predict the US- emission to differ?
arrow_forward
Although natural gas is currently inexpensive and nuclear power currently (and perhaps deservedly) does not have a good reputation, it is possible that more nuclear power plants will be constructed in the future. Table 4 presents data concerning the construction costs of light water reactor (LWR) nuclear power plants. The dependent variable, C, construction cost, is expressed in millions of dollars, adjusted to a 1976 base. Preliminary analysis of the data and economic theory indicate that variation in cost increases as cost increases. This suggests transforming cost by taking its natural logarithm.
S Power plant capacity in MWe
N Cumulative number of power plants built by the contractor
Build a multiple regression model to predict ln(C) by taking S and N or their natural logarithms as the independent variables. Make sure to check for multicollinearity. Use residual analysis and R2 to check your model.
State which variables are important in predicting the cost of constructing an…
arrow_forward
Please help me answer this question in detail
arrow_forward
SEE MORE QUESTIONS
Recommended textbooks for you
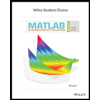
MATLAB: An Introduction with Applications
Statistics
ISBN:9781119256830
Author:Amos Gilat
Publisher:John Wiley & Sons Inc
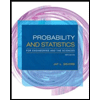
Probability and Statistics for Engineering and th...
Statistics
ISBN:9781305251809
Author:Jay L. Devore
Publisher:Cengage Learning
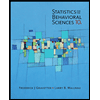
Statistics for The Behavioral Sciences (MindTap C...
Statistics
ISBN:9781305504912
Author:Frederick J Gravetter, Larry B. Wallnau
Publisher:Cengage Learning
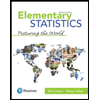
Elementary Statistics: Picturing the World (7th E...
Statistics
ISBN:9780134683416
Author:Ron Larson, Betsy Farber
Publisher:PEARSON
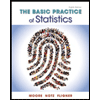
The Basic Practice of Statistics
Statistics
ISBN:9781319042578
Author:David S. Moore, William I. Notz, Michael A. Fligner
Publisher:W. H. Freeman
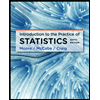
Introduction to the Practice of Statistics
Statistics
ISBN:9781319013387
Author:David S. Moore, George P. McCabe, Bruce A. Craig
Publisher:W. H. Freeman
Related Questions
- If data set has a relationtionship that is best described by a linear model, then the residual plot willarrow_forwardYear 2011 2012 2013 2014 2015 Number of federal credit unions 4432 4260 4100 3925 3760 Find a linear model for these data with x=11 correspoding to the year 2011 a) The linear model for these data is y=( )x+( ) b) assuming the trend continues, estimate the number of federal credit unions in the year 2018arrow_forwardWhich of the following is true about the direction of the relationship between education and health? In other words, is it positive, negative, or nonexistent? Group of answer choices There is no significant relationship between education and health. As education goes up, health gets better. As education goes up, health gets worsearrow_forward
- 20) Which of the following is not an assumption about the data when using CVP analysis? Total fixed costs remain at a constant level and variable costs per unit remain unchanged at all sales levels. More inventory is purchased than sold. A linear relationship exists between variable costs and sales activity over the relevant range of interest. All costs can be easily and accurately separated into fixed and variable components.arrow_forwardNO chatgpt, solve by hand, and provide all graphs , tables, and visualzaions.arrow_forwardMAPEH-10-Q3-Week-4.pdfX Filipino-10-Q3-Week-4.pdf X TLE CSS 10 Quarter3 Week X ESP-10-03-Weeks-3-4.pdf Math 10 Q3 Week 5.p sers/L%20E%2ON%200%20V%200%20-%20P%20C/Downloads/Math%2010%20Q3%20Week%205.pdf Facebook - + E| D Page view A Read aloud | Draw E Highlight b. 362 880 b. 181 440 C. 60 480 d. 30 240 3. Abby is selecting 3 desserts from 10 possible choices displayed on the dessert cart at a restaurant. How many selections are there? b. 120 b. 240 C. 360 d. 720 4. A mother, a father, and their 4 children will dine in a restaurant with circular tables. In how many ways can the family be seated in a table? b. 24 b. 56 C. 120 d. 720 5. From a Grade 10 class of 28 students, five representatives are to be chosen for academic competition. In how many ways can students be chosen to represent their class? b. 12 285 b. 19 565 C. 49 140 d. 98 280 6. Mr. Reyes has a vault with four-digit combination lock. He can set the combination himself. He can use the digits 0 - 9. Each digit can be used…arrow_forward
