13.ARTIFICIAL SELECTION EXPERIMENT RESULTS HW-2
docx
keyboard_arrow_up
School
California State University, Chico *
*We aren’t endorsed by this school
Course
330
Subject
Statistics
Date
Feb 20, 2024
Type
docx
Pages
9
Uploaded by ChiefHorse3969
ARTIFICIAL SELECTION EXPERIMENTS HOMEWORK WORKSHEET (Simply fill in the tables and answer questions 1-
10; 30 pts total).
YOU SHOULD HAVE COMPLETED THE FOLLOWING:
Descriptive Statistics (drop-down menu showing Sigma):
o
(means, standard deviation of the samples, coefficients of variation)
Histogram of progeny population
Selection Differential (S), Selection Response (R), using Breeder’s Eqn
Statistical Analysis (data analysis toolpak):
o
(are the two sample populations significantly different?)
FILL OUT THE TABLE BELOW BY INCLUDING THE INFORMATION DETAILED IN QUESTIONS 1-4 (12 pts):
1.
Report the means, standard deviations, and coefficients of variation for the original population, the parent population, and progeny population.
2.
Calculate S, the strength of selection or selection differential, which is the absolute value of the mean progeny/offspring population sample minus the mean of the original population sample: I T
s
– T
o
I
3.
Calculate R, the response to selection, which is the absolute value of the mean of the parent population sample minus the mean of the original population sample: I T
p
– T
o
I
4.
Calculate how heritable the trait we measured was in our samples, h
2
, which is equal to R/S. (Note that you do not square the value for h.)
NOTE: YOUR ANSWERS IN TABLE BELOW WILL BE COUNTED WRONG IF YOU INCLUDE TOO MANY SIGNIFICANT DIGITS! You should only include as many significant digits as your data justify. You don’t want to claim that you had higher accuracy than your data allowed. (That’s like saying you counted an average of 4.5678989468 people, when you are only counting whole people, so your average should be not more than 4.7.)
SAMPLE
SAMPLE MEAN
STANDARD DEVIATION (SD)
COEFFICIENT OF VARIATION
Original Population
Selected Parents
Progeny/Offspring Population
S
R
h
2
5.
What was our response/dependent variable (2 pts)?
6.
Copy and paste below your bar chart with custom error bars for your three sample populations (4 pts).
7.
Was each sample population equally variable? In other words, did their coefficients of variation (CV) remain about the same, or were some more or less variable? Explain the pattern you observed with respect to variability. Feel free to include your histograms to illustrate if you wish (not required, but do report and explain CVs (2 pts).
8.
Did our plants respond to the artificial selection that we introduced? We can use the Breeder’s Equation
to determine the degree to which our progeny/offspring sample changed relative to the original sample:
R = h
2
S (2 pts). If R was greater than zero, there was a response.
9.
Was our trait heritable? (2 pts)
h
2 ranges from 0 to 1 because it is simply the ratio of the response to selection divided by the strength of selection. This ratio essentially gives a measure of how much of the phenotypic character we focused on for change (selection)
is actually under genetic control. This value is almost always less than 1! (We may have had an issue with “novice data collection” the first time we counted hairs in the original population compared to “expert data collectors” when we assessed the offspring population for the same character (hairiness).
10.
Finally, using the data analysis toolpak in Excel, you conducted a t test to determine whether the sample
progeny (offspring) differed significantly from the original population. To report the results of your test, you must include the following information (because it is NEVER sufficient to simply report a p value). Basically, you want to know how big your sample sizes were, how much power you had to detect differences, and whether those differences were statistically significant. Recall also that we decided ahead of time that if there was going to be a change in trichome number, we expected the progeny population to be hairier than the original, therefore are using a one-tailed test (the samples are either the same or the progeny are hairier; we don’t expect them to get less hairy) (6 pts).
NOTE: YOUR ANSWERS IN TABLE BELOW WILL BE COUNTED WRONG IF YOU INCLUDE TOO MANY SIGNIFICANT DIGITS! SAMPLE
SAMPLE SIZE
SAMPLE MEAN +/- SD
TEST RESULTS
Original Population
Selected Parents
Progeny Population
Degrees of Freedom (df)
Calculated t value from data
Critical t value from t table (ONE TAILED)
p Value
11.
Now, to interpret your results, look at the p value that resulted from your output. If that value is > 0.05, your data did not support a significant change in trichome number from the original population to the progeny (offspring) population. If that value is <0.05, then your data support that there was a significant
difference between the original plants and
the progeny that resulted from our selection
experiment. You can think of this as a 95%
chance that your data were significantly
different, assuming normal bell curves:
95% chance that means are different!
Basically, if our comparison of means (the t test) shows an extreme value of t for the degrees of freedom we had, then the sample populations are not the same. I AM INCLUDING THE ENTIRE “STATS BLAST” HERE FOR YOUR CONVENIENCE:
A STATISTICAL BLAST! (Very brief overview of statistics)
(Modified from R. Schleiger.) Statistics is one of the most important tools for scientific inquiry. Because we usually can’t sample everything on Earth, scientists use data collected from a representative sample of individuals to draw inferences about basic biological phenomena for the entire population. Two major misconceptions about statistics:
1.
Fancy, difficult to understand statistics make good science = FALSE
2.
Statistically significant relationships are always biologically important = FALSE General statistical terms:
Data
– Systematically recorded information. Value
– Each measurement or observation
Variable
– The object being controlled, manipulated, measured or observed. There are two main types: Independent and Dependent
Variables (see below).
Population
– Entire set of study objects. Parameter
– Numerical characteristic of population.
Sample
– Sub-collection of objects from population. Statistic
– Numerical characteristic of sample from population.
Descriptive statistics
– Summarized measures of center (mean/average, median, mode) and spread (variance, standard deviation, standard error, etc.) from data recorded.
Inferential statistics
– Hypothesis testing and confidence intervals.
Types of data:
Qualitative (categorical) data
– Data expressed in words, not in terms of numbers:
Ordinal
– (“ordered”) When categories are in a particular order (ex: large, medium, small)
Nominal
– (“named” types) When categories have no natural ordering (ex: dog breed, color)
Your preview ends here
Eager to read complete document? Join bartleby learn and gain access to the full version
- Access to all documents
- Unlimited textbook solutions
- 24/7 expert homework help
How to present
: Pie & bar charts
Quantitative (numerical) data
– Data expressed in terms of numbers.
Continuous
– Whole & fractional numbers (ex: time, height, or weight)
Discrete
–Only whole numbers possible (ex: counts)
How to present
:
Histograms, line-graphs, scatterplots, boxplots, bar charts
DESCRIPTIVE STATISTICS: DESCRIBING THE DATA
Measures of central tendency
– How data cluster around some middle value; 2 most common:
Mean
(average)
– Sum of all values divided by total number of values in the sample/population. This is the most commonly used measure of center under symmetrical data distributions, but is sensitive to outliers (extreme values relative to rest of sample).
Median
– The middle value when the data are ordered sequentially (e.g., lowest to highest). Used when data are skewed (not a bell-shaped curve); the median is resistant to outliers.
Measures of variability (spread)
– Describes how spread out or dispersed the data are.
There are two main measures of spread used in biological inquiry, based on the variance:
Standard deviation
– Quantifies the variation or dispersion from the average of a dataset. A low standard deviation indicates that the data tends to be very close to the mean; a high standard deviation indicates that the data points are spread out over a large range of values. This calculation is sensitive to outliers.
Standard error
– Quantifies the variation in the means from multiple datasets or a sample distribution of your original dataset.
Variance
– Measure of how data points are spread around the mean. Used to calculate standard deviation and error as well.
Data distributions
– Describes the numbers of times each possible outcome occurs in a sample or population. There are two main shapes:
Bimodal
– Two distinct “clumps” of data
Unimodal
(symmetric, right skewed, left skewed) – One distinct “clump” of data
One hump = unimodal (Dromedary Camel)
Two humps = bimodal (Bactrian Camel)
Normal distribution (symmetric, unimodal)
– “bell-shaped”; data are often assumed to be normally distributed (see graph below).
INFERENTIAL STATISTICS: HYPOTHESIS TESTING
Scientific hypothesis:
Tentative explanation about a phenomenon or a narrow set of phenomena observed in the
natural world. This is the backbone of all scientific inquiry! It is important to have a solid biological hypothesis first; it can then be simplified into a statistical hypothesis (as defined below) that becomes the basis for how the data will be collected, analyzed, and interpreted.
Statistical hypotheses:
After defining a strong biological hypothesis, statistical
hypotheses can be created based on what you predict will be the measured outcome(s) in the dependent variable(s). If a study has multiple measured outcomes there can be multiple
statistical hypotheses. Each statistical hypothesis will have two components (Null and Alternative).
Null hypothesis (H
O
)
–
This hypothesis states that there is no relationship (no pattern) between the independent and dependent variables. Example: NO difference in amount of corn harvested from organic vs conventional fertilizer.
Alternative hypothesis (H
1
)
– This hypothesis states that there is a relationship (is a pattern) between the independent and dependent variables. Example: there IS a difference in the quantity of corn harvested from organic vs conventional fertilizer.
For both biological and statistical hypotheses there should be two types of variables defined:
Independent (explanatory) variable
– The phenomenon or phenomena you think will affect the measure you are interested in. Example: type of fertilizer.
Dependent (response) variable
– What you measure in the experiment and what
is affected during the experiment. The dependent variable responds to (depends on) the independent variable. Example: corn harvest yield. Statistical analysis and conclusion:
Finally, after defining the biological hypothesis, statistical hypothesis, and collecting all your data, you can begin statistical analysis. A statistical test will mathematically compare your data patterns against the statistical hypothesis. After computing the statistical test, the outcome will tell you which statistical hypothesis was supported and then how that relates to the biological hypothesis (the focus of your study).
P-value
– Most statistical tests will give a probability that a statistical hypothesis with your observed values could occur just by chance alone, even if the null hypothesis (no relationship between independent and dependent variables) is true. Thus, it is the probability that the null hypothesis is true. (See Table 1 below for how
to use your p-value to make a conclusion.) Note:
P-values are not, however, sufficient to report alone without the context of your experimental design and sample sizes.