- These question need to be solved using R with the given data, please do not provide AI solution , also i need detailed solution , do everything in detail which is required, answer it as soon as possible.arrow_forwardA pediatrician records the age x (in yr) and average height y (in inches) for girls between the ages of 2 and 10. Height (in.) 50- y Height of Girls vs. Age (7,48) 40- (3,38) 30- 20 10- 2 6 8 10 Age(yr) X (a) Use the points (3, 38) and (7, 48) to write a linear model for these data. (b) Interpret the meaning of the slope in the context. (c) Use the model to forecast the average height of 11-yr-old girls. Round all calculations to the nearest hundredth of an inch, if necessary. (d) If the height of a girl at age 11 is 90% of her full-grown adult height, use the result of part (c) to estimate the average height of adult women. Round to the nearest tenth of an inch. CYDIANATION.arrow_forwardIn order for a linear model between variable and age to be appropriate, the plot must have no pattern right?arrow_forward
- The data in the following table indicate that between the ages of 1 and 11, the human brain does not grow linearly, or steadily. A scatter plot for the data is shown below the table. The graphing calculator screen to the right displays the percentage of an adult brain, y, for a child at age x, where 1 sxs11. Use this information to complete parts (a) through (c) below. LnReg y = a+b ln x a = 29.67892724 b= 29.78535801 E Click the icon to view the table. W Click the icon to view the scatterplot. a. Explain why a logarithmic function was used to model the data. Choose the correct answer below. O A. The data increase rapidly and then continue to increase even more rapidly. B. The data increase at a steady rate. OC. The data increase rapidly and then begin to decrease. D. The data increase rapidly and then begin to level off. b. Use the graphing calculator screen to express the model in function notation, with numbers rounded to the nearest whole number. f(x) = (Type an expression using x…arrow_forwardA study was conducted to see if a person's income will affect their well-being. We want to create a model with Y = well-being index, and X = income in $1,000. The dependent variable in this study is: Income in $1,000 Well-being index Neitherarrow_forwardAn individual's adjusted gross income is the amount of income that is subject to federal income tax. The following table shows the total adjusted gross income (AGI), in trillions of dollars, reported to the IRS in the given year. 2009 2010 2011 2012 9.1 Year AGI (trillions) 7.6 8.1 8.6 (a) Show that the data are linear. , the difference from 2010 to 2011 is and the difference from 2011 to 2012 is The difference in the total AGI (in trillions) from 2009 to 2010 is Because these values are all the same v , the function is linear. (b) Let t denote the time in years since 2009, and let A denote the total adjusted gross income. Find a linear model for A as a function of t. A(t) = (c) Identify the slope of the linear model you found in part (b). trillion dollars per year Explain its meaning in practical terms. O The slope is the initial amount, in trillion of dollars, of the AGI. O The slope is the number of months it will take the AGI to increase by one trillion dollars. O The slope is the…arrow_forward
arrow_back_ios
SEE MORE QUESTIONS
arrow_forward_ios
Recommended textbooks for you
- MATLAB: An Introduction with ApplicationsStatisticsISBN:9781119256830Author:Amos GilatPublisher:John Wiley & Sons IncProbability and Statistics for Engineering and th...StatisticsISBN:9781305251809Author:Jay L. DevorePublisher:Cengage LearningStatistics for The Behavioral Sciences (MindTap C...StatisticsISBN:9781305504912Author:Frederick J Gravetter, Larry B. WallnauPublisher:Cengage Learning
- Elementary Statistics: Picturing the World (7th E...StatisticsISBN:9780134683416Author:Ron Larson, Betsy FarberPublisher:PEARSONThe Basic Practice of StatisticsStatisticsISBN:9781319042578Author:David S. Moore, William I. Notz, Michael A. FlignerPublisher:W. H. FreemanIntroduction to the Practice of StatisticsStatisticsISBN:9781319013387Author:David S. Moore, George P. McCabe, Bruce A. CraigPublisher:W. H. Freeman
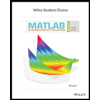
MATLAB: An Introduction with Applications
Statistics
ISBN:9781119256830
Author:Amos Gilat
Publisher:John Wiley & Sons Inc
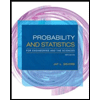
Probability and Statistics for Engineering and th...
Statistics
ISBN:9781305251809
Author:Jay L. Devore
Publisher:Cengage Learning
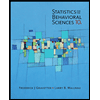
Statistics for The Behavioral Sciences (MindTap C...
Statistics
ISBN:9781305504912
Author:Frederick J Gravetter, Larry B. Wallnau
Publisher:Cengage Learning
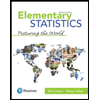
Elementary Statistics: Picturing the World (7th E...
Statistics
ISBN:9780134683416
Author:Ron Larson, Betsy Farber
Publisher:PEARSON
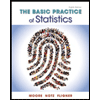
The Basic Practice of Statistics
Statistics
ISBN:9781319042578
Author:David S. Moore, William I. Notz, Michael A. Fligner
Publisher:W. H. Freeman
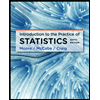
Introduction to the Practice of Statistics
Statistics
ISBN:9781319013387
Author:David S. Moore, George P. McCabe, Bruce A. Craig
Publisher:W. H. Freeman