Significance value (α, “alpha” level)
– The significance level of a statistical hypothesis test is a fixed probability of wrongly rejecting the null hypothesis (no relationship), if it is in fact true. Most of the time, a 5% (or 0.05) significance level used. This means that 5 times out of 100, you could get the answer that you got by
chance alone; you are 95% confident that the pattern/relationship you found between the independent and dependent variables is real.
Table 1. How to interpret a p-value. *** Although statistics tests statistical hypotheses, your conclusion should ALWAYS be in light of your scientific hypothesis. At an alpha level of 0.05:
If P < 0.05
If P > 0.05
Null hypothesis is rejected, and there is evidence to support the alternative hypothesis.
Null hypothesis is not rejected,
and there is not sufficient evidence to support the alternative hypothesis.
Statistical test options:
The statistical tests described below will be discussed most often in your undergraduate career. For now, we’ll just focus on a putting a few simple tools in your statistical toolbox (there are many more!). Later on (in upper division classes, your job, or graduate school), you will encounter more complicated tools for more specialized biological and statistical problems. Use Table 2 and Table 3 below to guide you to the appropriate statistical test for the data collected, and the important outputs for each test.
T-test (1 or 2 sample)
Your preview ends here
Eager to read complete document? Join bartleby learn and gain access to the full version
- Access to all documents
- Unlimited textbook solutions
- 24/7 expert homework help
Z-test (1 or 2 sample proportion)
Analysis of Variance aka ANOVA (One- or Two-way, depending on 1 or 2 independent variables)
Linear regression
Chi-square (Goodness of Fit, Homogeneity of Variances, or Independence of Variables)
Most commonly used statistical packages (software to do the math) in undergraduate statistics:
*Excel
Analysis Toolpak in Data tab (free add in)
Google sheets
XL Miner Analysis ToolPak in Add-ons tab (free add in; not as good yet)
SPSS or StatCrunch (usually rented by semester for a class you are taking)
R (free download to your computer but has steep learning curve)
Table 2. How do you know which statistical test to use?
Research
question:
Dependent
variable type (#):
Independent
variable type (# (# groups)):
Main
calculation:
Test to use:
Is there a
difference
between
groups?
Categorical (1)
Categorical
(0(-))
X
2
Chi-square
Numerical (1)
Categorical
(1(2))
Mean
Proportions
Z-test
Mean values
T-test
Categorical (1(3+))
Mean values
ANOVA
What is the
degree of
relationship
between
variables?
Numerical (1)
Numerical (1(1))
Line equation
Linear
Regression
Table 3. What is important to know about each test?
Test:
Important Calculations:
Goal of analysis:
Chi-square
Expected values (E), Sample size (# of categories), X2 value
Determine if there is a difference
between Observed and Expected
data
Prop Z-test
Mean Proportion for each group,
Standard deviation for each group, Sample size, Z-value
Determine if there is a significant
difference (>, <, ≠) between the
two groups
T-test
Mean for each group,
Standard deviation for each group, Sample size, T-value
ANOVA
Mean for each group,
Standard deviation for each group, Sample size, F-value
Determine if one group is
significantly different from the
other groups
Linear
Regression
Equation of the line (y-intercept and slope), Determine strength of correlation
(relationship) between variables
Coefficient of determination (R2),
correlation coefficient (r)
Your preview ends here
Eager to read complete document? Join bartleby learn and gain access to the full version
- Access to all documents
- Unlimited textbook solutions
- 24/7 expert homework help
Recommended textbooks for you
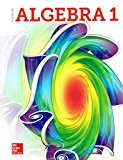
Glencoe Algebra 1, Student Edition, 9780079039897...
Algebra
ISBN:9780079039897
Author:Carter
Publisher:McGraw Hill
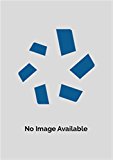
Big Ideas Math A Bridge To Success Algebra 1: Stu...
Algebra
ISBN:9781680331141
Author:HOUGHTON MIFFLIN HARCOURT
Publisher:Houghton Mifflin Harcourt
Recommended textbooks for you
- Glencoe Algebra 1, Student Edition, 9780079039897...AlgebraISBN:9780079039897Author:CarterPublisher:McGraw HillBig Ideas Math A Bridge To Success Algebra 1: Stu...AlgebraISBN:9781680331141Author:HOUGHTON MIFFLIN HARCOURTPublisher:Houghton Mifflin Harcourt
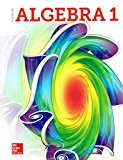
Glencoe Algebra 1, Student Edition, 9780079039897...
Algebra
ISBN:9780079039897
Author:Carter
Publisher:McGraw Hill
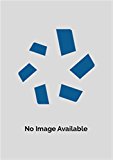
Big Ideas Math A Bridge To Success Algebra 1: Stu...
Algebra
ISBN:9781680331141
Author:HOUGHTON MIFFLIN HARCOURT
Publisher:Houghton Mifflin Harcourt